Contact: Roberto Castello
The activity, carried out in the context of SNSF and SCCER project grants, focuses on the assessment of renewable energy generation potential for the built environment, with particular emphasis on rooftop solar photovoltaic (PV) and solar thermal (STC) energy generation. For the analysis, data driven and Machine Learning approaches (in particular Random Forests and Extreme Learning Machines) are combined with GIS tools to extract information from various sources including LiDAR-based surface models, 3D building data, satellite imagery and meteorological weather stations. The final aim is to combine the estimated PV technical potential at hourly resolution with additional renewable resources such as wind and geothermal, to assess the potential of a hybrid renewable energy potential across Switzerland.
Parallel activities include the forecast of future development of PV potential based on projected population statistics and climate scenarios, as well as a comprehensive assessment of the currently installed PV capacity in Switzerland using the most advanced Convolution Neural Networks and semantic image segmentation techniques on aerial photo maps of Switzerland.
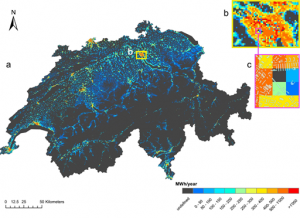