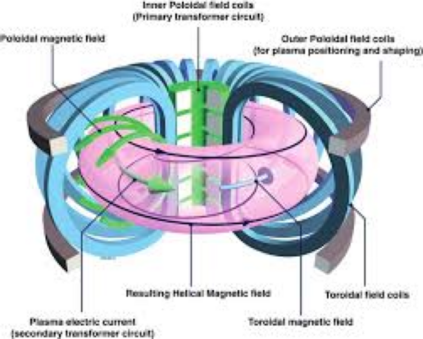
An active research field is the prediction of physics mechanisms degrading plasma confinement and performance, eventually leading to disruptions on Tokamaks. Disruptions are the ultimate consequence of highly coupled non-linear plasma physics processes, resulting in an abrupt loss of plasma current and confinement inducing huge electromagnetic forces and thermal loads on Plasma Facing Components and surrounding structures, potentially causing serious damages to the machine. Their causes for some of their types are not yet well understood, and no first principle models able to exhaustively describe disruption mechanisms exist. They represent probably the highest concern for large scale fusion reactors, and their prediction, avoidance and mitigation are a critical need for the success of next-step fusion devices.
In this project, we will explore different ML methods, such as hybrid schemes including supervised, semi-supervised, and unsupervised ones to predict such catastrophic events.
Key Domains
- Deep-Learning
- High-energy physics
Prerequisites
- Knowledge of machine learning, deep-learning.
- Background in physics.
- Proficiency in Python.