EPFL Data Champions
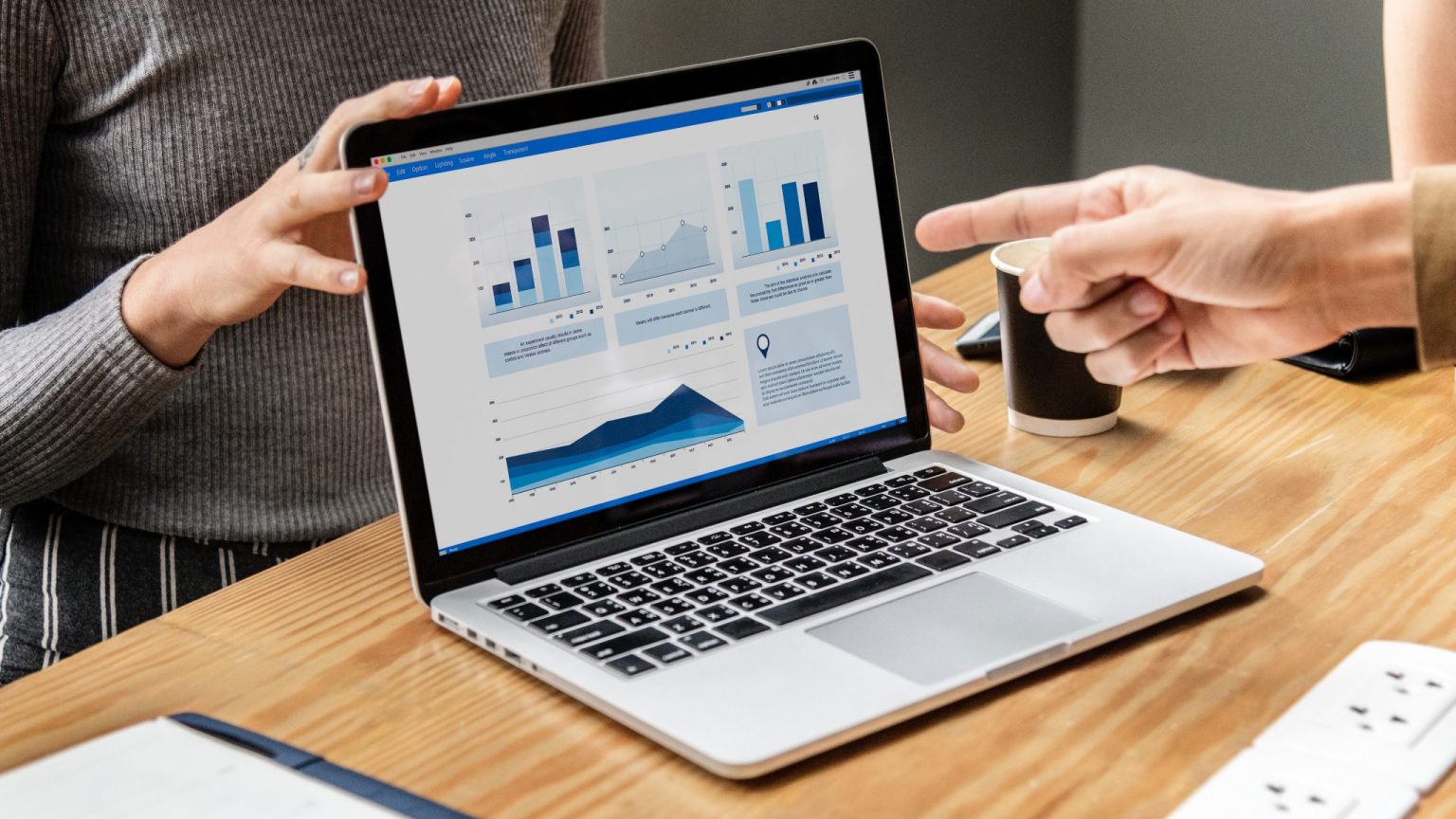
Learn more about the EPFL Data Champions community! Join it or contact [email protected] for further information.
Choose and contact a Data Champion from the list. Not sure who to ask? Then, simply contact [email protected].
Useful info: contacts, Q&A, Drive, and other links, e.g. LinkedIn and GitHub. Share your thoughts or work with the Data Champions, at [email protected].
EPFL Data Champions
Managing data is often a fragmented, frustrating experience on top of research activities … But data generation, analysis, visualization, sharing, etc. greatly affect research projects. Ask an EPFL Data Champion.
Who | What | Where |
Giovanna Ambrosini![]() | Senior Scientist • Bioinformatics • Genomics • Web development | EPFL ORCID GitHub |
Nicolas Argento![]() | Team Leader • ELN/LIMS services manager • IT/Life sciences communication • IT services management | EPFL ORCID |
Manos Barmpounakis![]() | Postdoctoral Researcher • Data in Transportation • Data science • Intelligent Transportation Systems | EPFL ORCID |
Kirell Benzi![]() | Scientist • Data visualization • Data mining • Software engineering | EPFL ORCID GitHub |
Luc Sébastian Bondaz![]() | Doctoral Assistant • Business Analytics • Machine learning • Chemical Process simulation | EPFL |
Olivier Burri![]() | Engineer • Light Microscopy • Image Analysis • Deep Learning | EPFL ORCID GitHub |
Roberto Castello![]() | Data Scientist • Urban data • Machine Learning • Statistics | EPFL ORCID GitHub |
Tom de Geus![]() | Scientist • Mechanics • Comp. Physics • Statistical Physics | EPFL ORCID GitHub |
Simon Dürr![]() | Doctoral Assistant • Molecular Dynamics Simulations • Quantum Chemistry • Genetic Algorithms | EPFL ORCID GitHub |
Maud Ehrmann![]() | Scientist • Natural Language Processing • Large-scale textual data • Digital Humanities | EPFL ORCID GitHub |
Juan Carlos Farah![]() | Research Assistant • Human-Computer Interaction • Software Engineering • Digital Education | EPFL ORCID GitHub |
Brad Fetter![]() | Analyst • Bibliometrics • Data visualisation • Research indicator | EPFL |
Robert Fonod![]() | Research Associate • Machine Learning • Computer Vision • Estimation and Tracking | EPFL ORCID |
Jean-Marie Fuerbringer![]() | Research Associate • Design of experiments • Sensitivity analysis • Matlab | EPFL |
Denis Gillet![]() | Group Leader • Open Educational Data • Learning Analytics • GDPR | EPFL ORCID GitHub |
Hung-Chi Han![]() | Doctoral Assistant • Electronics • Physics • Python | EPFL ORCID |
Johannes Hentschel![]() | Doctoral Assistant • Musicology corpus research • Linked open data • Semantic web | EPFL ORCID GitHub |
Eriks Kletnieks![]() | Doctoral Assistant • Storage • Backup • Visualization | EPFL GitHub |
Graham Knott![]() | Head of Unit • Electron microscopy • Neuroscience • 3D modelling | EPFL ORCID GitHub |
Helena Kovacs![]() | Scientist • Research on teaching • Innovation in education • Qualitative research | EPFL ORCID |
Igor Krawczuk![]() | Doctoral Assistant • Deep learning • Reinforcement learning • pytorch, tensorflow, jax | EPFL ORCID GitHub |
Gleb Kurovskiy![]() | Doctoral Assistant • Macroeconometrics • Causal inference • R | EPFL ORCID |
Bertrand Lacour![]() | Scientist and Lecturer • Product Data Management • Product Lifecycle Management | EPFL |
Rubén Laplaza Solanas![]() | Scientist • Quantum chemistry • Computational chemistry • Computational biology | EPFL ORCID GitHub |
Cyril Matthey-Doret![]() | System specialist • Reproducible research • Genomics • Large-Scale data | EPFL ORCID GitHub |
Noémie Mazaré![]() | Scientific Advisor • Open Science • Science Policy • Open Research Data | EPFL ORCID |
Alexander Nebel![]() | Head of Unit • Data governance • Academic rankings • Institutional data | EPFL |
Hatef Otroshi![]() | Doctoral Assistant • Machine learning • Deep Learning • Computer Vision | EPFL ORCID |
Luc Patiny![]() | Scientist and Lecturer • Information management • Chemistry • ELN | EPFL ORCID GitHub |
Evarist Planet![]() | Bioinformatician • Data science • Statistics • Bioinformatics | EPFL |
Oksana Riba![]() | System Specialist • Ethical data science • FAIR data • Reproducible research | EPFL ORCID GitHub |
Carlos Ronchi![]() | Doctoral Assistant • Bioinformatics • Applied mathematics • Bayesian statistics | EPFL ORCID GitHub |
Charlotte Weil![]() | Data Scientist • Data visualization • Interactive web maps • Spatial data imagery | EPFL ORCID GitHub |
Ivica Zivkovic![]() | Scientist and Lecturer • Physics • Quantum magnetism • FAIR principles | EPFL ORCID |
Community of practice and interest
■ Your colleagues come to you for tips and tricks on how to manage their data? Would you like support and recognition for your help?
■ Are you enthusiastic about data sharing, visualization, anonymization, or publishing? Do they appeal to you beyond your research?
■ Do you wish for a bottom-up cultural shift regarding data management? Are you intrigued by meeting others that feel the same?
If you answered ‘yes’ to any of the above, you should join the EPFL Data Champions community!
Interested? Fill in the form or contact [email protected].
Check out the Community mission statement.
Whether you are an EPFL researcher (PhD student , postdoc, professor, etc.) or staff (admin, technician, etc.) with a keen interest in research data and willing to share your expertise, the EPFL Data Champion community is for you!
Of course, previous data management experience, along with a little programming or communication skills are great advantages… but we believe in diversity!
Whatever your level of expertise in data science, data management, data visualization, etc. we would love to have you on board.
This is a voluntary engagement and we will encourage Data Champions to invest only the time they think they can dedicate to helping others, no more, no less.
EPFL Data Champions will get a chance to play the following roles, according to their personal availability and field experience:
- Advise researchers on data handling or redirect them to expert support on Campus
- Act as spokespersons in your faculty
- Endorse the FAIR (Findable, Accessible, Interoperable, and Re-usable) principles
- Promote (or participate in) training or events
- Develop or promote RDM tools useful for research
- Participate to possible publications on RDM
Don’t miss the opportunity to meet other EPFL Data Champions and interesting guest speakers at the Data Champions Meetings, meetings organized 3 times per year.
Data Champions invest some of their time to help others and this effort must be rewarded. We believe members of the EPFL DC community will enjoy the following personal benefits:
Increase your impact
- Help people!
- Be the spokesperson and local data expert, reporting to EPFL management about the needs of your faculty or research community
- Gain visibility with your personal profile on the Data Champions webpage
Develop your professional network
- Get in touch with others interested in RDM at the EPFL and outside
- Network with researchers in workshops, conferences and events
- Receive news and get to know EPFL services as ReO, TTO, Library, etc.
Learn new skills
- Attend workshops or conferences on data science and data management
- Communicate effectively, enhance presentation skills, lead workshops
- Learn by doing, collaborate to projects for possible development or acquisition of data tools
Boost your CV
- Distinguish yourself with qualifying activities and transferable skills
- Add “EPFL Data Champion” to your personal profile page
- Receive news on career opportunities related to RDM
The Research Data Library Team supports the DCs and
- Creates and maintains this webpage
- Invites the DCs to RDM training opportunities, both introductory and specialized
- Provides basic material and IT resources for the DCs activities
- Support the DCs in responding to the requests they might have
- Organizes the DCs Meetings for the community 3 times per year
- Generates and diffuses reports on DCs’ activities
- Sends to the DCs a monthly newsletter to report on the DCs’ activities (via a short survey), inform on new tools, career opportunities, and invite the DCs to talks, events or publications about RDM
An EPFL Data Champion is not expected to replace other professional roles (e.g. Ethics Committee, IT staff, etc.), nor is held responsible for the consequences of advices to researchers.
The Research Data Library Team supports the functioning of the EPFL Data Champions community. The EPFL Library provides the financial support. Other institutional support also comes from the EPFL Open Science Strategic Committee.
The EPFL is not the first and it’s not alone in this initiative, although it takes some pioneering spirit. We acknowledge the work and stimulating discussions with colleagues and fellow Data Champions at Cambridge University and TU Delft.
The EPFL Library is also a member of SPARC Europe, whose similar initiative more focused on OA, the Open Access Champions, connects people from different countries.
These are just a few examples and, ultimately, the EPFL Data Champions are part of a bigger community and we look positively forward to creating synergies, and sharing ideas and practices around data.
Resources
Contacts
- EPFL Data Champions: [email protected]
- Research Data Library Team: [email protected]
Q&A
Not to be confused with a Data management platform, a Data Management Plan (DMP) is a written document describing how data of a research project is managed during its life-cycle. The DMP covers the processes relative to the collection, analysis, transformation, publication, preservation of data and code of a single project. For multiple projects, you might want to devise a RDM Strategy instead: in case, please contact [email protected].
A DMP is as of now demanded by all major research funders such as SNSF or the EC (H2020, MSCA, etc.). It can also be asked by EPFL ReO in order to assess the correct manipulation of sensitive data, or by the EPFL TTO to document the data management bringing to a patent or industrial collaboration.
In general, a substantial increase in science reproducibility is pursued as a result of this strategic document and its implementation. Its main specific purposes are:
- to be more transparent;
- to comply with research funders;
- to forecast resources and needs;
- to help avoid data loss;
- to improve accountability for data workflow;
- to use data in a future-proof way.
Depending on the complexity, purpose and field of a project, it might take anything between 10 minutes to a few workdays to implement a DMP. This might also depend on the pre-existent familiarity with some Research Data Management (RDM) topics, as well as on the personal or team organization.
For a review of your DMP or for help in implementing it, do not hesitate to contact [email protected].
Also, check out the FastGuides on DMP – Data Management Plan and on Storage, Publication and Preservation.
With its various sites and many schools and affiliated institutes, there are many possible storage options at the EPFL.
The principal one is offered by the VPSI, with file storage services and backup for individual workstations. The VPSI also offers an on-premise “object storage” service (hosted on-site) based on the Amazon S3 protocol and based on a Scality (software) and Cisco (hardware) infrastructure: use the XaaS portal to request for buckets.
The IT within different faculties might also offer more customized storage options, as NAS, for your faculty (as for ex. in STI).
You can have a larger view and find general information on the EPFL offerings at https://support.epfl.ch/epfl.
The national platform for academic use, www.switch.ch, offers cloud storage services such as SWITCHdrive and many others.
Even if deprecated for all sensitive, commercial or any valuable data, the EPFL has also a Google Drive cloud solution (servers are not at EPFL).
For coders, SCITAS offers work storage and the c4science for code repository similar to GitHub (and using the GitHub API for integration with this platform). RENKU is a collaborative data science platform that can be used for managing data, code and code environments. For more educational purposes, you might also want to try NOTO, a JupyterLab platform conceived to test and train with coding in Python, Bash, Octave, C, R, plus other useful features, all in the cloud.
Yes. In particular, you can check the upcoming training opportunities at go.epfl.ch/rdm-training.
The RDM training catalog spans from a crash course to more specialized ones, and with different time lengths to accommodate each one’s agenda.
You can also Book a data librarian or simply contact [email protected] and ask for the next scheduled training opportunities.
The EPFL and SWITCH provide a certain choice of communication tools. For research groups, and depending on the particular needs and sensitivity of discussion and information transmitted, these are the main communication tools for videoconferencing:
- Whereby (GDPR compliant)
- Zoom (EPFL account, security problems)
- Jabber Softphone (EPFL account, cumbersome use)
- SWITCH Online Meetings (& other Jitsi-based, open-source)
Of course, many other communication tools and platforms exist beyond the ones supported by the EPFL. In particular, if one does not need the videoconferencing capability, many groups would benefit from free messaging platforms as
- MatterMost (Open Source, self-hosted alternative to Slack)
- Slack (Popular, commercial solution)
- Meet Infomaniak (Swiss, GDPR compliant solution)
At EPFL there is no central department focusing on data analysis or processing, even though Swiss Data Science Center (SDSC) might be a close match. Moreover, it fundamentally depends on the specific needs and field. Don’t hesitate to contact EPFL Research Data Library Team or the EPFL Data Champions community to explore possible solutions.
Moreover, we suggest getting in touch with the C4Science team, the various ICT centers, the CECAM, etc. for possible solutions, depending on the needs.
You have many options. Of course, the first one would be to contact [email protected], the EPFL service for Research Data Management (RDM). And yes, you can always contact a friendly EPFL Data Champion, they are here to help you out!
If you don’t like the idea of getting in touch directly with someone, you’ll maybe find the information on the RDM webpages go.epfl.ch/rdm. Depending on the specific problem, it might take more or less time to get to a solution, but we got you covered.
At the EPFL there are two main services that can accompany you on the matter of licensing, on which license to adopt as well as how to reuse the data that come with (or without) a certain license. While the Technology Transfer Office (TTO) can help especially if the licensing is linked to contracts or intellectual property issues (as patents), for all other inquiries on data or code licensing you can contact EPFL Research Data Library Team. Don’t hesitate to ask a Data Champion with your interests about possible first-hand experience with licensing issues.
BTW, you might want to take a look at the FastGuide Data & Code Licensing for a short overview and first (fast) guide.
While some platforms for code preservation as Software Heritage offer some clear guidelines on the licensing of code, others as GitHub or Zenodo allow you to choose a license from a vast selection. To know more, check out the FastGuide Data & Code Licensing. In general, while all digital work created at EPFL is owned by the EPFL, the authors might decide for its possible exploitation and use, and the license to attach to it. Of course, this freedom of choice is limited by possible funding agencies (ex. SNSF and EC ask to justify when deciding not to make your work openly available and check for a consistent licensing). Another limit can come by using code derived by a 3rd party, as well as when a contract exists, for instance, between two collaborating research groups or institutes.
While all digital work created at EPFL is owned by the EPFL, the authors might decide on its possible exploitation and use, and the license to attach to it. Thus, the copyright will be retained by the authors and co-authors: in principle, the authorship of code is a difficult matter, because usually pieces of code from different sources and with different existing licenses are integrated. In this case, the single pieces of code will lead to the decision of the correct license. To know more, check out the FastGuide Data & Code Licensing.
Even if in Europe the code cannot be patented, the Technology Transfer Office (TTO) can accompany you in choosing the licensing, especially if linked to contracts or intellectual property issues. For all other inquiries on code or data licensing, please contact EPFL Research Data Library Team or ask a Data Champion.
In general, there exist two types of formats: proprietary and open ones. In order to improve diffusion and reproducibility of your work, consider using open formats from day zero, or converting your data or code from proprietary to open formats. You might check out the FastGuide File Formats, containing a selection of formats and easy guidelines.
Tools like Pandoc and FileConverter can be handy for converting general-use file formats, in many cases there’s the ability to export data to open formats directly from most of the proprietary software, even though sometimes the final files can still be unable to completely map the original files.
As many tools and coding libraries exist that support operations with open formats, working directly with open formats can simplify the collaborations, shorten the time to publication, plus you can skip the conversion from proprietary to open formats.
There exist many data formats and some are specific to the scientific disciplines. If in doubt about using some specific formats or operations, feel free to ask colleagues of another lab if they deal with similar data or code, or contact EPFL Research Data Library Team or ask a Data Champion.
You can always search the internet for code that has already been conceived or tested by others, in web portals such as StackExchange or CodeProject, apart from googling a question.
Last but not least, once you have a code or program to process the format of choice, try to provide means for others to use that code (the code itself, or a link to the correct version): it will also shorten the time to publication, as you will already have clear documentations, plus it boosts the transparency of your work and the possibilities for collaborations or citations, as well as a wider adoption of the format.
Yes. The EPFL Library and the VPSI (now DSI) have working to make one available to the EPFL community, and it’s been inaugurated in 2021!
It is called ACOUA, for Academic Output Archive. In case you want to use it or just some info, simply contact [email protected].
As ACOUA is not made for data publication, even if not optimized for data archiving, you might want to use Infoscience to deposit (small) datasets, or use a full-fledged data repository as Zenodo, with the use of the EPFL Zenodo Community tag, for dataset dissemination.
The choice of a data or code repository depends on different factors, such as collaboration or backup purposes during the research, or for publication or archiving purposes after the research.
Moreover, the decision should account for the specific research field, both for targeting the right public and for possible particular features that the repository might offer.
Generally, one might want to check re3data.org, from subfield specific, to field-specific, to more generic repositories.
Be aware that some research funders such as the SNSF do not reimburse the deposit of data or code on repositories that are for-profit or that do not assign a PID (eg. DOI) such as FigShare or GitHub.
Examples of data repositories:
- Materialscloud (computational materials)
- ioChemBd (chemistry)
- Zenodo (generic)
- FigShare (generic)
- Dryad (bio / medical)
- Dataverse (generic)
- Eudat (generic)
Examples of code repositories:
You might want to take a look at the Open Research Data: SNSF monitoring report 2017-2018 (Page 9) to discover other data repositories currently used by the Swiss researchers.
This might depend on your strategy, on the technical constraints, or on the nature of the dataset itself (genomic sequence data, topographic imagery or molecular dynamics numerical simulations, for instance, can pose different issues).
Some legal issues can be expected in some cases: need for ethics committee approval, or 3rd party data (with other institutions or industrial partners), or licensing problems. In these cases, you might consider using two repositories or two deposits on the same repository:
- a private one, containing data shareable under request
- a public one, containing data shareable with anyone
The size limit policy of a data repository can also be a constraint. For instance, if you want to publish a large dataset (ex. let’s say > 50 GB on Zenodo), you might want to split it up into smaller chunks (ex.: Zenodo has a 50 GB restriction). But if the dataset needs to stay together, then some options exist (ex. you can contact Zenodo’s support).
Moreover, not all datasets allow a splitting that makes sense, as data might be more valuable being together or because they need to accompany the same research paper.
Whenever you deposit data in a data repository, it is assumed that you know who will be the public accessing your dataset.
If depositing data underlying some published results, ie. an article, it would be good practice to deposit data in the format that maximizes the chances for reproducibility of your work.
This might depend on the specific repository, on the field, and on the kind of data. In general, you might want to consider open vs. proprietary formats:
- If you already have worked using files or databases with open format, then you are good to go. The open format boosts interoperability of your work, ie. the possibility to access and modify it within different OSs and many software or coding solutions (eg. CSV instead of PPTX files for tables).
- If you have used files or databases with proprietary format, then you could convert your dataset from a proprietary format to an alternative, open one (eg. PPTX files to CSV ones). This is not always possible, nor it automatically implies that others can reproduce your results with the open files if the used software is proprietary. In this case, try to deposit the dataset in both the proprietary and the converted open formats and – if possible – write in a README some instructions on how to reproduce your work with a non-proprietary software.
To know more about File formats, check out the relative FastGuide.
A first practical aspect is to verify the uniqueness of a dataset, as a Digital Object Identifier (DOI) is a particular case of Persistent IDentifier (PID).
Another reason would be to reduce the probability of link rot: some data or code repositories (as for instance GitHub) do not provide a PID, but a simple URL, which might change depending on many factors. A DOI persistence is instead guaranteed.
A third reason to use a DOI for every publication is to allow for citation metrics, as many citation tools use the DOI to track articles as well as datasets. Last but not least, it is the very first condition for findability of the F.A.I.R. dataset principles that “F1. (Meta)data are assigned a globally unique and persistent identifier”.
“One would think that the desire for high quality would motivate any researcher to implement good data management practices. That is not necessarily the case. Data Management also requires time and effort, which may compete with other research activities such as publishing. So at times there may be a trade-off between data management activities and other research activities. A researcher also needs the skills and tools to implement good data practices.” Research Data Management – A European Perspective (by Filip Kruse, Jesper Boserup Thestrup)
Here follows a list of some of the efforts and benefits to be estimated, both for the community and the single researchers:
Efforts:
- Time subtracted from research activities
- Maintenance of data management tools
- Respect for common rules along the research life-cycle
- Conversion of tools or formats in open alternatives
- Data curation with quality control
- Learn new management and technical skills
- Adapt to new software and tools
Benefits:
- Simplified collaborations
- Enhanced reuse of data for
- the researchers at a later time
- new researchers entering a project
- other researchers
- Reduced time to data curation before publication
- Avoid legal and monetary complaints, especially for collaborations
- Reduce the wasted storage and the impact on finances and environment
- Learn new management and technical skills
- Avoid being at the mercy of private companies for your own workflow
Datasets and procedures already compliant with FAIR principles and funders requests
DC Community mission statement
go.epfl.ch/DC-mission (publicly visible, only DCs can modify)
Gdrive storage
go.epfl.ch/DCdrive (visible by EPFL DCs only)
GitHub organization
go.epfl.ch/DC_github (publicly visible)
Interesting reads
- Creating a Community of Data Champions
- Establishing, Developing, and Sustaining a Community of Data Champions
& - How to build a community of Data Champions: Six Steps to Success
10.5281/zenodo.3383814
Former EPFL Data Champions
As careers in science or elsewhere continue, the Data Champions community recognizes the people who made it thrive, by helping others manage their data and sharing their expertise and passion within the EPFL and beyond.