One of the general goals in our methodological development is to incorporate physics-based modeling ideas into machine-learning models of chemistry and materials. This takes the form of both learning complicated properties, such as those related to the electronic structure of a material, and of building models that reflect basic physical ideas, such as locality and range separation.
Machine-learning for electronic structure
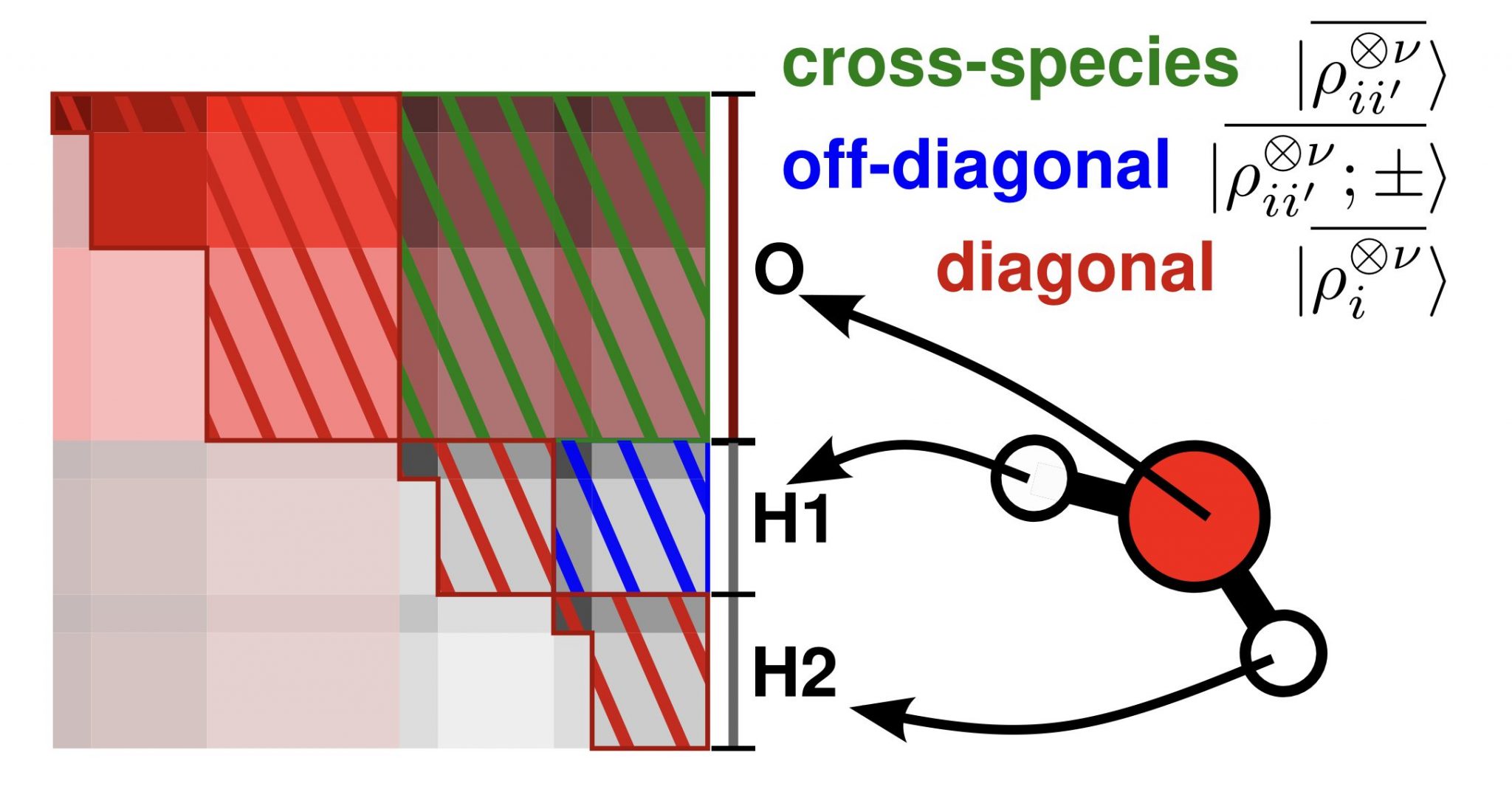
We develop strategies to learn key electronic properties, like the electronic density of states or the electronic charge density, that can be combined with physical theories to predict further observables. Another direction we pursue is to target, in a symmetry-adapted fashion, the effective single-particle Hamiltonian of a system, usually expressed on an atomic-orbital basis.
Locality and long-range physics modeling
Examples range from the inclusion, in machine learning models, of electronic excitations effects on the phase diagrams of metals and warm dense matter to the prediction of electrostatic screening properties of liquid electrolytes under finite electric fields. We also develop new approaches to capture long-range (Coulomb) interactions into machine learning models while keeping a description based on local environments.
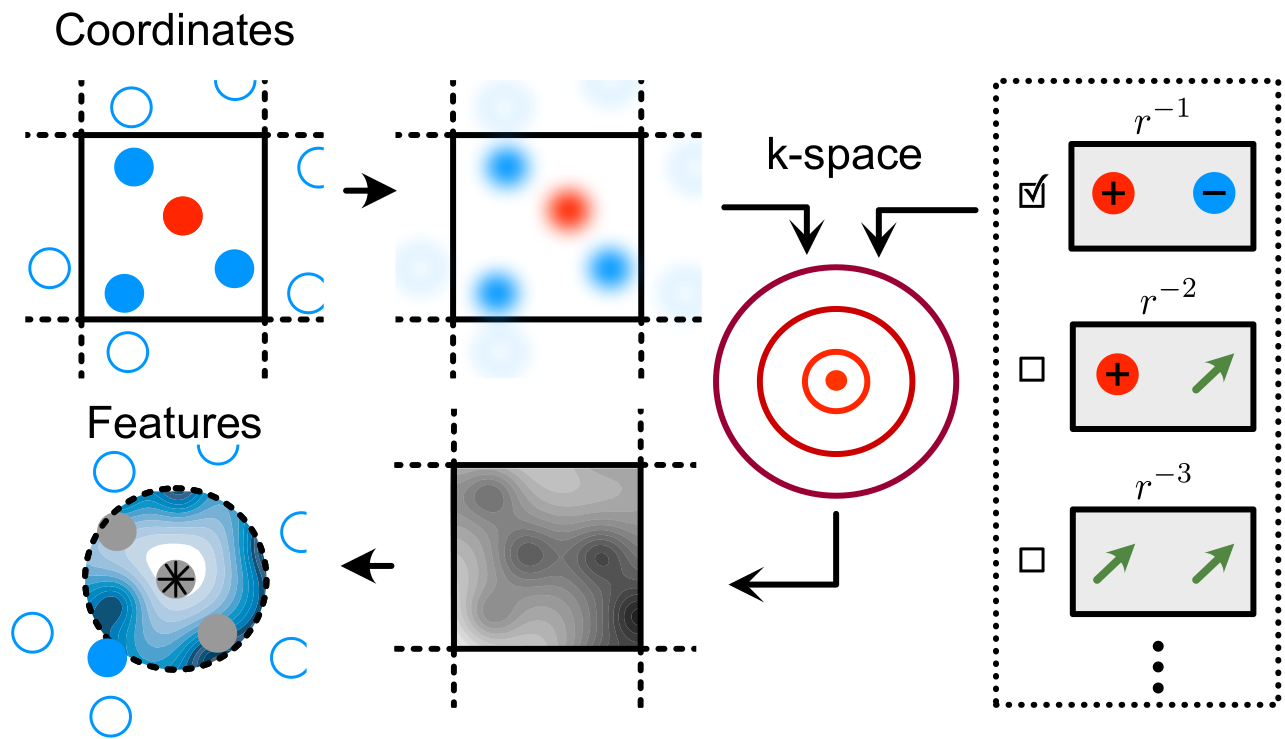
This line of research has strong interconnection with the FIAMMA grant funded under the Horizon 2020 program of the European Research Council.