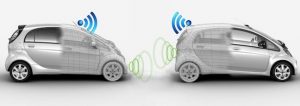
The goal of this collaborative research is to improve driver safety and overall traffic fluidity using innovative intelligent vehicle technology.
This project focuses on the development of novel cooperative perception algorithms for intelligent vehicles operating in realistic traffic scenarios. Scientific challenges are concerned with designing an efficient sensory system, as well as designing perception and sensor fusion algorithms that involve V2X communication. In terms of algorithmic validation, we will work both with real vehicles, provided by PSA Peugeot-Citroën, and simulation tools at various abstraction levels.
This project was featured in the EPFL news.
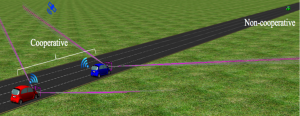
Team and Collaborators
Sponsors and Research Period
This project was sponsored by PSA (Peugeot Citroën Automobiles SA), from September 2012 to August 2017.
Related Student Projects and Internships
DISAL-MP33: Johannes Brakker Løje, Gaussian Process Labeled Multi-Bernoulli Filter for Tracking in Dynamic Environments
DISAL-MP32: Romain Michael Desarzens, Multiple Extended Target Tracking Based on PHD Filter and Gaussian Processes
DISAL-SP85: Jonathan Gan, A Collaborative Fusion and Tracking Algorithm Based on a Sequential Monte Carlo Probability Hypothesis Density Filter
DISAL-SP79: Raphaël Lüthi, Object Classification in Urban Environments by Means of Machine Learning Techniques
DISAL-SP88: David Rivollet, Cooperative Localization Based on Topology Matching
DISAL-SP89: Loïc Veyssière, Comparison of Centralized and Distributed Fusion Architectures in a Sensor Network
DISAL-SP66: Gael Lederrey, Collaborative Sensing and Decision Making for Intelligent Vehicle Maneuvers
DISAL-SP55: Titus Cieslewski, Feature-Based Localization for Autonomous Vehicles
DISAL-SP59: Luca Brusatin, Detection and Classification Using Data from an Automotive Laser Range-Finder
Videos
Embed of video is only possible from Mediaspace, Vimeo or Youtube
Embed of video is only possible from Mediaspace, Vimeo or Youtube
Embed of video is only possible from Mediaspace, Vimeo or Youtube
Publications
Please note that the publication lists from Infoscience integrated into the EPFL website, lab or people pages are frozen following the launch of the new version of platform. The owners of these pages are invited to recreate their publication list from Infoscience. For any assistance, please consult the Infoscience help or contact support.