Quantitative precipitation estimation in Switzerland
Quantitative precipitation estimation (QPE) is important for many hydrological, meteorological and climatological studies. Precipitation is spatially and temporally highly heterogeneous, and the complex topography of Switzerland renders accurate and reliable QPEs even more challenging. To be able to cover large areas at a high temporal resolution, QPEs are typically derived using weather radar measurements.
The Federal Office of Meteorology and Climate MeteoSwiss maintains five operational C-band dual-polarized radars in Switzerland. These radars provide information on hydrometeors within the atmosphere. Currently, the Swiss QPE is derived by using the single polarimetric radar measurements of these operational radars and converting them to rainfall estimates through a reflectivity-rainfall parameterization (Z-R relationship) or by kriging radar-based estimates with gauge observations.
In this research, we apply a machine learning approach to derive precipitation amounts from the dual-polarized radar measurements. More specifically, we use the horizontal (Zh)– and vertical (Zv) reflectivity, specific differential phase shift (Kdp), copolar correlation coefficient (ρhv), spectral width, coverage of each radar, visibility, height of the radar measurements and the 0° isothermal altitude as input into the random forest model. This random forest model (RainForest) was trained to match gauge observations. RainForest outperforms the more traditional way of converting reflectivity to rainfall rates. In addition, the approach is easy to parallelize and thus allows for a fast computation of near real-time precipitation estimation in Switzerland at a 5 minute and 1×1 km resolution.
Further research will look into deriving uncertainties and ensemble members from this random forest algorithm. Moreover, solid precipitation will be specifically investigated.
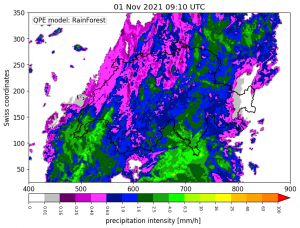
Researcher: Rebecca Gugerli
Cloud and precipitation monitoring with geostationary satellites
This project aims to enhance the monitoring of precipitation across vast areas of the globe, which is currently challenging due to sparse rain gauge networks and limited ground weather radars. Traditional methods of estimating precipitation at a global scale are complex and rely on merging data from a constellation of passive microwave (PMW) satellites. However, the revisit time of low-orbit (LEO) PMW satellites is too long to capture the fast-paced variability of precipitation and cloud systems.
To overcome this limitation, the project involves the use of cutting-edge artificial intelligence techniques and geostationary (GEO) spaceborne instruments with unprecedented observational capabilities. By doing so, the research aims to characterize precipitation structures and dynamics at high spatial and temporal resolutions.
The project’s first phase concerns the creation of the GPM-GEO analysis-ready dataset that spatio-temporally collocates third-generation GEO satellite multispectral VIS/IR imagery, precipitation and cloud products, with precipitation measurements acquired by the Global Precipitation Measurement Mission (GPM) Dual-frequency Precipitation Radar (DPR) (see the figure below for a collocation illustration). This dataset will facilitate the synergistic and collaborative use of multiple remote sensing data sources, enable a broad range of cloud and precipitation studies, and support the development of new GEO precipitation products.
The software and datasets developed in the context of this research are publicly available at the following links:
- GPM-API: https://github.com/ghiggi/gpm_api
- GOES-API: https://github.com/ghiggi/goes_api
- HIMAWARI-API: https://github.com/ghiggi/himawari_api
GOES-16 True Color Image with (overlayed) GPM-DPR precipitation estimates
Researcher: Gionata Ghiggi