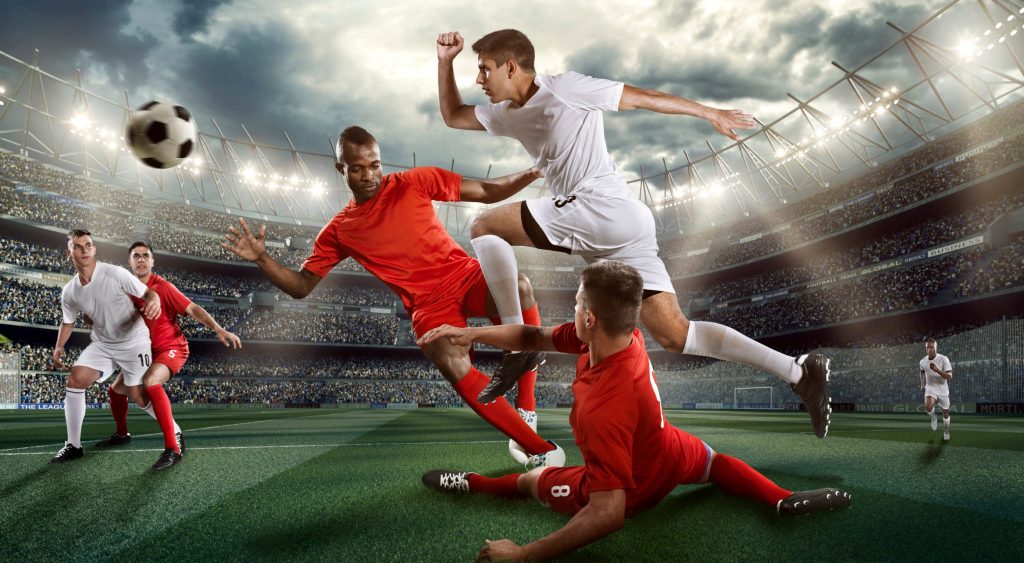
The aim of this project is to raise reliability and accuracy of the human tracking system.
Dartfish aims to help coaches take better advantage of existing data by automating much of its processing. Automated video-based tracking of players, to be developed in this project, is a key component of that. Combined with Dartfish’s data analytics solution, it will power a unique system that combines coaches’ insights with automatically produced data. However, reliability and accuracy are key to ensuring customer acceptance.
The aim of this project therefore is to increase the reliability and accuracy of the system to the point where game tagging can be fully automated in many sports, such as ice-hockey and handball. The core challenge is to keep track of the individual identity of each tracked player. Specifically, researchers will address the identity switch problem in overhead video sequences. To compensate for the limited appearance information, they will introduce a new representation of humans based on semantic keypoints to resolve ambiguities. To this end, they will train a deep network to jointly detect and track semantic keypoints in a unified network. Ultimately, the resulting robust tracking solution will be integrated into Dartfish’s analytics ecosystems.
The Visual Intelligence for Transportation Laboratory (VITA) of Professor Alexandre Alahi has a vast and unique expertise in detecting, tracking, and predicting the motion behaviors of multiple humans. In this project, they will not only advance the performance of each task in the context of sports games but they will combine them in a unified framework to jointly and robustly detect, track humans’ behaviors.
Principal investigator |
Prof. Alexandre Alahi |
Project manager |
Michael Frossard |
Sponsor |
Innosuisse |
Industrial partner |
|
Period |
2021-2023 |
Laboratory |