Visual Intelligence for Transportation VITA
Our work has significant implications for the design of safer and more efficient transportation systems, urban planning, and crowd management strategies. For instance, our work is particularly suited to enable Autonomous Mobility such as self-driving cars or delivery robots coexist with humans in a trustworthy manner.
Beyond embodied agents, we also enable our living spaces – our homes, buildings, and cities – become equipped with ambient intelligence which can sense and respond to human behavior.
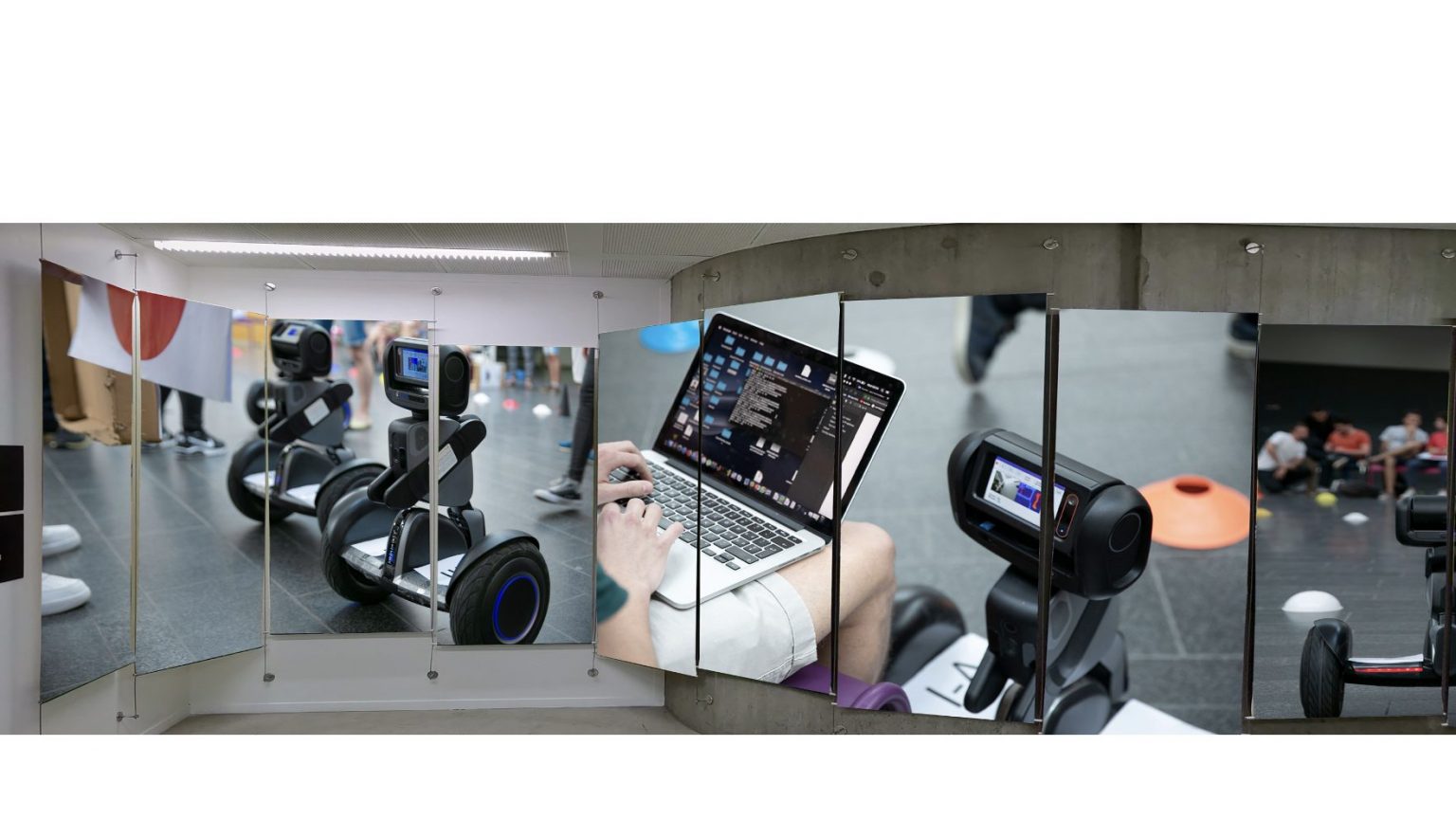

Our Journey at the Intersection of AI and Humanity
Welcome to the VITA lab at EPFL. At the heart of our exploration is a concept we are passionate about: Socially-aware AI for Transportation. This idea represents our quest to inject Artificial Intelligence with Social Intelligence, enhancing not just how we move, but how we coexist with technology in our daily lives.
Our ambition is to develop systems that not only perceive the world with unprecedented clarity but also understand the intricate dance of human behavior in bustling, complex settings. Through our work, we strive to grant machines the gift of social foresight—the ability to anticipate human actions, predict interactions, and, crucially, to act in ways that are both safe and harmonious within human environments.
Our approach is founded on what we call the three ‘P’s of socially-aware AI: Perceive, Predict, and Plan. These pillars guide our research as we develop algorithms capable of navigating the delicate balance between safety and efficiency.
We are especially drawn to the potential for our research to make autonomous mobility—from self-driving cars to delivery robots—not just feasible, but also trusted and seamless parts of our everyday lives. By advancing the field, we aim to contribute to a future where mobility is safe and accessible to all, a vision encapsulated in our goal to “make mobility a safe commodity for all”.
For a glimpse into the heart of our work, here is a brief 3-minute video (or a 12-minute TEDx talk) that summarizes our lab’s pursuits and aspirations.
As we continue on this path, we remain humbled by the complexity of the challenges ahead and inspired by the potential to contribute to a world where technology and humanity advance, hand in hand, towards a more connected and mobile future.
Have fun with our latest real-time demo showcasing some of our lab work on Human/Car/Animal pose estimation, Object detection, Action recognition, 2D to 3D reasoning, Scene Graph generation… and some cool analytics:
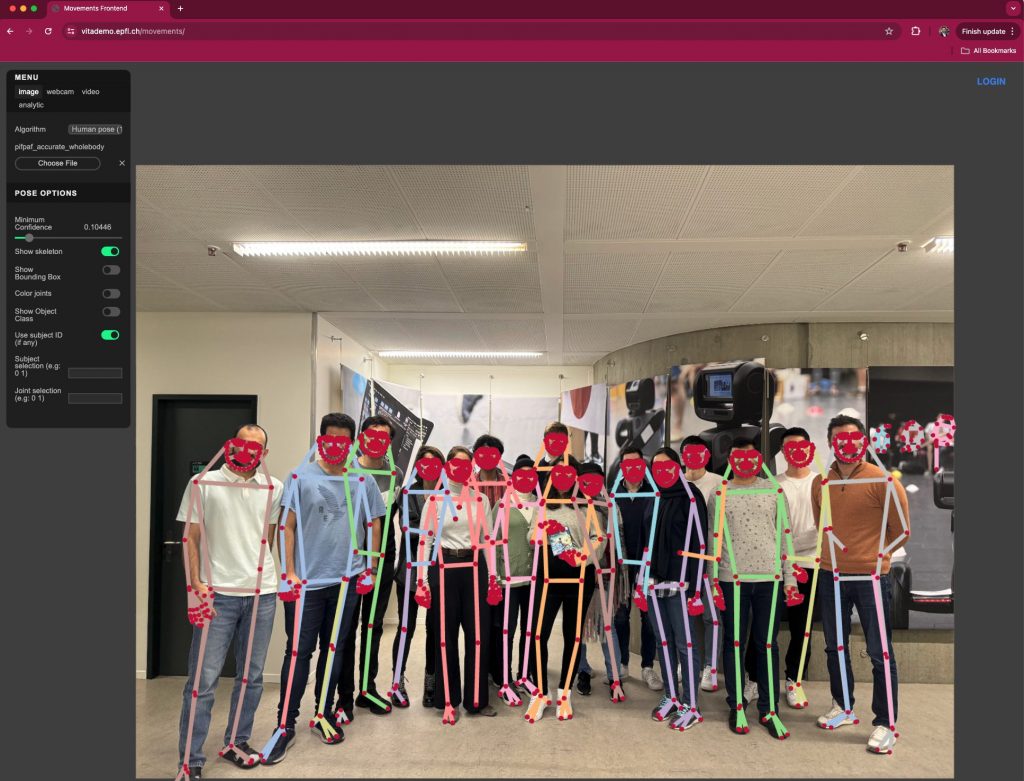