Projects and labs with Jupyter notebooks
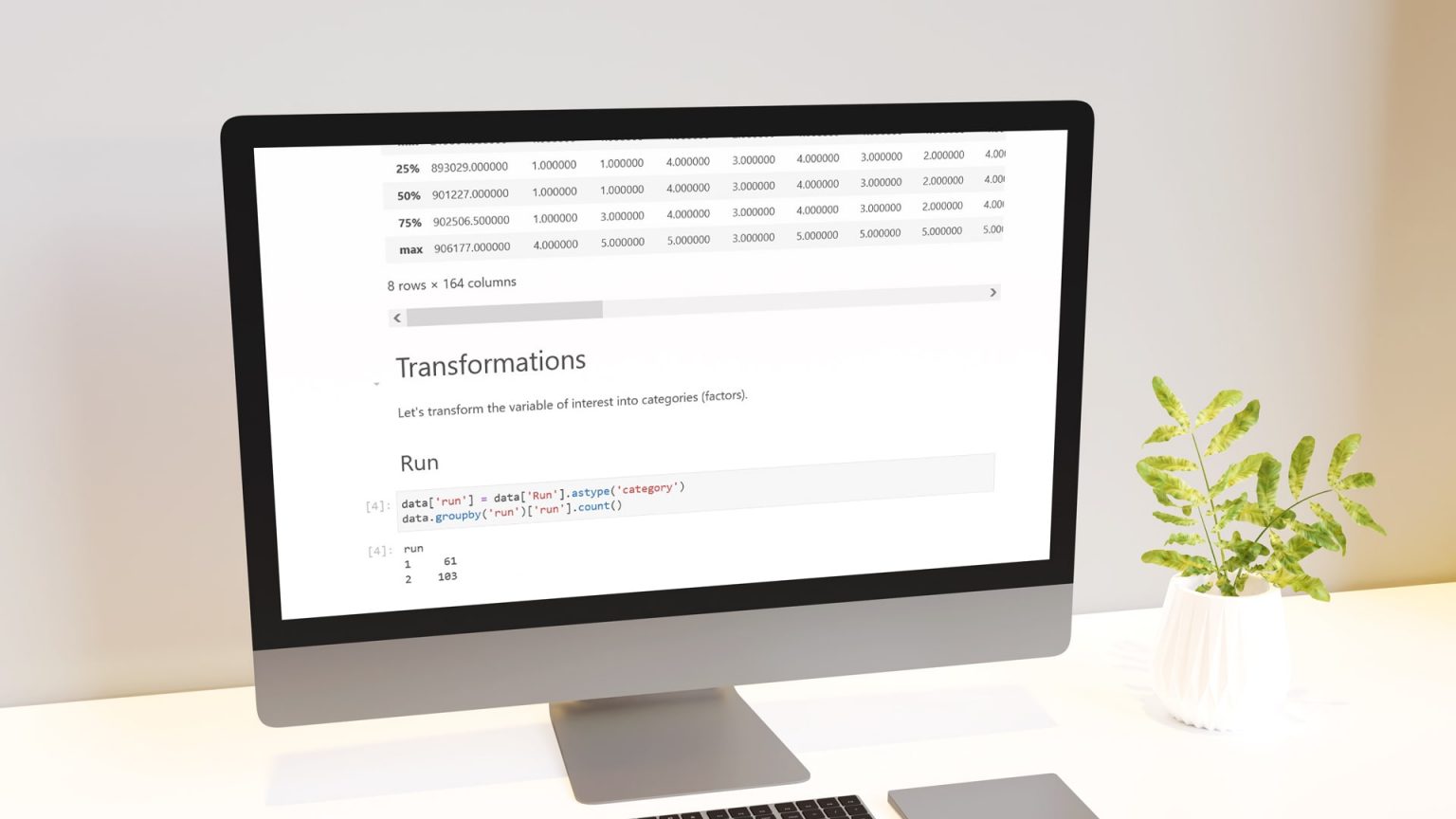
Originally designed by and for researchers as a support for reproducible research, Jupyter Notebooks are a tool of choice for labs and projects involving working with data. But beyond data analysis skills, notebooks can also help students develop scientific investigation skills and computational problem solving.
We detail below how to use notebooks for:
- Scientific data-driven investigations
- Exploratory computational problem solving
- Content writing and collaborative edition
Scientific data-driven investigations with notebooks
Jupyter notebooks are more and more considered an essential tool in many research areas when it comes to exploring data and/or communicating results (Perkel, 2018). As a teacher, you can use notebooks as a bridge between research and education by creating opportunities for students to use notebooks in the way researchers use them, i.e. trying to answer a question by performing a scientific data-driven investigation.
Notebook-based data exploration / analysis activities where students are provided with a real or realistic dataset to analyse make motivating and exciting exercises that can help them develop their data science skills. More generally, such activities are also an opportunity to develop more broadly their scientific investigation skills. We look at how you can support students in acquiring these skills.
#1 Developing students’ data science skills
Experiencing (part of) the research process can be valuable, but for this experience to lead to actual learning, research on education shows that students have to be guided (Furtak et al., 2012; Kirschner et al., 2006). Indeed, one of the challenges for students is to learn from going through a process which is very much exploratory in nature. We share with you two tips to help students to acquire the critical methodological skills involved
A simple and scalable option is to structure your notebooks so that the steps in the investigation process are explicit, in the form of a template for students to complete or to reproduce when they perform their investigation. For instance, a template can make explicit the data cleaning step and provide some guidance as to how to clean data before analysis.
If they blindly follow the steps in the template you provide, students might not realize that the goal is for them to learn about the data analysis process. This is why we advise you to include reflection questions at the end of your notebook to lead students to identify and memorize the methodological steps they have followed during their investigation. Such questions can be as simple as: “list for yourself the different steps involved in analysing a dataset like the one we have used in this notebook”.
#2 Scientific investigation design
Notebook-based data analysis activities are an opportunity for students to learn more broadly about the whole scientific investigation process. Factors such as where the data comes from, how it has been collected, how the experiment has been designed, which hypotheses were made, all influence what can be extracted from the data and how the data can be used to answer questions.
There are different ways to make students aware of these issues in notebook-based data analysis activities, for instance by including information and questions that make the links between the data analysis phase and the wider investigation process. If students can be involved in designing an experiment from scratch and carry it out to answer a scientific question, that is even better!
#3 Conclusion writing and discussion
One particular step in the scientific investigation process that is often underinvested by students – and which happens to involve some of the most important skills we want them to develop – is that of the formulation and discussion of conclusions based on data. Thanks to their narrative component, notebooks support conclusion writing in the same document where data is analysed. Therefore one step you can take for students to develop conclusion drawing skills is to include in your notebook activities that ask students to write conclusions based on the evidence they have analysed. Here are two tips to make such activities effective.
You will probably find out that students often struggle to put their results into words and to assess these results critically. Undeniably, evidence interpretation and critical thinking are skills that are challenging to acquire. In terms of support, including questions into your notebook to guide students through the conclusion writing process can be very helpful for students. Such questions can be for instance: “what piece of evidence allows you to draw your conclusion?”, “what was the hypothesis tested in the experiment and does the evidence support it?”, “what are the potential limits / biases involved in this experiment?”, “what counter-arguments could someone oppose to your interpretation of the evidence?”.
Discussing conclusions requires students to step back from their results and take an external stance, which many will find challenging (just as us researchers do!). An effective approach is to encourage students to discuss their conclusions with peers and/or with teaching assistants, either through small group discussion sessions or through structured peer-review activities. Although a bit more challenging to organize, this latter option has the benefit of formalizing peer review as an authentic and important part of the scientific process. With appropriate guidance and support, e.g. through peer-feedback platforms such as Peergrade, peer review activities can really help students develop essential writing and feedback skills (Price et al., 2016).
Exploratory computational problem solving
So far we have discussed the use of notebooks for activities related to scientific investigation, e.g. practicals and labs involving data analysis. But what can notebooks bring to more general computational problem solving projects? In this section, we review two important ways in which notebooks support thinking and problem solving in projects: the possibility to “tinker” and the possibility to explain.
#1 “Tinkering” in notebooks
In their recent paper on Jupyter, Granger and Pérez share the idea that notebooks support users in a Write – Eval – Think – Loop (WETL): users can write code, evaluate the result with the interactive computing component, think about that result and repeat (Granger & Pérez, 2021). In other words, notebooks are a natural environment for iterative and exploratory problem solving using computation, a type of activity often referred to as “tinkering” (Berland et al., 2013). But why is it (potentially) good to tinker and how to do if effectively?
Tinkering in the sense of goal-oriented experimentation (which therefore differs from trial-and-error) helps us solve problems we have never seen before. It has also been shown to be beneficial for learning, in particular for learning to program (Hancock, 2003). Encouraging students to use notebooks to develop prototypes of their ideas in projects involving computation and/or data can therefore help them both in the development process and in their learning.
Some practices can facilitate structured exploratory problem solving in Jupyter notebooks (in the JupyterLab interface), among which some are regularly used by researchers (Kery et al., 2018; Rule et al., 2019), for instance:
- splitting long cells into smaller ones
- rearranging cells around in a notebook
- collapsing sections and/or cells to hide them
- “deactivating” code cells which are no longer used by transforming them into “raw” cells
- moving cells from one notebook to another
- viewing different portions of the same notebooks side by side using synchronized views
- splitting long notebooks into smaller ones and passing data from one to another using a persistence system (for instance the %store magic, JSON serialization or pickle)
- using the find and replace function
- using the visual debugger
- using version control – see our section below on git
Sharing these tips with students, and considering doing a demo, can prove useful.
#2 Generating computational explanations
Jupyter notebooks have been designed with reproducibility in mind, which means the narrative component is as important as the computation component. As Granger and Pérez put it, “the outcome here is not a software product but ideas and understanding that are “deployed” to other humans.” (Granger & Pérez, 2021). Therefore, inciting students to generate explanations in their notebooks is modelling for them the importance of accompanying software with narrative in notebooks.
When documenting a project or lab work, the process through which the results are obtained is often as (if not more) important as the results themselves. However, students often think the opposite and/or struggle to describe the process in useful terms. Peer review or reproducibility contests can help students identify what is a useful explanatory narrative and what is not.
As Rule et al. identify, there is a tension between exploration and explanation in notebooks (Rule et al., 2018). Documenting a tinkering process is challenging because of the many successive changes made on the notebook (that may even be contradictory). While it remains useful to try to document the work as one goes, producing a notebook that is really suitable for communication to others should probably be a separate task, which happens a posteriori in a separate notebook.
Wondering if your notebook is well designed? Self-assess your notebook using our rubric!
Writing and collaborating in Jupyter notebooks
Using Jupyter notebooks in projects or labs means students will need to write text and/or equations in their notebooks. Depending on their background, your students may be relatively familiar with mainstream word processors with wysiwyg interfaces such as Microsoft Office but have never heard about Markdown or LaTeX. If they work in teams, they also need to figure out how to work collaboratively on notebooks with solutions such as git.
While these tools form a valuable computational toolkit for students’ studies and professional life, learning to use them can be quite challenging. To avoid students spending too much time on formatting issues or solving git conflicts instead of thinking critically about their lab results, it is worth providing them with some support!
Fortunately, Markdown is quite easy to learn, at least for basic formatting. It is worth providing students with a syntax cheatsheet and/or a notebook exemplar with the elements they might need most. A quick introductory tutorial or demo can also help.
Using LaTeX to write equations can prove a bit more difficult. An alternative to documentation and examples is to suggest students to use online visual editors (such as the one by codecogs) that automatically generate the corresponding code. While this solution is not perfect, it can give students a leg up in understanding and using the syntax.
Unfortunately, collaboration is not (yet) a built-in feature of JupyterLab. While collaboration features are progressively being added to JupyterLab, the current tool of choice for collaboration on notebooks is git.
What is git and how does collaboration work on git?
Originally, git is a version control system, which means its role is to track successive versions of files in order to never lose any piece of work. It allows multiple persons to work collaboratively on files through the use of a shared remote repository. Collaboration in git is not real time like in Google Docs, instead each person works on their own local copy of the file and then pushes their modifications to the remote repository. In case of conflicting modifications, the last person to push their modifications will have to go through a conflict resolution process to define which modifications should be kept in the remote repository.
Learning how to use git takes time
Knowing how to use git is a must for anyone who works collaboratively on documents and particularly on code. However, learning how to use git properly takes a bit of time. If you want your students to collaborate using git, it is worth investing in organizing a tutorial or an exercise session on using git and training your teaching assistants for providing students with help. Here are some useful resources on git: the “git for beginners” lesson on Software Carpentry and the Git cheat sheet from Atlassian.
Using git in JupyterLab
Git can be used directly from the JupyterLab interface, either in command line or in point and click interface. For more information, you can see the documentation of the git extension for JupyterLab and our documentation on using git from noto.
Alternatives to git
The platform Google Colab offers a version of Jupyter with collaboration features similar to those of Google Docs, i.e. real time collaboration. A drawback of the platform is data protection issues.
Author: Cécile Hardebolle
Other resources
Discover other ways of using Jupyter Notebooks in your teaching
Bibliography
Berland, M., Martin, T., Benton, T., Smith, C. P., & Davis, D. (2013). Using Learning Analytics to Understand the Learning Pathways of Novice Programmers. Journal of the Learning Sciences, 22(4), 564–599. https://doi.org/10.1080/10508406.2013.836655
Furtak, E. M., Seidel, T., Iverson, H., & Briggs, D. C. (2012). Experimental and Quasi-Experimental Studies of Inquiry-Based Science Teaching: A Meta-Analysis. Review of Educational Research, 82(3), 300–329. https://doi.org/10.3102/0034654312457206
Granger, B. E., & Pérez, F. (2021). Jupyter: Thinking and Storytelling With Code and Data. Computing in Science Engineering, 23(2), 7–14. https://doi.org/10.1109/MCSE.2021.3059263
Hancock, C. M. (2003). Real-time programming and the big ideas of computational literacy [Thesis, Massachusetts Institute of Technology]. https://dspace.mit.edu/handle/1721.1/61549
Kery, M. B., Radensky, M., Arya, M., John, B. E., & Myers, B. A. (2018). The Story in the Notebook: Exploratory Data Science using a Literate Programming Tool. Proceedings of the 2018 CHI Conference on Human Factors in Computing Systems, 1–11. https://doi.org/10.1145/3173574.3173748
Kirschner, P. A., Sweller, J., & Clark, R. E. (2006). Why Minimal Guidance During Instruction Does Not Work: An Analysis of the Failure of Constructivist, Discovery, Problem-Based, Experiential, and Inquiry-Based Teaching. Educational Psychologist, 41(2), 75–86. https://doi.org/10.1207/s15326985ep4102_1
Perkel, J. M. (2018). Why Jupyter is data scientists’ computational notebook of choice. Nature, 563(7729), 145–146. https://doi.org/10.1038/d41586-018-07196-1
Price, E., Goldberg, F., Robinson, S., & McKean, M. (2016). Validity of peer grading using Calibrated Peer Review in a guided-inquiry, conceptual physics course. Physical Review Physics Education Research, 12(2), 020145. https://doi.org/10.1103/PhysRevPhysEducRes.12.020145
Rule, A., Birmingham, A., Zuniga, C., Altintas, I., Huang, S.-C., Knight, R., Moshiri, N., Nguyen, M. H., Rosenthal, S. B., Pérez, F., & Rose, P. W. (2019). Ten simple rules for writing and sharing computational analyses in Jupyter Notebooks. PLOS Computational Biology, 15(7), e1007007. https://doi.org/10.1371/journal.pcbi.1007007
Rule, A., Tabard, A., & Hollan, J. D. (2018). Exploration and Explanation in Computational Notebooks. Proceedings of the 2018 CHI Conference on Human Factors in Computing Systems, 1–12. https://doi.org/10.1145/3173574.3173606