Théau Vannier

Description
Instadeep is a Franco–Tunisian start–up specialized in decision–making AI products for the Enterprise that was founded in 2014. It is now based all over the globe, namely London, Paris, Tunis, Cape Town, … The goal of the company is mainly to “accelerate the transition to an AI–First World that benefits everyone” to reuse their own words. It is to me a very interesting company that aims at using the more advanced skills and technologies we have nowadays to solve real life problems (bin–packing, “PCB”, BioAI, …). The company also aims at developing research environments like Jumanji, a suite of open–source Reinforcement Learning environments written in JAX providing clean, hardware–accelerated environments for industry–driven research. Finally, they also work on developing new methods and AI tools.
The DeepPCB team, with whom I worked, is working on solving and optimizing the PCB solution. Nowadays the PCB (printed circuit board) are manually designed by engineers. They sometimes use softwares to help them, but none is powerful enough to give a descent result and the engineers end up doing most of the job in designing them. DeepPCB is trying to find a solution to this problem using reinforcement learning. A PCB is a circuit board with chips like capacitance, USB port, … laying on it. The chips are also called the components and they have pins on them. Some pins must be connected to each other; a connected group of pins is called a net. Typically, a PCB can easily have up to 100 nets to connect.
The PCB problem can be seen as two subproblems:
• The routing: We give you a set of points (pins) in a 2/3–dimensional space, the net to which each pin belongs to and some physical constraints (obstacles, minimal distance between wires, etc…). The goal is to connect all the nets while minimizing some constraints (wire length, number of vias used, etc…)
• The placement: Here the goal is to spatially place the components such that the pins are optimally placed for the routing.
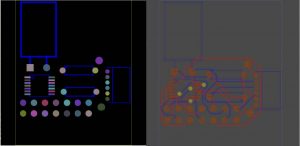
Ningwei MA
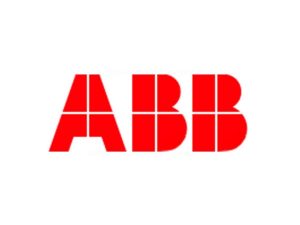
Description
ABB is a global technology company in electrification and automation. The company’s solutions connect engineering know-how and software to optimize how things are manufactured, moved, powered and operated. I worked at the cooperate research center which explores the new solution for engineering problems. The team I worked with is the software team, which aimed at modularizing an existing ABB electricity grid protection product. My work was to find or implement an in-memory key-value database that serves as a communication media among different modules. I first tried commercial in-memory database like Redis/Dragonfly but they were too slow for our industrial target, so we decided to implement a shared-memory-based hash map ourselves with the help of Boost library. After this was done, we tried to integrate it with the code base of the protection software and tried to optimize it, from the implementation aspect and algorithm of smartly distributing the keys and values to several hash maps to avoid contention. Once we finished a working fast shared-memory tool for each module process, we began to add different modules like Python module or specific recording module that monitors the change of the database. In the meantime, we were also optimizing the design of the system to simplify the interface of each module. After the backend worked fine, we add visualization tools like Grafana and used the data recorded in the recording module to show the change in the system over time. The project was done mostly in C++.
Giulia Mescolini
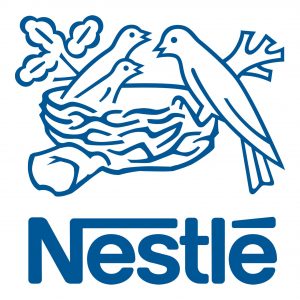
Description
Nestlé is the largest food and beverage company in the world, and Nestlé Research, based in Lausanne, is the division devoted to scientific research in various related fields, such as health & nutrition, food science, or materials. I worked within the Digital Health group, specialized in developing innovative digital solutions to support health and nutrition research and guide consumers to balance their nutrition or prevent diseases.
The aim of my project was the enrichment of the information on Nestlé food databases through Machine Learning tools. In particular, I worked at the classification of food descriptions according to the ingredients and the cooking methods, mapping them to labels organized hierarchically in a food ontology. For this task, I relied on Natural Language Processing Models, and had the opportunity to challenge myself with state-of-the-art techniques, but also with the development of strategies to handle complex and heterogeneous real data.
Moreover, I lived an involving experience at my workplace, learning the dynamics of teamwork and empowering my soft skills. I am specially grateful to the colleagues that I met for the welcoming environment that surrounded me during my internship.
Ali Garjani
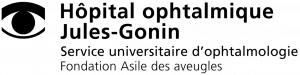
Description
Jules-Gonin Ophthalmic Hospital – Fondation Asile Des Aveugles is an eye hospital in Lausanne, and its roots go back to 1843. The hospital’s data science team explores projects involving using machine learning and data science to analyze medical images. During my internship, I worked on the prediction of disease recurrence in patients with Central Serous Chorioretinopathy (CSCR) from multimodal imaging. CSCR is the fourth most common eye disease considering the retina, and it typically occurs in males between their 20s to 50s who experience central vision loss or distortion. Although there are treatments that mitigate the symptoms of CSCR, there is still a chance of the recurrence of the disease. Hence having a tool to predict this recurrence can help the doctors to treat the patient before the disease goes to a critical stage. In my six-month internship, I worked on developing this tool, which consists of applying image processing and medical imaging techniques on raw scans to extract features and parameters, forming sequential data out of these features, and training a time-series deep model on the data to make the prediction. Figure below shows different models’ performance with receiver operating characteristic (ROC) curve.
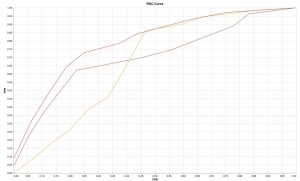
Paolo Motta
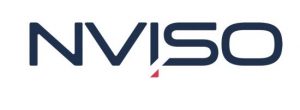
Description
NVISO is an artificial intelligence company founded in 2009 and headquartered at the Innovation Park of the ´Ecole Polytechnique F´ed´erale de Lausanne in Switzerland. It provides artificial intelligence solutions that can sense, comprehend and act upon human behavior using emotion analytics.NVISO’s products and services consist of applications, software development kits (SDK’s), and data services. These are used by NVISO customers to measure and increase productivity, and to accurately perform specific business functions, such as the automation of customer-facing operations. NVISO commercialization is focused on AI solutions for several key industries.
I have worked in the Research and Development (R&D) group in the field of Computer Vision, focusing on training deep learning models for various customer projects. Specifically, my projects dealt with object detection, facial action unit recognition, and body pose estimation. These models are crucial for various applications such as security systems, autonomous vehicles, and emotion recognition.
During my time in the R&D group, I was able to successfully develop deep learning models that achieved high levels of accuracy and performance on our datasets. The models were trained using a combination of convolutional neural networks (CNNs) and graph neural networks (GCNs) and were optimized using various techniques such as transfer learning and data augmentation. The main outcome of these projects were highly accurate deep learning models performing the tasks required by the clients, receiving positive feedback for their performances and accuracies. Additionally, I was also responsible
for the maintenance and improvement of the models over time, ensuring that they continue to meet the evolving needs of our customers.
Moritz Waldleben
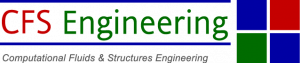
Description
CFS Engineering is a small company located at the EPFL Innovation Park with a mission to offer services in the numerical simulation of fluid and structural mechanics engineering problems. They specialize in aerodynamics and use their in-house Navier-Stokes Multi-Block solver to perform simulations on their servers.
My project was about mesh smoothing. A computational fluid mesh divides the field around an object into grid cells. These cells are then used in a computational fluid dynamics solver. Constructing complex meshes is not an easy task and is normally done with commercial software such as ICEM CFD. A standard way is to use transfinite interpolation (TFI) for the generation. After constructing an initial
mesh quality metrics such as skewness and boundary orthogonality can be improved with smoothing. I was working on elliptic mesh smoothing. In this procedure, elliptic differential equations are used to smooth existing TFI cells. I extended and further developed a Fortran program that should be integrated into their flow solver to improve a generated mesh in 3D.
Thomas Rimbot
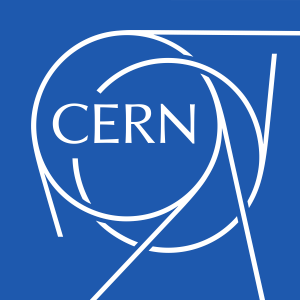
Description
CERN is the most prominent European research center in nuclear physics, providing researchers around the world with advanced technological tools to uncover the secrets of the universe, the most famous being the Large Hadron Collider (LHC). I did my internship in the TE-MSC-TM section (TEchnology department, Magnets, Superconductors and Cryostats group, Tests and Measurements section), under the supervision
of section leader Dr. Stephan Russenschuck. The goal was to develop and implement a generalized field description in strongly-curved accelerator magnets.
In practical applications, accelerator magnets are either straight or very slightly curved. The description of the magnetic field in the aperture is therefore developed with classical Fourier expansion in cylindrical coordinates, assuming a cylindrical geometry. However, if we move on to more curved magnets with bigger eccentricities, we expect this approximation to get worse, if not completely unusable. My goal was to formalize this framework and develop the theory in a more suited coordinate systems: the toroidal coordinates, with the expansion in toroidal harmonics. I derived and implemented formulas for their computation, compared them to the classical expansion, proved that the latter was completely wrong because of curvature, applied them to real test cases like the Extra Low Energy Antiproton ring (ELENA), and wrote a paper for publication together with the people I worked with.
Figure 1: ELENA magnet.
In particular, one of the main questions was about scaling laws. In a classical setting, the Fourier expansion allows us to compute the expansion coefficients (harmonics) on a reference circle inside the aperture of the magnet. However, these harmonics will only allow to reconstruct the field on this specific circle. Instead of recomputing the harmonics at every point inside the magnets, we can use scaling laws, which give us formulas to directly modify the computed coefficient and get the harmonics everywhere inside the aperture. The problem is that we expect these scaling laws to not hold in a curved setting. On the other hand, if we use the more suited toroidal harmonics, their scaling laws do in fact hold, allowing to reconstruct the field everywhere in the magnet.
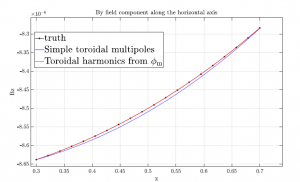
Figure 2: Scaling laws of the toroidal harmonics (red) vs the classical Fourier harmonics (blue) in stronglycurved
setting.
Fekih Selim
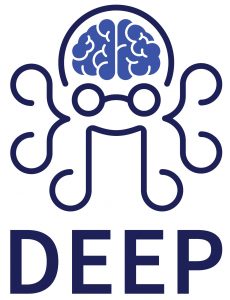
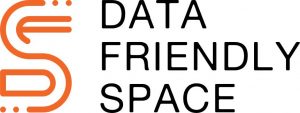
Description
I performed my master internship in Data Friendly Space , an INGO that works on providing humanitarian organizations with software development capacities and Machine Learning solutions. I worked on implementing NLP solutions for the Data Entry and Extraction Platform
(DEEP), a platform designed to perform secondary data review, by annotating documents and performing analysis on them. My internship’s objective was to help design NLP solutions to faster and optimize the analysis process for analysts.
More specifically, I worked on designing different NLP models, one for extracting relevant entries from a document and another for classifying relevant entries into a large predefined set of tags (referred to as analysis framework in the humanitarian sphere). The training data is
previously annotated data by humanitarian analysts. I contributed by helping make the models faster, less memory consuming and more accurate. This helped reduce costs for inference and make the models more helpful to humanitarian analysts.
Here is a visualization of some of the results we have on the Entry Classification task.
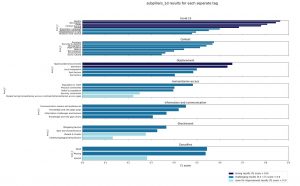
Anna Peruso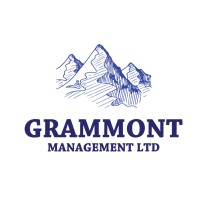
Description
I conducted my internship at Grammont Finance, in Lutry (VD). Grammont Finance SA is a small Swiss company specialised in financial engineering and trading. The main activity of the company is trading of Swiss equity and index derivatives in the EUREX market.
The goal of my project was to compare European and American options prices obtained by Heston’s stochastic volatility model with those obtained by the Local Volatility model, when the local volatility surface is indeed obtained by Heston prices. Both models find their roots in the attempt to overcome Black-Scholes’ unrealistic assumption of constant volatility and are an important tool for trading companies to better price options.
One typical approach to price derivatives in computational finance is to solve the associated parabolic PDE by means of Feynman-Kac theorem. My main assignment was to implement from scratch these two models in C++, together with a multi-dimensional Finite Difference solver for PDEs which was flexible enough to deal with both. Since many simulations needs to be run to price different options, much attention was given to find stable
and fast numerical schemes, in order to optimize computational costs.
Philippine des Courtils
Description
Metadvice is a 4-years old start-up located in St-Sulpice (VD) whose core activity is to build AI for health care systems, clinicians and pharma; it has offices England, the US and in Switzerland for the moment. More specifically, the company specialised in some major cardiometabolic and autoimmune diseases such as hypertension, diabetes Type II and rheumatoid arthritis along with cancer diseases, in collaboration with experts (professors and clinicians).
I first wrapped up a project centred around precision medicine for rheumatoid arthritis, in collaboration with a clinic in England. Using cleaned anonymized clinical data and medicine knowledge, my goal was to produce therapy recommendations for patients with transfer learning and fairly simple neural nets.
Justine Stoll
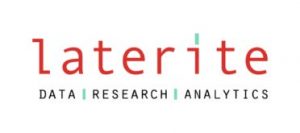
Description
Founded in 2010 in Rwanda, Laterite is a data, research, and technical advisory firm that helps clients understand and analyze complex development challenges in the social sector. Aside from its main activities, Laterite is developing Laterite.ai, a platform providing a collection of apps that researchers in the social sector can use to design better surveys, analyze data faster and explore new ideas.
Throughout my internship I worked on several of these tools. For example, one of the apps I contributed to aims at facilitating the processing and extraction of information from long lists of answers to open-ended questions. It is common to include open-ended questions, as opposed to multiple choice questions, in surveys. While these allow for a totally unbiased expression of opinions, answers are immensely harder to interpret and use than with multiple choice questions. In fact, if we ask the same open-ended question to 10,000 people, it is likely that we end up with 10,000 different answers, even though only say 5 or 6 themes are covered. The tool we developed, groups all answers to an open-ended question into clusters of common content, and summarizes each cluster. In this way, the researcher immediately has an overview of the themes that are covered in the answers.
The figure below shows the output of the app, where we analyzed 100 answers to the open-ended question “In your opinion, how could this education program be improved?”. Out of the 100 responses, only two underlying themes where identified and summarized.
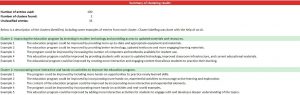
Patron Théo
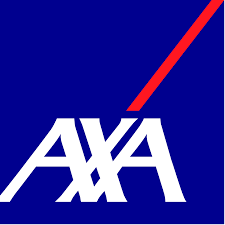
Description
During my internship, I had the opportunity to work at AXA, a French insurance company that is also heavily involved in technology research. I worked in a tech lab focused on computer vision research, where I collaborated with a team of interns and supervisors. My work included two main projects: one focused on flood mapping using satellite imagery, and the other on developing an internal tool to assist risk engineers.
In the first project, I was able to use my skills in Python and JavaScript to develop a prototype tool on Google Earth Engine that allowed us to dynamically compute metrics related to flood mapping and show computed flooded areas. I gained significant knowledge in how satellite imagery works, including both SAR and optical imagery, and used data from the Sentinel satellite. These skills have been of great use to me in my current thesis work.
In the second project, I worked as a software developer and data scientist in a team of 15 people using Scrum methodology. I learned how to write efficient, clean, and well–documented code in Python, how to work effectively in a team environment, and how to apply modern data science tools and techniques.
Overall, my experience at AXA was invaluable in terms of developing my skills and understanding of computer vision, data science, and software engineering.
Fadel Mamar Seydou
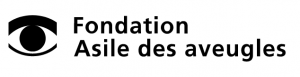
Description
The eye hospital Jules-Gonin or ”Fondation Asile des aveugles” is a 180 year-old privately owned
foundation . It has specialists in every domain of ophthalmology making it an impressive compact onestop solution for people with eye diseases. This makes it a reference in ophthalmology in Switzerland and Europe. The foundation is located in the heart of Lausanne at Avenue de France 15, 1002 Lausanne. It is a medium sized hospital with over 600 collaborators.
My role as an intern was to develop a deep learning model for the segmentation of atrophy lesion second to wet age-related macular degeneration (AMD). AMD is a disease that happens when the macula (i.e. a part of the retina) get damaged as the person gets older. It results in a loss of central vision and is currently the leading cause of irreversible blindness in the developed world. It is expected that by 2040, around 288 million people will suffer from it. Currently, no effective treatment exists making it an active area of research. In my case, I focused on the analysis of the ”wet” case as it is the most challenging one and no known segmentation algorithm from SD-OCT (i.e. spectral domain optical coherence tomography) scans exist. It is important to note that the dry AMD accounts for 80% of the cases. My work built up on a previous intern’s work and a publication by RetinAI (i.e. a partner and strong stakeholder in the project). I leveraged the publication done by RetinAI on the segmentation of atrophy lesions second to Dry AMD and extended the methodology to the Wet AMD.
The internship environment along with the deliverables expected from the intern made the experience very positive. It was very rich in learning. I was able to collaborate with an external stakeholder (RetinAI) and domain experts which helped get a better understanding of the responsibilities of a data scientist.
Figure 1 Example of hyperparameters search: tuning of learning rate. The selection of the best learning rate
—————————————————————————————————————–
Leonhard X. Driever
Company website: https://hypr-space.com/
Description
HyPrSpace, or Hybrid Propulsion for Space, is a young rocketry startup in southern France. Pushing for fast innovation and growth, the company aims to revolutionize European commercial spaceflight using a novel hybrid propulsion concept. I joined HyPrSpace with the objective of synergizing my bachelor’s degree in aerospace engineering with my masters in CSE.
My main task during the internship was the development of a simulation tool for the rocket’s cryogenic oxidizer tank. This required me to dive into the depths of thermodynamics, identify how to best simulate real gasses and supercritical fluids, and to combine all aspects in an efficient computational framework. The natural first step was a literature study, which brought me upon a simulation method developed at the NASA Ames Research center. After adapting the mathematics to the case at hand and incorporating features specific to the company’s design, I implemented the revised model and created a user-friendly interface for my colleagues. The simulation is efficient to run and the produced results have been validated through comparison with a software developed by the European Space Agency.
The image below shows sample results computed using my simulation program. The oxidizer tank is first filled, then pressurized, and finally emptied while maintaining the desired pressure. On the left-hand-side of the image, the temperature stratification within the tank is clearly visible. As expected for the given conditions, there is a clear downwards growth of the boundary layer within the tank ullage.
—————————————————————————————————————-
Haojun Zhu
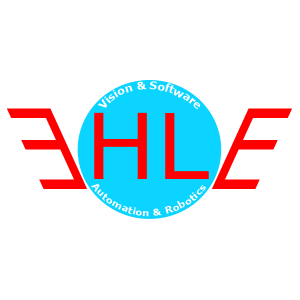
Description:
3HLE Automation & Robotics SA is a young and dynamic Swiss quality systems integrator and distributor specialising in industrial automation solutions. The company is mainly active in Switzerland but also successfully delivered services to multi-national partners and customers from prestigious brands. The company offers services in various areas, including the watch manufacturing industry, food production, medical services, and logistics operations.
My role as an intern was to use deep learning and computer vision techniques to solve industrial automation problems. My internship at 3HLE mainly focused on a robot arm control project. I first designed and wrote a control module for the integration of a Real-Time Data Exchange package to enable the communication between the PC and an UR robot. Then I implemented different commands to manipulate the robot arm that can eventually form various quality testing scenarios for the products from the client’s company. Finally I packaged all the utilities and distributed the product to the client. In addition to this, I also contributed to a small project using a ResNeT-based model to classify the food in the package and another project for object pose estimation.
This opportunity at 3HLE was very precious for me in terms of developing my general coding skills and understanding of software engineering and deep learning.
——————————————————————————————————————
Antoine Salaün
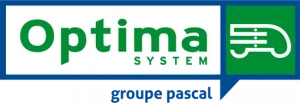
Description
My internship at Optima System, a truck conversion company, provided valuable experience in the field of truck conversions. With a reputation for delivering innovative and reliable solutions, Optima System specializes in transforming standard trucks into custom vehicles tailored to specific industry needs.
Under the guidance of Olivier Hutteau, the company’s co-director and main engineer, I developed an Excel sheet to compute load distribution on wheels after truck conversions. This tool facilitated the provision of detailed information to clients, enabling them to obtain legal authorization for road use.
During my internship, I collaborated with various departments, including procurement and production, gaining insights into material specifications and the manufacturing process. Working closely with Olivier, our regular meetings ensured a smooth workflow and successful project completion.
Through this experience, I enhanced my technical skills in Excel. I also developed problem-solving, analytical, communication, and organizational skills. Thesupportive environment at Optima System and Olivier’s guidance contributed
to a rewarding internship.
——————————————————————————————————————-
Laura Mismetti
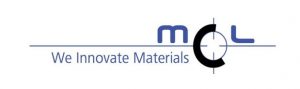
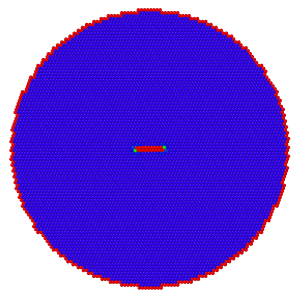
Initial fcc system (Aluminum) containing 2 partial Shockley dislocations
10μm far apart from each other.
Tomás Feith
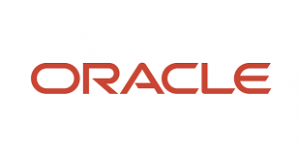
Description
Oracle is a multinational technology corporation founded in 1977. The company sells database software and technology, cloud engineered systems, and enterprise software products. Oracle Labs is a research and development organization within Oracle. I worked in the KeyBridge project, a multi-disciplinary project using expertise from systems-programming, data visualization and machine learning in order to understand massive streams of data and apply the lessons learned to systems operation.
The goal of my project was to develop a documentation recommender system, exploiting code representation models to find documentation pages containing similar code. I worked on improving a novel pre-training task to inject structural knowledge into models and prototyping a VSCode extension to expose the recommender system to users. Via quantitative and qualitative experiments, we showed our pre-training task is competitive with state-of-the-art models. At the end of the internship, I had a set of deliverables consisting of a fully functional VSCode extension prototype ready to move into production, and 6 invention disclosures detailing novel designs/techniques to be patented.
—————————————————————————————————————
Servane Lunven
Description
Merck KGaA is a German company actively engaged in research, development, and production of a diverse range of products and services, spanning pharmaceutical drugs, laboratory equipment, specialty chemicals, and innovative solutions for the life science industry. I worked in the Advanced Data Analytics team, a sub–team of the Quantitative Pharmacology division, whose aim is to develop machine learning algorithms to assess and predict the impact of treatments on patients.
My work consisted in one main project: implementing and training deep learning networks to predict the evolution in time of lesion sizes in a patient suffering from metastatic colorectal cancer. The data I worked with were real clinical data, which required a lot of preprocessing steps. Three different neural networks have been implemented for this time series forecast task: LSTM (Long Short–Term Memory), CNN–LSTM and Neural ODE, a recent model whose main advantage is to provide a continuous representation of the data, making it promising for handling dynamic and irregularly sampled clinical data. For each model, fine tuning, feature engineering and analysis of the obtained results were conducted. Overall, my internship at Merck provided me with valuable experience in data science and more particularly deep learning. It has also taught me how to efficiently present complex models to non–deep learning experts, either in writing or orally.
Manon Béchaz
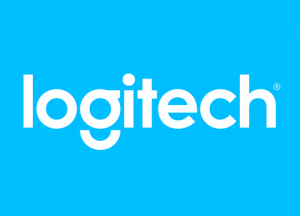
Description
Headquartered in Lausanne, Logitech is a manufacturer of software and computer accessories. Logitech develops a large variety of products, ranging from keyboards, mouse, tablet add-ons, to webcams, Bluetooth speakers, universal remotes, designed for both PC navigation, video communication and teamwork, and for gaming.
During this internship, I was integrated into the audio part of the AI team. Working ahead of R&D, the team explores and develops advanced technologies in the fields of speech and audio processing, whether it be denoising, speech enhancement, speech quality assessment… The goal of this internship was to investigate explicable, self-supervised deep learning methods for audio analysis. The idea was to explore methods that could help both to improve and to better understand Logitech’s models for audio analysis.
To this end, we followed two different approaches. At first, we considered hyperbolic geometry for neural networks, because such spaces have proven to be particularly suitable for data with hierarchical structure like audio. The results were promising, because hyperbolic networks almost always outperformed they standard counterparts, but we had to stop pushing further in that direction because of the lack of advances and scientific literature on the matter. We then focused on the choice of the training data and tested different methods of data pruning, with the goal of reducing the set of training data without compromising performance.
Charlotte Sertic
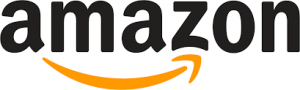
Description
During my internship, I worked at Amazon in the supply chain network sector as a Business Intelligence engineer. My team focused on inbound supply into EU and were tasked with optimising the inbound supply chain network. My internship focused on one main project, which was to optimise the inbound
supply chain network algorithm.
This project first consisted of understanding the interaction of different algorithms within the supply chain to understand how to optimise it. Then, one needed to gather data on this algorithm using large AWS datasets, querying using SQL. This was challenging as the data was hard to get and therefore
deep dives were also necessary to complete the full picture as well as assumptions.
One the data acquired, one needed to draft a business proposal on how to improve the supply chain distribution algorithm, backing it with data. This paper drove the project forward and enabled stakeholders to get onboard this new improvement. Finally, the improvement was tested in a randomised trial in production. This improvement was promising optimisation improvements driving annual savings of +8 figures. Moreover, since this improvement lead to a mean reduction of 3km of outbound distance per unit, this also lead to an overall reduction in CO2 emissions due to transport.
On the whole, I found this internship to be quite complete. It not only equipped me with technical skills, such as analysing large datasets, but also honed crucial soft skills like problem-solving and negotiation. The environment was friendly and fostered a culture of thinking big and nurturing curiosity. It was fascinating to be part of a large corporation and observe how the collaborative efforts of its members contribute to its remarkable
functionality.
Federico Betti
Description
Corintis is a Lausanne-based startup founded in February 2022 that is leading a breakthrough in semiconductor cooling solutions. Its cooling designs are directly embedded inside the chip and allow it to extract 10 times more heat compared to the current leading market alternatives. Mathematically speaking, for a given chip, Corintis designs performing microchannel geometries by means of topology optimization. The underlying Partial Differential Equation (PDE) constraints are the Navier-Stokes Equations for the motion of the fluid in the microchannels and the heat transfer equation through both the solid and the fluid layer. To reduce the computational resources associated with the solving of these equations, Corintis is exploring the application of Deep Learning and Reduced Order Models in order to create surrogate models of the governing physics of their problem.
The aim of my project was the investigation of Machine Learning techniques to accelerate the topology optimization processes, whose computational bottle- neck is represented by the evaluation of the underlying physics (in particular of the Navier-Stokes equations).
For this task, I relied on Graph Kernel Networks and Fourier Neural Oper- ators, which are state-of-the-art Deep Learning architectures that are able to learn differential operators from high-fidelity data.
Moreover, I lived an involving experience at my workplace, learning the dynamics of teamwork and empowering my communication and organizational skills. I am especially grateful to the colleagues that I met for the stimulating workplace I found myself immersed in, and for all the interesting research
discussions we had throughout the internship.
Pau Romeu Llordella
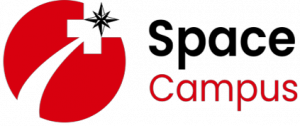
Description
Space Campus was founded in 2023 with the vision of fostering young talent in space technology research and development. My role there enabled collaboration with experts in the space domain. As of 2023, there have been more than 150 rocket launches, emphasizing the significance of having consistent and reliable information about such launches globally. A challenge identified was the inconsistency in launch communication by the organizations responsible. An approach to address this was to monitor aeronautical alerts, specifically NOTAMs (NOtice To AirMen), which are issued when airspaces are closed for rocket launches. The primary goal of my internship was to create a module for efficiently handling NOTAMs related to space activities. This was essential since thousands of NOTAMs are issued daily for various reasons, with no existing tool specifically focusing on space-related events. The culmination of this effort was a Python prototype capable of retrieving, processing, and filtering space-related NOTAMs. This tool not only archives historical launch-related NOTAMs but can also predict forthcoming launches based on the patterns in active alerts.
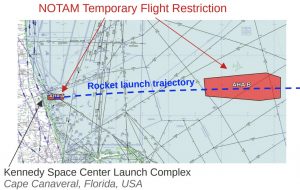
Valentin Comment
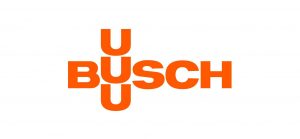
Description
Busch is a German company that was founded in 1963 specialized in the production of vacuum pumps and compressors. It then expanded globally around the world having 52 firms in 40 countries. One important technical data of vacuum pumps or compressors is the acoustic level they generate. Busch performs complex acoustic measurements with 9 microphones all around the pump. The current measurement process is long and tedious.
The aim of my project was to simplify their acoustic noise measurements by providing an application to assist the operators during their measures and to produce a final report with all the important information gathered in one Excel file. I developed this application using the graphic programming language Labview. I spent most of my days programming, testing, and debugging. During this internship, I had the opportunity to challenge my algorithms and software engineering skills and improved them a lot. In the end, the application was working perfectly well but was a bit too slow. I recommended possible modifications which could improve the situation. I also trained one Busch engineer about my Labview coding so that Busch could continue to improve and upgrade the tool.
The figure below shows a window of the application developed where some acoustic spectra are plotted in real time.
Philipp Weder
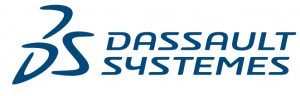
Description
Dassault Systèmes is a European multinational software company providing software for 3D design, simulation, manufacturing, and other 3D-related products. The company was created in 1981 to create 3D software to design aircraft, which nowadays is known under the name CATIA. Today, Dassault Systèmes counts 12 brands across 11 industries and provides solutions to over 250,000 companies worldwide. The company has revolutionized the industry several times over the years by introducing not only 3D design but also the 3D digital mock-up, 3D product life cycle management, and finally, the 3DEXPERIENCE platform, which is a collaborative business platform, allowing for the seamless integration of all software tools offered by the company. A few milestones which have been achieved thanks to these software solutions include the first-ever 3D protein structure model, the Boeing 777, the first 100% digitally designed jetliner, Virtual Singapore, a full 3D model of a city, and the living heart, the first-ever 3D model of a beating human heart enabling the personalized treatments of heart diseases. Dassault
Systèmes continues to push towards virtualizing the real world by integrating 3D modeling and
simulation into digital twins. A digital twin captures a real-world entity’s physical and functional
aspects. One target application for digital twins is healthcare, where they open the door to
personalized precision medicine and new ways of medical research.
During my internship, I worked at the Corporate Research and Sciences department of Das-
sault Syst `emes. More precisely, I worked in the Cardiology Twin Group, which works on digital
twin technology for the human heart and circulatory system for clinical applications. During
my internship, I managed to implement a state-of-the-art reduced-order modeling library from
scratch and physics-informed machine learning algorithms to estimate the models’ parameters.
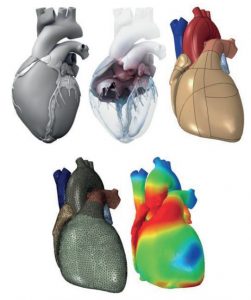
Bartul Kovacic
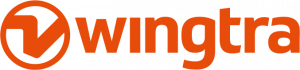
Description
Wingtra is venture-backed robotics scaleup with a global and international team of 180+ dedicated Wingtranauts who want to see their actions have a positive and lasting impact on the world. Founded more than 7 years ago at ETH Zurich, Europe’s leading robotics university, our goal is to build the best aerial robots to digitize the world at the push of a button and set the basis for faster and better decisions. Wingtra provides efficient and reliable data solutions to a variety of industries ranging from mining, construction and agriculture to humanitarian organizations, environmentalists and wildlife monitoring groups.
During my internship, I was a part of the embedded software team, a role I found particularly engaging due to its multifaceted nature, which necessitated frequent interaction with various other teams within the R&D department. In essence, my responsibilities centered around the implementation of control logic features for their product releases and conducting technical research for their next-generation drone.
One notable project involved the development of a user-facing failsafe strategy based on extensive data analysis. This strategy was subsequently released as a feature available to all Wingtra customers worldwide. Another task entailed extracting parametrized performance data and analyzing the benefits and impacts of replacing the inter-processor communication layer protocol with a faster and more reliable alternative. Additionally, I worked on tasks related to flight simulations, which required in-depth data analysis of detailed flight logs to identify and implement a wing servo current generation model.
Overall, this internship provided an excellent learning experience, allowing me to gain insights into various facets of embedded development, encompassing both software and electrical engineering aspects, as well as tools and techniques associated with both domains.
Emilien Seiler
Description
My internship at the Jules Gonin Hospital was very rewarding, providing me with a platform to cultivate both technical and professional skills in a multicultural, multidisciplinary environment. The group I was part of tackled ophthalmological research challenges through the lens of data science, employing cutting-edge deep learning and machine learning models. Situated within the ophthalmic hospital of Lausanne, we had direct access to medical experts, fostering invaluable collaboration.
The Jules Gonin Hospital, nestled within the ophthalmic center of Lausanne, stands as a beacon of excellence in ophthalmological research and care. With a distinguished history and a commitment to innovation, the hospital serves as a nexus for interdisciplinary collaboration between data scientists and medical experts.
My primary project focused on the detection and prediction of Central Serous Chorioretinopathy (CSCR) recurrences utilizing Optical Coherence Tomography (OCT) scans. This endeavor involved the implementation of intricate machine learning and deep learning models, including the adaptation of Vision Transformers for time series classification. The endeavor yielded significant results, culminating in the identification of critical biomarkers crucial for recurrence prediction.
These results are slated for imminent publication, a testament to the impact of our research. They will be disseminated through both my lab and the professor overseeing CSCR cases at the hospital, contributing to the broader academic and medical community.
Louis Philippe Valentin Poulain–Auzéau
Description
Internship at MeteoFrance – Enriching PEARO with GANs
Météo France is the national French agency for meteorology. The site I worked at is based in Toulouse. I worked within the CNRM, a research unit under the supervision of the CNRS and Météo France, in the GMAP/PREV team (Groupe de Modélisation et d’Assimilation pour la Prévision/Prévisibilité). This team is specialized in the predictability of meteorological phenomenon and develops tools, such as ensemble prevision, to help quantify the uncertainty of the forecasts.
The atmosphere is know for its chaotic nature. Thus, a simple way of exploring possible outcome from a given initial state consists in introducing a small perturbation to the initial conditions and to let the model give the prevision given the new initial conditions. This technique is referred to as ensemble forecasting. Very useful to predict extreme weather events, this technique is however very power consuming, only allowing for small sizes of ensemble previsions. This reduced size does not allow to explore fully the associated distribution of probability, in particular the rare events.
During this internship, I helped one Doctoral student on his thesis, which consists in enriching ensembles using Generative Adversarial Networks (GANs, Goodfellow et al., 2014). I mainly worked on this project with five other people (my supervisor, two interns and two engineers from the PNRIA).
My worked consisted in implementing post-processing codes to visualize the performance of our GAN (a styleGAN2 network, Karras et al., 2020) and launching experiments to understand the in-depth functioning on styleGAN. We took the architecture as implemented by its developers and modified it for our needs, i.e. generating meteorological variables. In the end, we obtained very good results and a lot of insights on how to modify the network to generate more complicated variables (such as very large scale variables). This works is currently continuing with another intern, working to generate the rain, a variable known for its difficulty to predict. An example of generation is presented in Figure 1.
Andreas Solheim
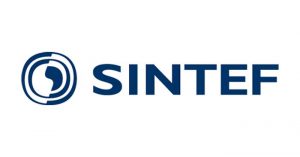
Description
SINTEF is one of the largest research institutes in Europe. The company is based in Norway
with locations in most major Norwegian cities. SINTEF is a non-profit company which aims to
contribute to the development of society through conducting research in the natural sciences,
technology, and health and social sciences. During the summer I worked in the computational
geosciences group based in Oslo focusing on solving problems involving nonlinear partial differential
equations. The group has a special focus on problems involving flow in porous media and
have developed several open-source software for solving such problems. During the summer I
worked on battery simulation as a part of the BattMo project which is a simulation software
for electrochemical devices.
There are currently two versions of BattMo: one in Matlab and one in Julia. BattMo-
Matlab is based on MRST which is an open-source software for reservoir simulation developed
by the group I worked in. Many of the techniques that are used to solve problems in reservoir
modeling can also be applied to batteries. BattMo-Julia is based on Jutul which is a framework
for fully differentiable multi-physics solvers. While the Julia version of BattMo is faster, the
Matlab version is more complete from a physics perspective and development is ongoing on both.
My work during the summer focused on two main tasks. The first was to implement support
for higher order DAE solvers in the BattMo project in Julia and Matlab. In particular I explored
solvers like ode15i in Matlab and various solver options in the DifferentialEquations.jl package in
Julia. I was able to create a baseline functioning implementation which will later be optimized
by the researchers in the group and hopefully eventually improve the performance of BattMo.
My second task during the summer was to implement an interface between BattMo-Matlab
and BattMo-Julia which would enable running parts of the Julia code from Matlab. The main
motivation for such an interface is to enable researchers to access the speed of Julia while using
Matlab for pre- and post-processing. I was able to solve this task by relying heavily on the
DaemonMode.jl package, which allows running a persistent Julia server as a background process
which can be called upon when needed. This interface will hopefully prove a useful tool for
researchers when performing parameter sweeps to test different battery configurations.