A joint project of EPFL Solar Energy & Building Physics Lab and UNIL Institute of Earth Surface Dynamics
Team @EPFL: Prof. Jean-Louis Scartezzini, Dr Roberto Castello, Alina Walch
Team @UNIL: Prof. Mikhail Kanevski, Fabian Guignard
External project supervisor: Dr Nahid Mohajeri (senior research fellow at University College London)
Funding: Swiss National Science Foundation – Nation Research Program 75 “Big Data”
Project duration: 2017-2020
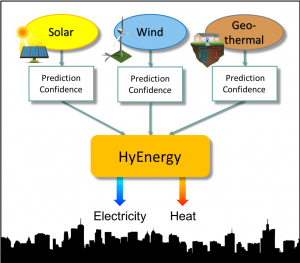
Project explained by Dr Roberto Castello
Objectives
The main objective of this project is to develop a methodology for forecasting the spatio-temporal potential of a combination of renewable energy resources for built areas. Using the available environmental and urban data together with the most advanced data learning techniques, the project aims at:
- Estimating the hybrid renewable energy potential in the built environment, in order to mitigate the effects of variability in individual energy resources and improve the reliability of power generation
- Developing machine learning algorithms for spatio-temporal environmental data processing and analysis as well as for urban and buildings dataset classification
- Applying the developed algorithms to the built environment for predicting energy generation and potential energy savings of hybrid renewable resources
- Providing forecasting models according to the projected climate scenarios for 2035 and 2050
- Estimating uncertainty and validate models using measurement data from weather stations and energy providers
- Proposing a Building Renewable Energy Database (BRED), geo-visualisation tools and renewable energy mapping to support evidence-based decision-making processes.
Impact
Using Big Data and the most advanced learning technologies, our project will make possible to assess for the first time how much of the energy demand from buildings can be reduced throughout Switzerland. The results will guide and influence urban energy policies in the country and the developed generic data-driven methods can also be used for other countries. Finally, the Building Renewable Energy Database and the mapping tools will allow building owners, communities and investors to visualise energy savings and supply for individual buildings as well as for groups of buildings anywhere in Switzerland.
Research @EPFL
The activity of EPFL team is focused on the assessment of renewable energy generation potential for the built environment, with particular focus of rooftop solar photovoltaic (PV) and solar thermal (STC) energy generation. This work includes the estimation of solar horizontal irradiance at high spatial and temporal resolution, as well as the quantification of urban factors impacting PV and STC potential such as shading and available rooftop area. For the analysis, data driven and Machine Learning approaches (in particular Random Forests and Extreme Learning Machines) are combined with GIS tools to extract information from various sources including LiDAR-based surface models, 3D building data, satellite imagery and meteorological weather stations.
Near-future work includes the combination of the estimated PV potential at hourly resolution with additional renewable resources such as wind, to assess the potential of a hybrid renewable energy potential across Switzerland. Parallel activities include the forecast of future development of PV potential based on projected population statistics and climate scenarios, as well as a comprehensive assessment of the currently installed PV and STC capacity in Switzerland using the most advanced Convolution Neural Networks and semantic image segmentation techniques on aerial photo maps of Switzerland.
Research @UNIL
The activity of UNIL team is focused on the development and application of analytical tools for the spatio-temporal analysis and visualization of environmental multivariate data using cutting-edge approaches in (geo)statistics and data mining. In particular, we are extensively exploring high frequency wind speed data at local and global scales using both linear and non-linear tools such as spatio-temporal variography, Fisher-Shannon complexity, wavelet variance, random fractals, temporal point processes, Alan factor, and complex network science. At present, we are also working on fundamental methodological developments for the quantification of uncertainties using Extreme Learning Machines.
Nearest future work will involve geostatistical spatio-temporal predictions and simulations, as well as adaptation and application of advanced machine learning algorithms for deep exploration and forecasting of environmental data relevant to the renewable energy potential assessment.
Publications
Alina Walch, Roberto Castello, Nahid Mohajeri, Jean-Louis Scartezzini, Big data mining for the estimation of hourly rooftop photovoltaic potential and its uncertainty, Applied Energy, 262, 2020
DOI: 10.1016/j.apenergy.2019.114404
Roberto Castello, Simon Roquette, Martin Esguerra, Adrian Guerra, Jean-Louis Scartezzini; Deep learning in the built environment: automatic detection of rooftop solar panels using Convolutional Neural Networks, Journal of Physics: Conference Series, Vol 1343, 2019
DOI: 10.1088/1742-6596/1343/1/012034
Alina Walch, Roberto Castello, Nahid Mohajeri, Fabian Guignard, Mikhail Kanevski, Jean-Louis Scartezzini, Spatio-temporal modelling and uncertainty estimation of hourly global solar irradiance using Extreme Learning Machines, Energy Procedia Vol. 158, 2019
DOI: 10.1016/j.egypro.2019.01.219
Fabian Guignard, Dasaraden Mauree, Michele Lovallo, Mikhail Kanevski, Luciano Telesca, Fisher-Shannon complexity analysis of high-frequency urban wind speed time series, Entropy 21, 1, 47, 2019
DOI: 10.3390/e21010047
Alina Walch, Nahid Mohajeri, Jean-Louis Scartezzini, A critical comparison of methods to estimate solar rooftop photovoltaic potential in Switzerland, Journal of Physics: Conference Series, 1343, 2019
DOI: 10.1088/1742-6596/1343/1/012035
In the media
Bulletin.ch 10/20: Les données éclairent les toits suisses (auteur R. Castello)
RTS 3.2.20: La moitié des toits suisses pourraient produire de l’électricitéLFM 3.2.20: La moitié des toits suisses pourraient produire de l’électricité
24heures, 10.2.20: L’énergie photovoltaïque a du potentiel en Suisse
NZZ, 3.2.20: Jedes zweite Dach in der Schweiz wäre für Solaranlagen geeignet
lenouvelliste.ch: Energies: 50% des toits suisses peuvent produire de l’électricité
Arcinfo 3.2.20: Energie: 50% des toits suisses peuvent produire de l’électricité
Radio Lac, 3.2.20: La moitié des toits suisses peuvent produire du couranttechxplore.com: What if half of Switzerland’s rooftops produced electricty?
watson: Neue Studie zeigt: Jedes zweite Dach in der Schweiz taugt für Solarzellen
Project partners
|
![]() |
|
|
|