At COSMO we perform bottom-up atomistic modeling of condensed matter and molecular systems, focusing on method development and statistical sampling. Our goal is to understand the mechanisms that underlie the macroscopic properties of materials and improve them through rational design.
We tackle this challenge through a combination of fundamental science and data-driven approaches. By incorporating physics and chemistry ideas into machine learning methods we build accurate and predictive surrogate models. These models allow us to run reliable simulations that bridge different time and size scales and capture subtle physical effects and complex, large-scale structural features.
We employ these simulations as a tool of discovery: by improving the fidelity of the simulations, we aim to achieve a better understanding of materials’ properties and a reliable description of physical reality. As we believe that research is a collective endeavour, we develop open-source tools for the benefit of other researchers and the larger scientific community.
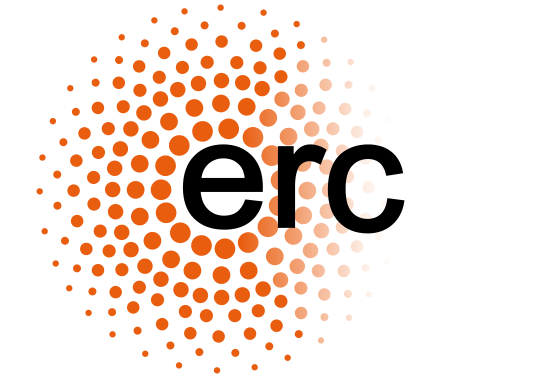
Creating a “plug and play” framework to combine seamlessly machine learning with physics-based modeling, funded by the European Research Council
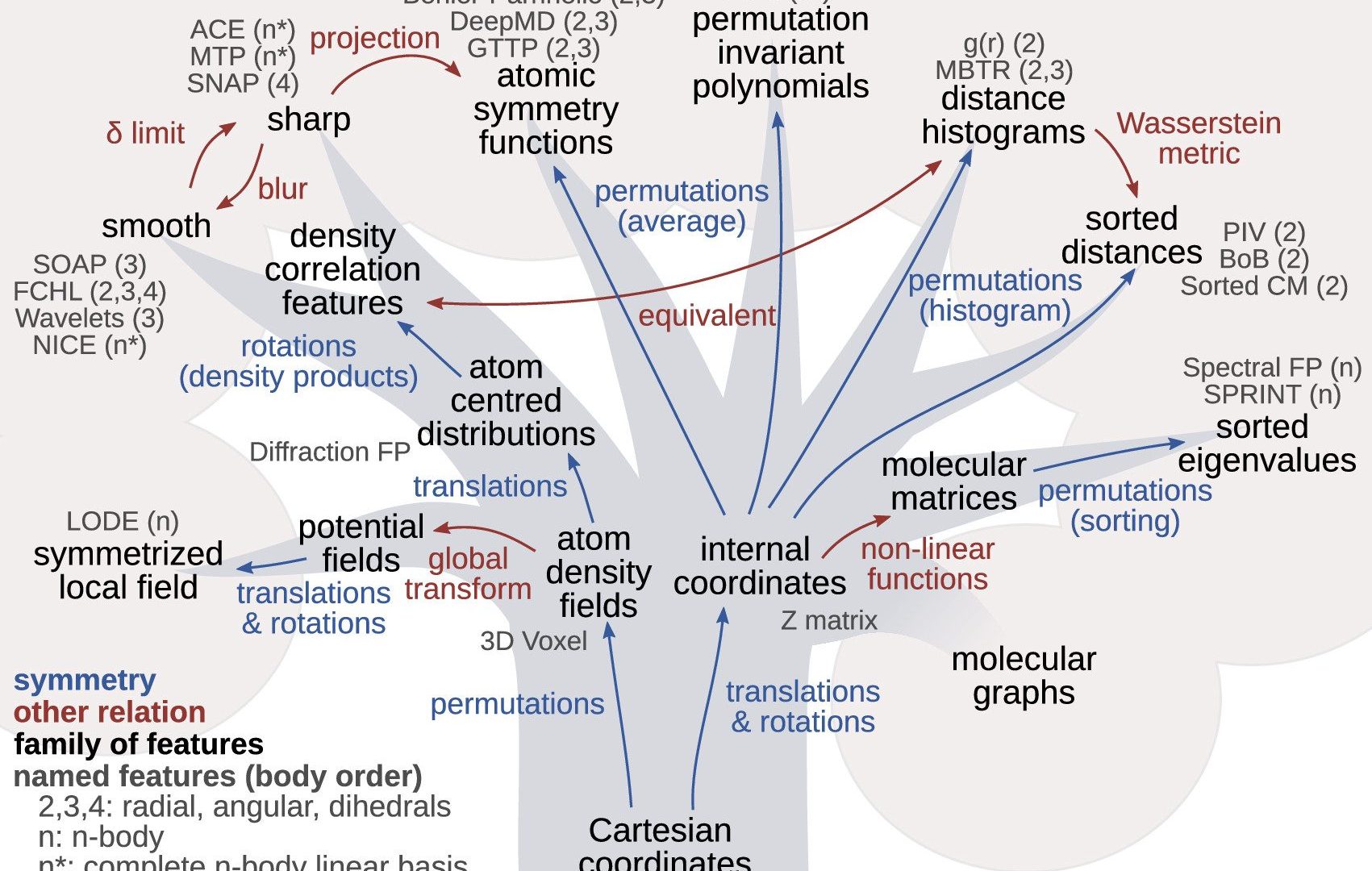
Investigating fundamental questions related to the mathematical data-driven modeling of matter at the atomic scale