Large-scale scientific spatial data
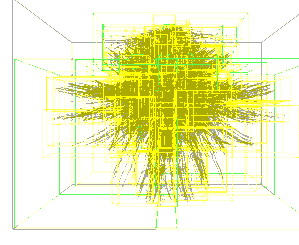
Scientists in disciplines like biology, chemistry, physics etc. produce vast amounts of data through experimentation and simulation. The amounts of data produced are already so big that they can barely be managed. And the problem is certain to get worse as the volume of scientific data doubles every year. In the DIAS laboratory we are working on next generation data management tools and techniques able to manage tomorrow’s scientific data.
We study large spatial databases and are particularly interested in:
- Designing space- and query-efficient access methods to facilitate common scientific tasks.
- Designing adaptive algorithms and index structures that efficiently identify and extract useful information from massive amounts of data in an ad-hoc manner.
- Developing data approximation techniques with low representation and complexity overhead to enable interactive data exploration.
Publications
Time- and Space-Efficient Spatial Data Analytics
Lausanne, EPFL, 2019.Space Odyssey – Efficient Exploration of Scientific Data
2016. 3rd International Workshop on Exploratory Search in Databases and the Web, San Francisco, California, USA, June 26 – July 1, 2016. p. 12-18. DOI : 10.1145/2948674.2948677.
Urban analytics
The recent explosion in the number and size of spatio-temporal datasets from urban environments and social sensors creates new opportunities for data-driven approaches to understand and improve cities. Visual analytics systems aim to empower domain experts to explore multiple datasets, at different time and space resolutions. Since these systems rely on computationally intensive spatial aggregation queries that slice and summarize the data over different regions, an important challenge is how to attain interactivity. To that end, we develop techniques that leverage the rendering pipeline of the graphics hardware (GPU) to evaluate queries on the fly at interactive speeds.
Collaboration: the results of this work was a collaboration with the VIDA lab at NYU.
https://github.com/VIDA-NYU/raster-join
Publications
Interactive Visual Exploration of Spatio-Temporal Urban Data Sets using Urbane
2018. ACM SIGMOD International Conference on Management of Data, Houston, Texas, USA, June 10-15, 2018. DOI : 10.1145/3183713.3193559.Point cloud data management
Nowadays, massive amounts of point cloud data can be collected thanks to advances in data acquisition and processing technologies like dense image matching and airborne LiDAR scanning. With the increase in volume and precision, point cloud data offers a useful source of information for natural resource management, urban planning, self-driving cars and more. At the same time, the scale at which point cloud data is produced, introduces management challenges: it is important to achieve efficiency both in terms of querying performance and space requirements. By leveraging point cloud characteristic and spatial proximity we design time- and space-efficient solutions to storing and managing point cloud data in the context of main memory column-store systems.
Publications
Dictionary Compression in Point Cloud Data Management
2017. 25th ACM SIGSPATIAL International Conference on Advances in Geographic Information Systems, Los Angeles Area, CA, USA, November 7-10, 2017. DOI : 10.1145/3139958.3139969.