Simple Yet Effective Action Recognition for Autonomous Driving
Weijiang Xiong, Lorenzo Bertoni, Taylor Mordan and Alexandre Alahi
11th Triennial Symposium on Transportation Analysis Conference
(TRISTAN XI) 2022
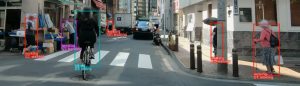
Self-driving cars and delivery robots are set to shape the future of transportation, but they still have to learn how to co-exist with humans in close proximity. Autonomous systems need to detect pedestrians and understand the meaning of their actions before making appropriate decisions in response. Action recognition is therefore an essential task for transportation applications, and yet very challenging, as there is no control over the distances of pedestrians or the real-world variations like lighting, weather, and occlusions.
In this paper, we focus on the action recognition task in the context of transportation applications and deal with real-world variations and challenging scenarios by representing humans through their 2D poses. Human poses are an effective intermediate representation for 2D and 3D human perception tasks. Representing human postures as sparse sets of keypoints allows focusing on essential details while providing invariance to many factors, including background scenes, lighting, textures, and clothes. However, keypoints’ greatest strength is also their main weakness, as such a low-dimensional representation risks neglecting other essential elements in a scene. We propose a simple approach using keypoints as intermediate representations and aim to shed light on which tasks keypoints are effective representations for. We conduct experiments on two datasets related to autonomous driving: TCG and TITAN.