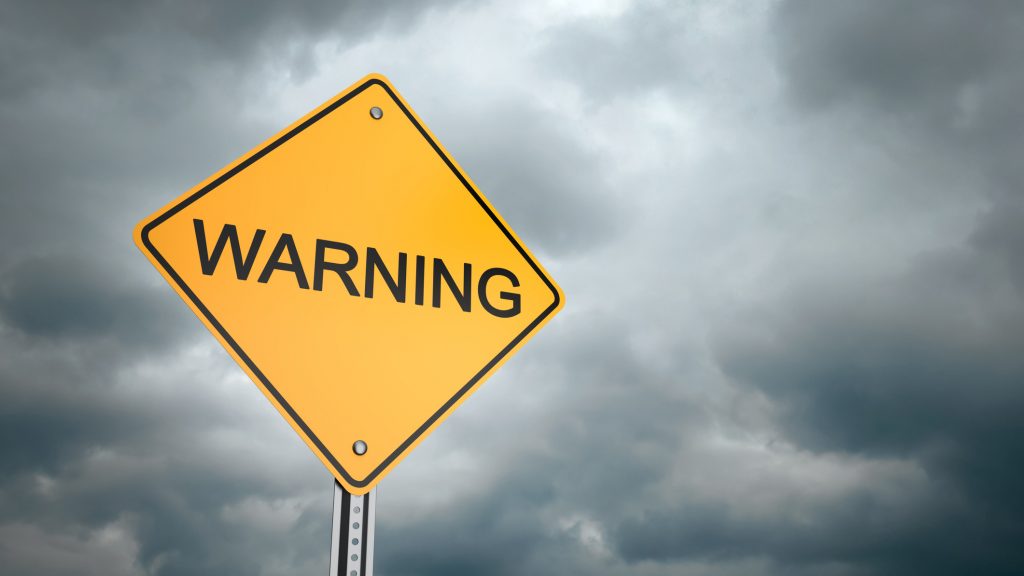
This project aims to develop original predictive methods for data analysis using topology.
Complex dynamical systems can undergo sudden transitions between radically different regimes as their parameters slowly evolve. Phase transitions between solids, liquids, and gases are examples, but this universal phenomenon appears in contexts as varied as financial crashes, climate changes, or traffic congestions. Because of the aberrant nature of these often catastrophic shifts, standard data analysis struggles to detect them early enough for corporations and governments to take appropriate preventive measures.
The goal of this project is to develop new topological methods that can provide early warning signals before unexpected transitions. This will provide powerful machine-learning models to anticipate surprises and make crucial decisions in time. For example, SBB would be able to better detect train failures beforehand.
This research project is led by the Laboratory for topology and neuroscience (LTN) of Professor Kathryn Hess Bellwald in collaboration with L2F, an EPFL start-up that offers mathematically sophisticated machine-learning solutions to corporate and institutional clients, and the HEIG-VD. It lasts 18 months and is sponsored by Innosuisse.
Principal investigator | Prof. Kathryn Hess Bellwald |
Sponsor | Innosuisse |
Period | 2018-2020 |
Partners | L2F, HEIG-VD |
Laboratory | UPHESS |
Collaboration | TRACE |