Accurate 3D human pose estimation from single images is possible with sophisticated deep-net architectures that have been trained on very large datasets. However, this still leaves open the problem of capturing motions for which no such database exists. Manual annotation is tedious, slow, and error-prone.
In this project, we propose to replace most of the annotations by the use of multiple views, at training time only. We propose the following two alternative methods.
Learning Monocular 3D Human Pose Estimation from Multi-view Images
In this pose-centered approach, we train the system to predict the same pose articulated pose in all available views. Such a consistency constraint is necessary but not sufficient to predict accurate poses. We therefore complement it with a supervised loss aiming to predict the correct pose in a small set of labeled images, and with a regularization term that penalizes drift from initial predictions. Furthermore, we propose a method to estimate camera pose jointly with human pose, which lets us utilize multi-view footage where calibration is difficult, e.g., for pan-tilt or moving handheld cameras. We demonstrate the effectiveness of our approach on established benchmarks, as well as on a new Ski dataset with rotating cameras and expert ski motion, for which annotations are truly hard to obtain. | ![]() |
The new Ski-pose PTZ-camera dataset is available here: Ski-PosePTZ-Dataset.
Pre-print: https://arxiv.org/abs/1803.04775
Unsupervised Geometry-Aware Representation for 3D Human Pose Estimation
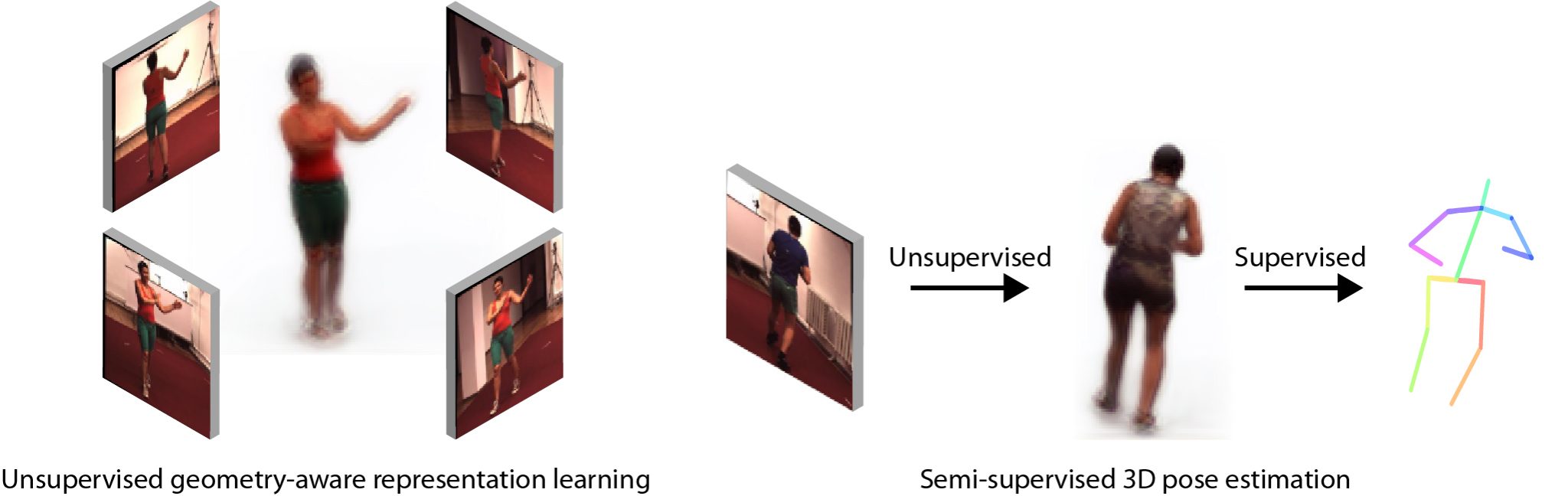
In this approach, we propose to overcome remaining problems by learning a geometry-aware body representation from multi-view images without any 3D annotations. To this end, we use an encoder-decoder that predicts an image from one viewpoint given an image from another viewpoint. Because this representation encodes 3D geometry, using it in a semi-supervised setting makes it easier to learn a mapping from it to 3D human pose. As evidenced by our experiments, our approach significantly outperforms fully-supervised methods given the same amount of labeled data, and improves over the first semi-supervised method while using as little as 1% of the labeled data.
Pre-print: https://arxiv.org/abs/1804.01110
Pytorch network definition and training code: github.com/hrhodin/
References
Please note that the publication lists from Infoscience integrated into the EPFL website, lab or people pages are frozen following the launch of the new version of platform. The owners of these pages are invited to recreate their publication list from Infoscience. For any assistance, please consult the Infoscience help or contact support.