The main task of materials science is to obtain detailed and accurate properties, and structure of materials. Quantum calculations today provide the most accurate results, but this accuracy is paid for by high computational costs. Machine learning allows to receive inexpensive approximation of quantum calculations. The key issues here are determining the models that give the best results, as well as representations of atomic structures in a mathematical format.
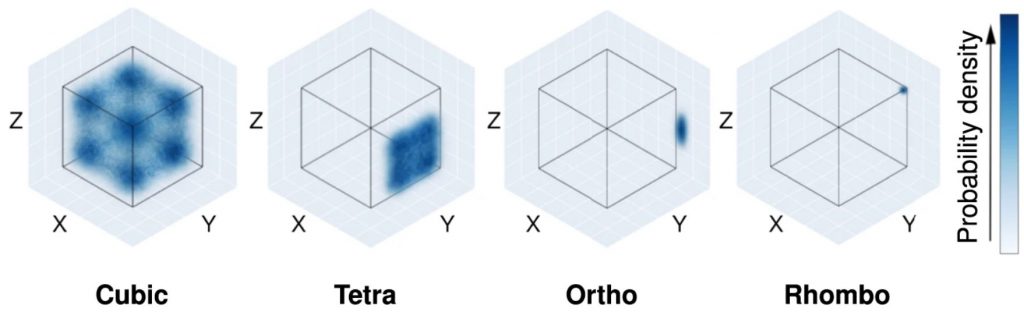
In our group we currently develop surrogate models to simulate:
- High-entropy alloys, characterized by a huge number of different chemical species whose interatomic potentials are learnt via alchemical learning
- Solid-state electrolytes for batteries, to study their electrochemical stability as well as their charge and thermal transport properties at different thermodynamic conditions
- Ferroelectric materials for which we develop machine learning models to learn the bulk polarization and study their phase stability.
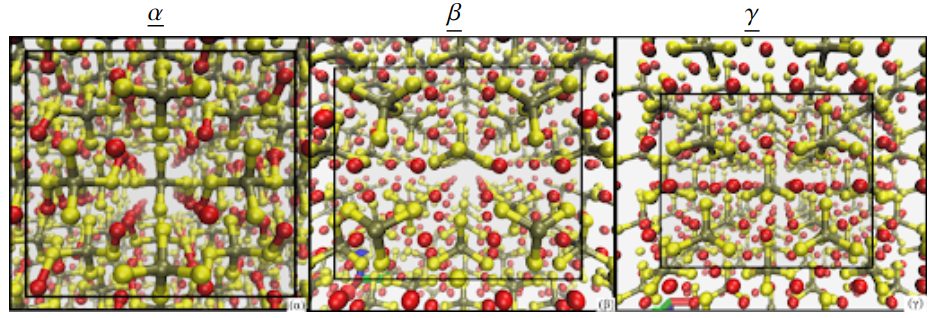