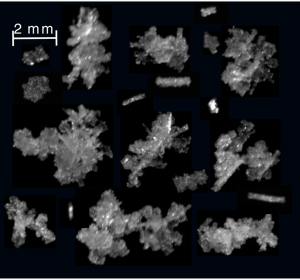
Snowflake pictures taken by the MASC instrument, from Gehring et al. 2020
Field campaign during the PyeongChang2018 Olympic Winter Games
From November 2017 to March 2018, LTE participated to the international field campaign « ICE-POP 2018 » to support the PyeongChang2018 Olympic Winter Games. We installed an X-band dual-polarization Doppler radar (MXPol), a W-band Doppler cloud profiler (WProf), a multi-angle snowflake camera (MASC) and a two-dimensional video disdrometer (2DVD). On 28 February 2018 an intense snowfall event cumulating 57 mm of equivalent liquid precipitation took place in PyeongChang. This event was associated to a so-called warm conveyor belt (WCB), which is a warm and moist airstream ascending along the cold front of an extratropical cyclone. WCBs are known to be the primary cloud and precipitation producing feature associated with extratropical cyclones. To better understand how this WCB produced such an intense snowfall, we studied how it influenced the precipitation growth processes by analyzing the data we collected. We found that the production of supercooled liquid water was favored by the strong ascent in the WCB, which promoted the riming process (accretion of supercooled water droplet on snowflakes). Moreover, the strong turbulence inside the WCB air masses enhanced aggregation by increasing the probability of collision between particles, creating large aggregated snowflakes. The figure below illustrates the findings of this study.
Conceptual model summarizing the key findings. From Gehring et al. 2020.
Gehring, J., Oertel, A., Vignon, E., Jullien, N., Besic, N., and Berne, A.: Microphysics and dynamics of snowfall associated with a warm conveyor belt over Korea, Atmospheric Chemistry and Physics, 20, 7373–7392, https://doi.org/10.5194/acp-20-7373-2020, https://www.atmos-chem-phys.net/20/7373/2020/, 2020
Understanding snowfall with machine learning
Atmospheric ice and snow processes play an important role in the formation of clouds and precipitation, and therefore the weather and climate of the Earth. In order to gather data about these processes, LTE, along with other groups around the world, has deployed automatic camera systems to different regions of the world to take high-resolution photographs of falling snowflakes. The snowflake images can help us understand the processes that created them. In total, millions of images have been produced, many more than can be analyzed manually, so we need automated analysis using computers in order to make use of the data. LTE has approached this problem using modern machine learning, which has revolutionized image processing in the recent years.
We have developed a method for classifying snowflake images automatically with minimal human input using generative adversarial networks, an unsupervised machine learning method that uses neural networks. The method analyzes a snowflake dataset and separates it into distinct classes, each consisting of similar snowflakes. This will help us analyze our snowflake image data more thoroughly and to understand the similarities and differences of precipitation processes in various regions.
Publication
LTE is also working on using neural networks to reconstruct the three-dimensional structure of snowflakes from two-dimensional images. This will help us better understand the structure, and consequently the aerodynamic properties of falling snow.
Researchers: Josué Gehring, Jussi Leinonen