Voice-leading schemata are common note patterns that can be found in various musical styles ranging from Renaissance, Baroque and Classical to modern Popular and Jazz music. A notorious difficulty when dealing with patterns in music is the highly irregular, multidimensional structure of music as opposed to, for example, text. In particular, finding these patterns when they occur as a variation or in polyphonic music has hitherto been impossible for computational approaches. As a novel approach to this problem, we developed an algorithm that instead considers the relation of different notes to one another and their development. Without the limitations of conventional approaches, these generalized skipgrams (also called polygrams) can form the basis for a wide range of techniques, such as finding occurrences of a given schema in a piece of music.
In relation with the skipgram matcher, we have created a compendium of schemata. First designed as an annotation manual to guide and provide the necessary information for human annotators to collect ground-truth data, this manual turned out to be much more. This compendium includes the formalisation of 26 schematas for a total of 150 subtypes of schematas . Each schema is described according to a formal description (number of stages, numbers of voices, mode, harmonic signature) and to its musical features. A series of prototype is then given for each variations of the schema, following a standard notation that we have developed. Already annotated real-life example are also provided.
In order to gather ground-truth data, we also have developed an annotation software. The development of this software went through several versions, increasingly more user-friendly. We have settled on using an online application for its simplicity of use and accessibility for the annotators. No particular software is needed to be installed on the annotators’ side, only a regular web browser, and therefore this annotation tool is available on all platforms.
Both hand annotation and matches generated by the schema matcher share the same encoding format. This way they can be used in order to train models of machine learning to improve the schema matcher. In that regard we started implementing several features of rhythmic and pitch similarities, to create models that will then be trained on the ground truth and optimised using a logistic regression.
In the figure below, we show a screenshot of the annotation web application with schema suggestions from the schema matcher.
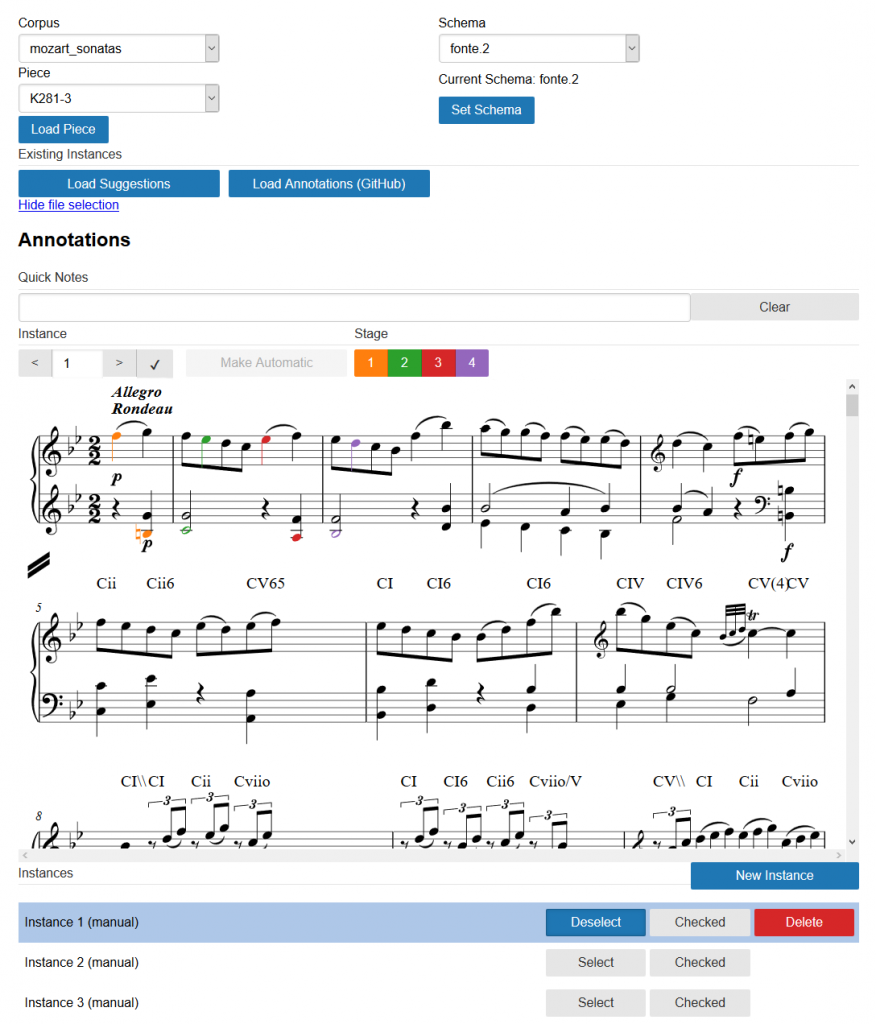