Blue Brain follows a rolling four-year roadmap with specific scientific milestones to achieve during this period as we work towards our ultimate goal of digitally reconstructing the entire mouse brain.
The scientific milestones, which are verified by independent scientists, guide the Blue Brain in our day-to-day science but when achieved and shared, help other brain initiatives and the wider scientific communities achieve their goals.
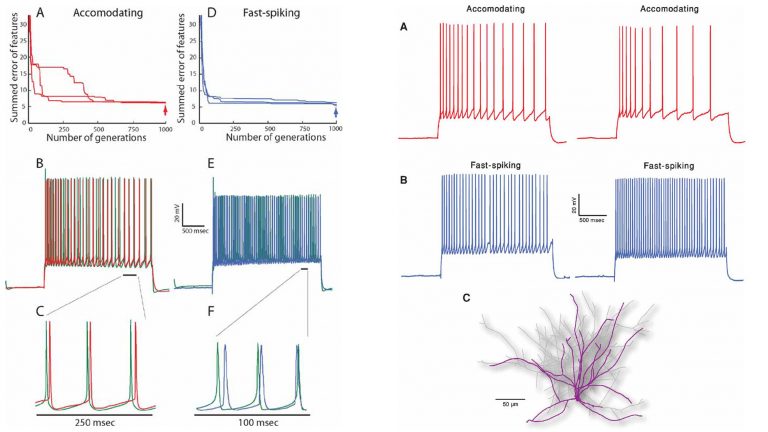
The automatic computer reconstruction of the electrical behavior of any neuron recorded in the brain.
Importance – this approach and respective software has become the standard for many who model neurons because it allows Blue Brain and others to automatically capture in the computer the realistic behavior of millions and even billions of neurons. This was the first essential step to building in the computer a whole brain of approximately a hundred billion neurons.
Achieved in – 2007
Publications
Druckmann, S., Banitt, Y., Gidon, A., Schürmann, F., Markram, H., Segev, I., A Novel Multiple Objective Optimization Framework for Constraining Conductance-Based Neuron Models by Experimental Data. Frontiers in Neuroscience, Vol. 1, Issue 1, 2007.
DOI: 10.3389/neuro.01.1.1.001.2007
Druckmann, S., Berger, TK., Hill, S., Schürmann, F., Markram, H., Segev, I.,
Evaluating automated parameter constraining procedures of neuron models by experimental and surrogate data.
Biol Cybern. 2008 Nov;99(4-5):371-9. Epub 2008 Nov 15.
DOI: 10.1007/s00422-008-0269-2
Druckmann, S., Berger, T., Schürmann, F., Hill, S,, Markram, H., Segev, I.,
Effective Stimuli for Constructing Reliable Neuron Models.
PLoS Comp Biol, 7(8): e1002133.
DOI:10.1371/ journal.pcbi.1002133
Hay, E., Hill, S., Schürmann, F., Markram, H., Segev, I.,
Models of Neocortical Layer 5b Pyramidal Cells Capturing a Wide Range of Dendritic and Perisomatic Active Properties.
PLoS Comp Biol, 7(7): e1002107.
DOI: 10.1371/journal.pcbi.1002107
Hay, E, Schürmann, F., Markram, H., Segev, I.,
Preserving axosomatic spiking features despite diverse dendritic morphology.
J Neurophysiol., 2013 Jun;109(12):2972-81.
DOI: 10.1152/jn.00048.2013. Epub 2013 Mar 27
Van Geit, W., Gevaert, M., Chindemi, G., Rössert, C., Courcol, JD., Muller, EB., Schürmann, F., Segev, I., Markram, H.,
BluePyOpt: Leveraging Open Source Software and Cloud Infrastructure to Optimise Model Parameters in Neuroscience.
Front Neuroinform. 2016 Jun 7;10:17.
DOI: 10.3389/fninf.2016.00017. eCollection 2016.
Masoli, S., Rizza, MF., Sgritta, M., van Geit, W., Schürmann, F., D’Angelo, E.,
Single neuron optimization as a basis for accurate biophysical modelling: the case of cerebellar granule cells.
Front. Cell. Neurosci. 2017; 11:71.
DOI: 10.3389/fncel.2017.00071
Migliore, R., Lupascu, CA., Bologna, LL., Romani, A., Courcol, J-D., Antonel, S., Van Geit, WAH., Thomson, AM., Mercer, A., Lange, S., Falck, J., Roessert, CA., Freund, TF., Kali, S., Muller, EB., Schürmann, F., Markram, H., Migliore, M.,
The physiological variability of channel density in hippocampal CA1 pyramidal cells and interneurons explored using a unified data-driven modeling workflow.
PLOS Computational Biology 14(9).
DOI: 10.1371/journal.pcbi.1006423 2018
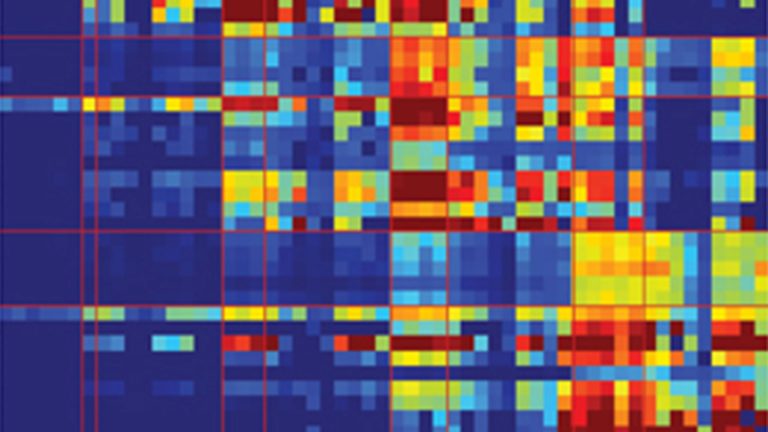
An algorithm that can recreate the connectome of a microcircuit of neurons. This algorithm allowed Blue Brain to capture the way that neurons are connected to each other in the brain and the 3D location of millions of synapses in the modelled microcircuit.
Importance – for the first time, Blue Brain and other scientists had a model to use to study how a neuron processes all the different types of synaptic inputs it receives and can begin understanding how the connectome shapes the function of a microcircuit.
Achieved in – 2015
Publications
Reimann, M.W., Muller, E., Ramaswamy, S., Markram, H: An Algorithm to Predict the Connectome of Neural Microcircuits. 2015. Frontiers in Neural Circuits 9 2015, 28.
DOI: 10.3389/fncom.2015.00120
Kozloski, J., Sfyrakis, K., Hill, S., Schürmann, F., Peck, C., Markram, H., Identifying, tabulating, and analyzing contacts between branched neuron morphologies.
IBM Journal of Research and Development 2008, 52 (1.2), 43–55.
DOI: 10.1147/rd.521.0043
Hill, SL., Wang, Y., Riachi, I., Schürmann, F., Markram, H., Statistical connectivity provides a sufficient foundation for specific functional connectivity in neocortical neural microcircuits. PNAS, 2012 Oct 16;109(42):E2885-94.
DOI: 10.1073/pnas.1202128109. Epub 2012 Sep 18
Magalhaes, B., Tauheed, F., Heinis, T., Ailamaki, A., Schürmann, F., In: Kunkel, J., Balaji, P., Dongarra, J.,
An Efficient Parallel Load-balancing Framework for Orthogonal Decomposition of Geometrical Data.
(eds) High Performance Computing. ISC High Performance 2016. Lecture Notes in Computer Science, vol 9697, Pp. 81-97. Springer, Cham
DOI: 10.1007/978-3-319-41321-1_5
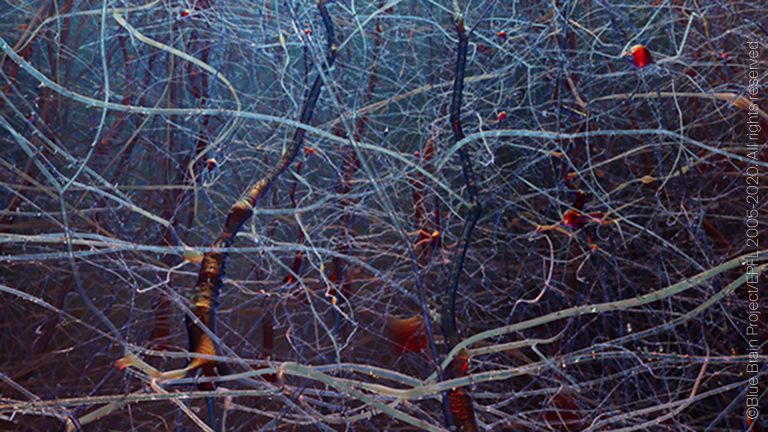
Reconstruction and Simulation of Neocortical Microcircuitry. To bring the different types of neurons and synapses together as a microcircuit, which was demonstrated in the form of the most biologically realistic copy to date of a neocortical column – the “CPU” of the neocortex.
Importance – it demonstrated the extent to which and the accuracy with which we can predict missing data, because it revealed the first glimpse of the cellular and synaptic map of the most complex microcircuit in the mammalian brain. It also provides a proof of concept for building larger circuits such as whole brain regions.
Achieved in – 2015
Publication – H. Markram et al, Reconstruction and Simulation of Neocortical Microcircuitry. Cell 163, 2015, 456-492.
DOI: 10.1016/j.cell.2015.09.029
Model – The Neocortical Microcircuit Collaboration Portal – https://bbp.epfl.ch/nmc-portal/welcome
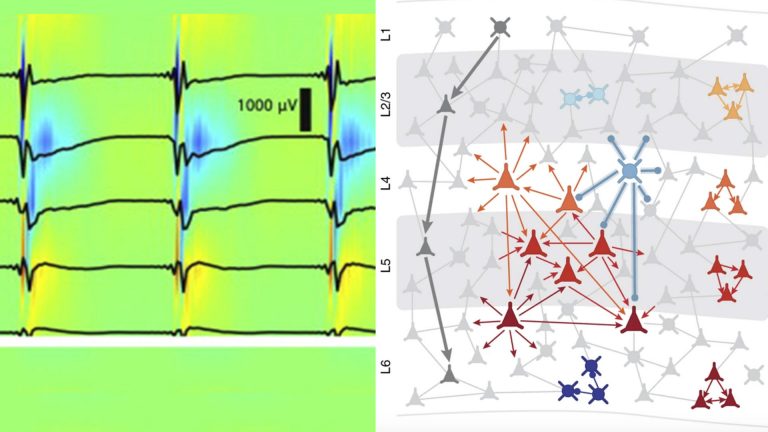
The validation and exploration of the emergent dynamics of the microcircuit in milestone three. The model gave rise to a whole range of states, solely by integrating measurements. It also provides new insights into the connectivity structure of cortical circuits and provided the key topological features of the neocortical microcircuit.
Importance – this was a key milestone because it showed, for the first time, that we could integrate all available data about a cortical microcircuit into a digital reconstruction that would give rise to a complex array of network states comparable to that observed in real circuitry. We could use this model to simulate the transition between states by changing ionic concentrations or conductances. As we can already compute the electrical field generated by all the elements in this circuit, this milestone paves the way for bridging between subcellular, cellular and synaptic activity and the electroencephalography (EEG) waveforms measured in the lab and clinic.
Achieved in – 2015
Publications
Markram et al, Reconstruction and Simulation of Neocortical Microcircuitry. Cell 163, 2015, 456-492.
DOI: 10.1016/j.cell.2015.09.029.
Reimann, M., Anastassiou, C., Perin, R., Hill, S., Markram, H., Koch, C., (2013) A biophysically detailed model of neocortical local field potentials predicts the critical role of active membrane currents. Neuron, 79(2), 375-390, 2013.
DOI: 0.1016/j.neuron.2013.05.023
Gal, E., London, M., Globerson, A., Ramaswamy, S., Reimann, M.W., Muller, E., Markram, H., and Segev, I. (2017). Rich cell-type-specific network topology in neocortical microcircuitry. Nat. Neurosci. 20, 1004–1013.
DOI: 10.1038/nn.4576
Reimann, M., Horlemann, A., Ramaswamy, S., Muller, E., Markram, H. (2017). Morphological Diversity Strongly Constrains Synaptic Connectivity and Plasticity. Cereb.Cortex 27, 4570–4585.
DOI: 10.1093/cercor/bhx150
Reimann, M., Nolte, M., Scolamiero, M., Turner, K., Perin, R., Chindemi, G., Dłotko, P., Levi, R., Hess, K., Markram, H. (2017). Cliques of Neurons Bound into Cavities Provide a Missing Link between Structure and Function. Front Comput Neurosci 11, 48.
DOI: 10.3389/fncom.2017.00048
Ramaswamy, S., Colangelo, C., Markram, H. (2018). Data-Driven Modeling of Cholinergic Modulation of Neural Microcircuits: Bridging Neurons, Synapses and Network Activity. Front. Neural Circuits 12, 77–77.
DOI: 10.3389/fncir.2018.00077
Nolte, M., Reimann, M., King, J., Markram, H., Muller, E., Cortical reliability amid noise and chaos. Nature Communications, 22 August 2019,
DOI: 10.1038/s41467-019-11633-8
Chindemi, G., Abdellah, M., Amsalem, O., Benavides-Piccione, R., Delattre, V., Doron, M., Ecker, A., King, J., Kumbhar, P., Monney, C., Perin, R., Rössert, C., Van Geit, W., DeFelipe, J., Graupner, M., Segev, I., Markram, H., Muller, E. (2020). A calcium-based plasticity model predicts long-term potentiation and depression in the neocortex. bioRxiv, 2020.04.19.
DOI: 10.1101/2020.04.19.043117
Nolte, M., Gal, E., Markram, H., and Reimann, M.W. (2020). Impact of higher-order network structure on emergent cortical activity. Network Neuroscience. 4, 1–36.
DOI: 10.1162/netn_a_00124.
Amsalem, O., King, J., Reimann, M., Ramaswamy, S., Muller, E., Markram, H., Dense Computer Replica of Cortical Microcircuits Unravels Cellular Underpinnings of Auditory Surprise Response (June 2020)
DOI: 10.1101/2020.05.31.126466
Newton, T., Abdellah, M., Chevtchenko, G., Muller, E., Markram, H.,
Voltage-sensitive dye imaging reveals inhibitory modulation of ongoing cortical activity (2019)
DOI: 10.1101/812008
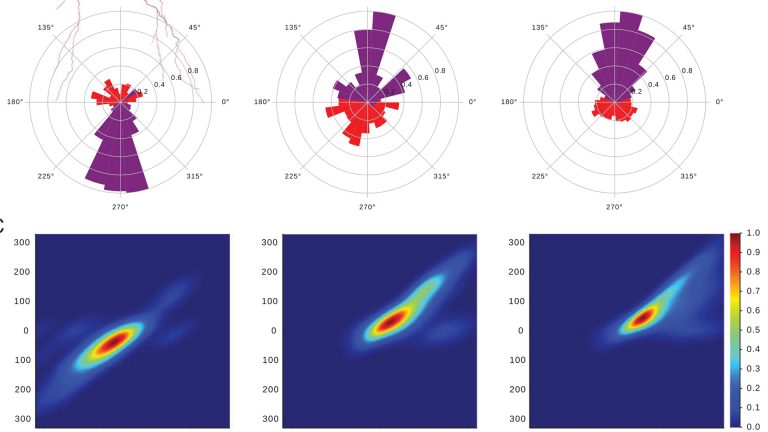
Blue Brain solved a decade old problem of mathematically growing the shape of neurons (their morphology).
Importance – for Blue Brain, we needed to reach this milestone because normally one can only use neurons that are recorded in the brain in the laboratory and 3D digitally drawn under a microscope; this can never yield enough neurons to build whole brain model. This milestone is also important because it provides us with a new way, in the future, to build neurons by reading their genetic information. For example, in the future, we may be able to study the effect of genetic changes in the brain in terms of how they affect the shapes of neurons.
Achieved in – 2019
Publications
Kanari, L., Ramaswamy, S., Shi, Y., Morand, S., Meystre, Julie., Perin, R., Abdellah, M., Wang, Y., Hess, K., Markram., Objective Morphological Classification of Neocortical Pyramidal Cells. Cerebral Cortex, Volume 29, Issue 4, April 2019
DOI: 10.1093/cercor/bhy339
Kanari, L., Dłotko, P., Scolamiero, M., Levi, R., Shillcock, J., Hess, K., Markram, H. (2018a). A Topological Representation of Branching Neuronal Morphologies. Neuroinformatics 16, 3–13.
DOI: 10.1007/s12021-017-9341-1
Vanherpe, L., Kanari, L., Atenekeng, G., Palacios, J., and Shillcock, J. (2016). Framework for efficient synthesis of spatially embedded morphologies. Phys Rev E 94, 023315.
DOI: 10.1103/PhysRevE.94.023315
Software release – the software used for the extraction of the persistence barcodes from neuronal reconstructions is available in the public domain https://github.com/BlueBrain/TMD under the GNU Lesser General Public License, version 3 (LGPLv3).
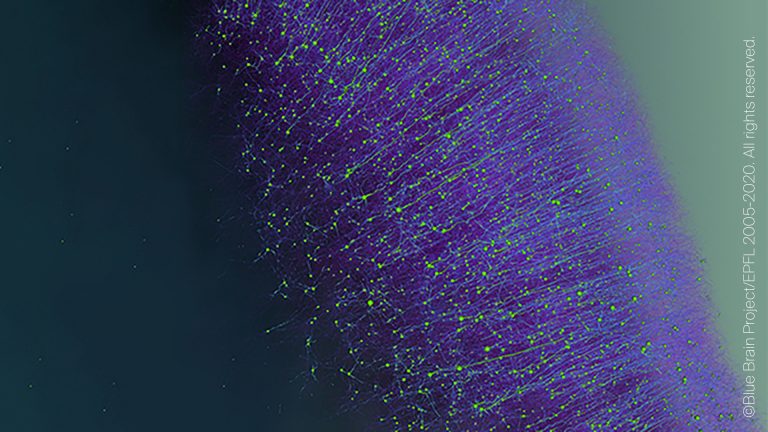
Blue Brain validated that the methods developed to build microcircuits can be generalized to building a whole brain region with curved shape and differences in cellular composition and synaptic properties. Here we targeted the somatosensory cortex for which a paper is in preparation.
Importance – this demonstrates that the principles Blue Brain had developed to reconstruct the microcircuitry can be extended to a much larger region – around two million neurons.
Achieved in – 2020
Publication – pending 2022
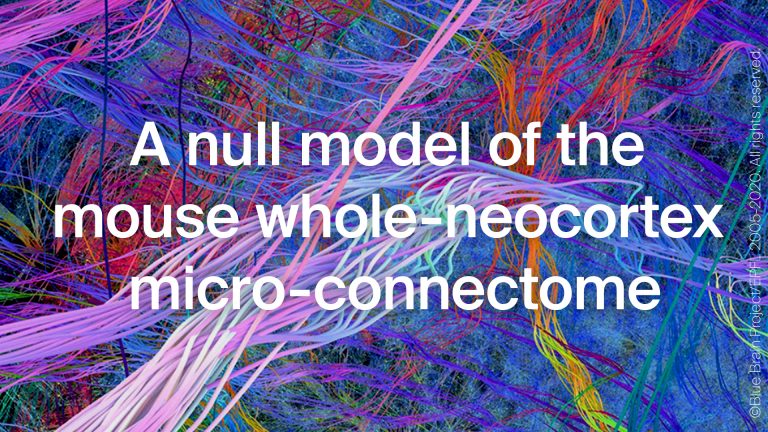
An algorithm to connect the ~11 million neurons in the mouse neocortex. This was a very challenging milestone because the data was very sparse. There existed some tracing data on which parts of the neocortex connect to which other parts, that we used to find a set of rules, which allowed us to predict the formation and location of 88 billion synapses that connect these neurons.
Importance – Blue Brain can now build all the brain regions making up the neocortex. It also demonstrates how completely surprising and novel solutions can be found using very sparse experimental data and it reveals a number of interesting principles of how every neuron is connected in the neocortex. Passing the milestone allows us now to build the first digital reconstruction of the entire neocortex.
Achieved in – 2019
Publication – Reimann, M.W., Gevaert, M., Shi, Y., Lu, H., Markram, H., and Muller, E. A null model of the mouse whole-neocortex micro-connectome. Nature Communications 29 August 2019.
DOI: 10.1038/s41467-019-11630-x
Model released – Mouse whole-neocortex connectome model via the Blue Brain Portal
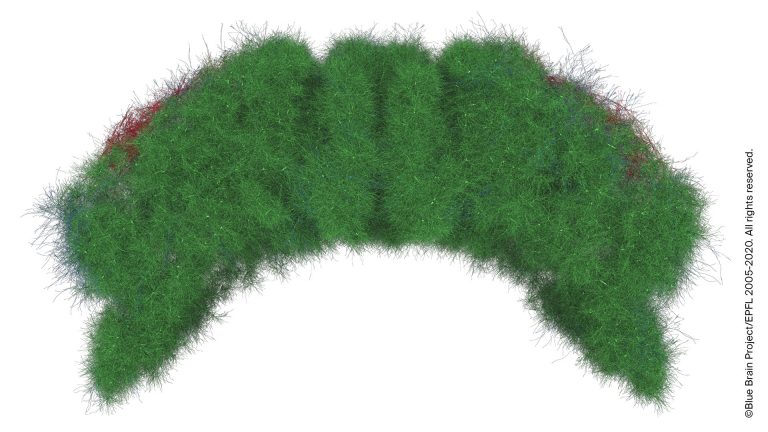
The eighth milestone was to extend our algorithmic reconstruction approach to structures with direct relevance for the neocortex. Specifically, we started with the thalamus due to its importance for funnelling sensory input to the neocortex.
Importance – this milestone was important because it allows us to refine in silico experiments of cortical models due to the improved details on the sensory input pathways and it furthermore allowed us to see how our algorithmic approaches generalize to other brain regions.
Achieved in – 2019
Publications on circuit
O’Reilly, C., Iavarone, E., Yi, J., & Hill, S. L. (2021). Rodent somatosensory thalamocortical circuitry: Neurons, synapses, and connectivity. Neuroscience & Biobehavioral Reviews, 126, 213–235. https://doi.org/10.1016/j.neubiorev.2021.03.015
Simko, J., & Markram, H. (2021). Morphology, physiology and synaptic connectivity of local interneurons in the mouse somatosensory thalamus. The Journal of Physiology, 599(22), 5085–5101. https://doi.org/10.1113/JP281711
Publication on neurons
Iavarone E., Yi J., Shi Y., Zandt B.J., O’Reilly C., Van Geit W., Rössert C., Markram, H., Hill, S.L. (2019) Experimentally constrained biophysical models of tonic and burst firing modes in thalamocortical neurons. PLOS Computational Biology 15(5): 1-23. e1006753. https://doi.org/10.1371/journal.pcbi.1006753
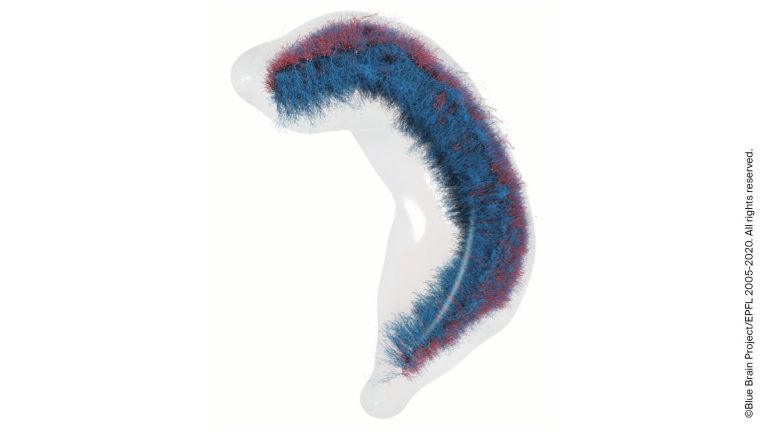
The ninth milestone was to validate more generally that our algorithmic reconstruction approach works outside of the neocortex, which we tested by reconstructing the hippocampus in collaboration with external groups.
Importance – this milestone is important because it means that the processes and algorithms that we had developed for the neocortex also work, with some adaptation, for other brain regions. The specific adaptations taught us about different principles operating in their different brain regions.
Achieved in – 2019
Publication on circuit
Ecker, A., Romani, A., Sáray, S., Káli, S., Migliore, M., Falck, J., Lange, S., Mercer, A., Thomson, A. M., Muller, E., Reimann, M. W., & Ramaswamy, S. (2020). Data‐driven integration of hippocampal CA1 synaptic physiology in silico. Hippocampus, 30(11), 1129–1145. https://doi.org/10.1002/hipo.23220
Publication on neurons
Migliore, R., Lupascu, C.A., Bologna, L.L., Romani, A., Courcol, J.-D., Antonel, S., Van Geit, W.A.H., Thomson, A.M., Mercer, A., Lange, S., Falck, J., Roessert, C. A., Freund, T. F., Kali, S., Muller, E. B., Schürmann, F., Markram, H., Migliore, M. (2018). The physiological variability of channel density in hippocampal CA1 pyramidal cells and interneurons explored using a unified data-driven modeling workflow. PLOS Computational Biology 14(9).
DOI: 10.1371/journal.pcbi.1006423
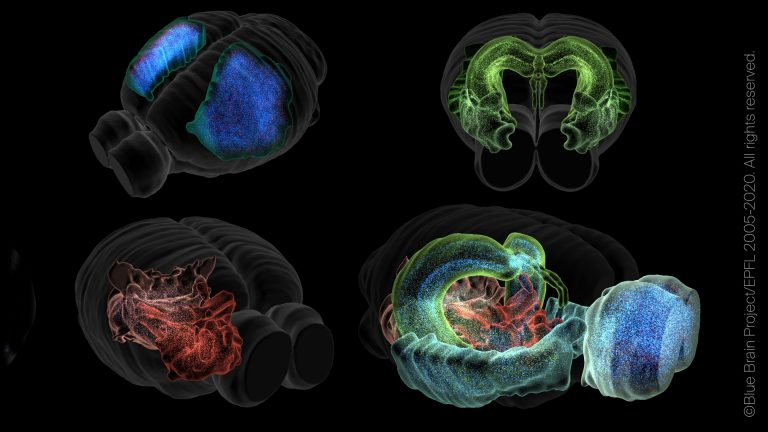
Our tenth milestone was to build a full cell atlas of every neuron and glial cell in the whole mouse brain. We published and released this atlas for the community to use in 2018.
Importance – This milestone is an important building block which will enable us to grow all the neuronal morphologies, capture all the different electrical behaviors of each type of neuron, and connect all the neurons to build the first draft of the whole mouse brain by 2024.
Achieved in – 2018
Publication – Erö, C., Gewaltig, M.-O., Keller, D., and Markram, H. (2018). A Cell Atlas for the Mouse Brain. Frontiers in Neuroinformatics 12, 84.
Cell Atlas – https://portal.bluebrain.epfl.ch/resources/models/cell-atlas/
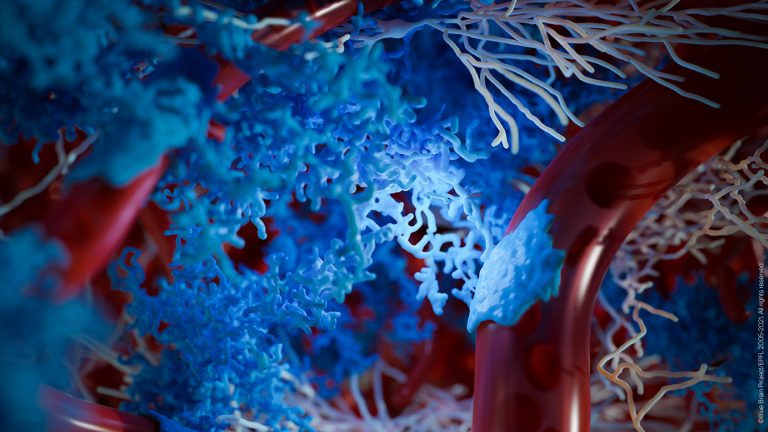
The first digital reconstruction of the brain’s power source – the Neuro-Glia-Vascular Architecture. The complex structural organization of the two dominant cell classes in the brain, neurons and glia, which play a role in these effects, is known as the Neuro-Glia-Vascular (NGV) system. Now, for the first time, Blue Brain scientists have digitally reconstructed the architecture of this system including neurons, glia and blood vessels, providing a new framework to study brain function in health and disease.
Importance – Now we can reconstruct the architecture of non-neuronal entities such as blood vessels and the supporting cells called glia. This means it is possible to capture the way that neurons, glia and the blood supply interact. These reconstructions of the brain tissue provide a sub-micron precise framework needed to simulate the molecular interactions relevant to understanding how neurons are supported and nurtured. They can also be used to investigate how drugs interact and explore how neurodegenerative diseases arise.
Achieved in – 2021
Publication – Zisis, E., Keller, D., Kanari, L., Arnaudon, A., Gevaert, M., Delemontex, T., Coste, B., Foni, A., Abdellah, M., Calì, C., Hess, K., Magistretti, P. J., Schürmann, F., & Markram, H. (2021). Digital reconstruction of the neuro-glia-vascular architecture. Cerebral Cortex. Volume 31, Issue 12, December 2021, Pages 5686–5703, https://doi.org/10.1093/cercor/bhab254
Model released – Neuro-Glia-Vasculature Portal – https://bbp.epfl.ch/ngv-portal/
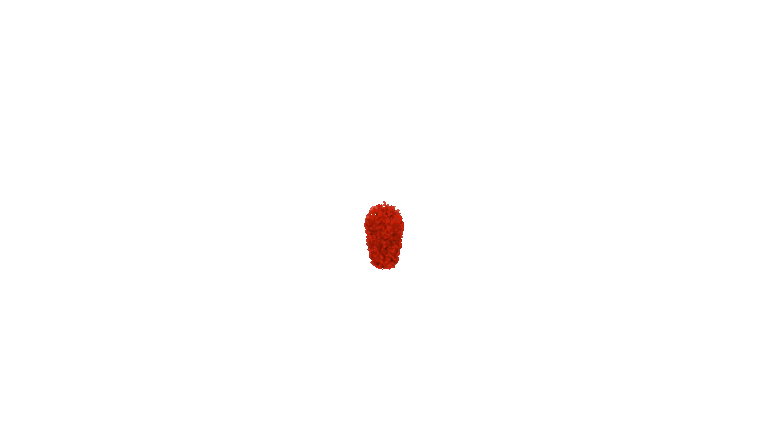
The mouse brain point neuron framework, a dynamic model with accurate cellular composition. Starting with the Blue Brain Cell Atlas, a complete framework that accurately represents cell densities, and the gene expression of the ca. 70M neurons was built. It already includes placeholders for individual neuron shapes, electrical properties, synapse, and connectivity.
Importance – The model will act as a framework in which the synthesized neurons can be accurately placed and their connections and activity simulated. While the Cell Atlas achieved in Milestone Ten described brain anatomy from a static point of view, the point neuron model is a functional framework. It will allow the dynamic connections between various components (neurons, brain areas, etc) to be simulated and studied.
Achieved in – 2022
Publications
Rodarie D, Verasztó C, Roussel Y, Reimann M, Keller D, Ramaswamy S, et al. (2022) A method to estimate the cellular composition of the mouse brain from heterogeneous datasets. PLoS Comput Biol 18(12): e1010739. https://doi.org/10.1371/journal.pcbi.1010739
Roussel Y, Verasztó C, Rodarie D, Damart T, Reimann M, Ramaswamy S, et al. (2023) Mapping of morpho-electric features to molecular identity of cortical inhibitory neurons. PLoS Comput Biol 19(1): e1010058. https://doi.org/10.1371/journal.pcbi.1010058
So far, Blue Brain has established a solid approach to feasibly reconstruct, simulate, visualize and analyze a digital copy of mouse brain tissue and the whole mouse brain. We are now at the stage where we can accelerate the building of larger mouse brain regions and integrate more biological detail. Furthermore, we can use the modeled circuit for gaining new insights into the operation of large-scale circuits, connecting the micro-scale cellular level (e.g., membrane ion channels, synaptic and spike dynamics) to the macro-scale level (the emergence of behavioral-related brain states) – e.g., the work by Reimann et al. (2013), Newton et al. (2021) and Chindemi et al (2022) or the works released as preprints by Amsalem et al. and Romani et al.