The ongoing data explosion necessitates that the database software efficiently uses the available hardware and exploits data properties, to enable timely business intelligence. Additionally, data and hardware become increasingly heterogeneous: modern servers are adopting a variety of hardware accelerators to increase their energy efficiency while data scientists analyze a wide variety of heterogeneous datasets to gain insights. In this line of work, we are enabling query engines to adapt to the available hardware and data formats as well as automatically exploit domain-specific properties by generating specialized query engines on demand, achieving the performance of specialized engines without the extra development effort and time.
Analytical engines with Context-Rich Processing
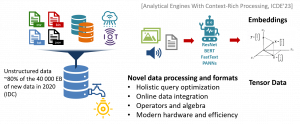
As modern data pipelines continue to collect, produce, and store a variety of data formats, extracting and combining value from traditional and context-rich sources such as strings, text, video, audio, and logs becomes a manual process where such formats are unsuitable for RDBMS. To tap into the dark data, domain experts analyze and extract insights and integrate them into the data repositories. This process can involve out-of-DBMS, ad-hoc analysis, and processing, resulting in ETL, engineering effort, and suboptimal performance. While AI systems based on ML models can automate the analysis process, they often further generate context-rich answers. Using multiple sources of truth, for either training the models or in the form of knowledge bases, further exacerbates the problem of consolidating the data of interest.
We envision an analytical engine co-optimized with components that enable context-rich analysis. Firstly, as the data from different sources or resulting from model answering cannot be cleaned ahead of time, we propose using online data integration via model-assisted similarity operations. Secondly, we aim for a holistic pipeline cost- and rule-based optimization across relational and model-based operators. Thirdly, with increasingly heterogeneous hardware and equally heterogeneous workloads ranging from traditional relational analytics to generative model inference, we envision a system that just-in-time adapts to the complex analytical query requirements. To solve increasingly complex analytical problems, ML offers attractive solutions that must be combined with traditional analytical processing and benefit from decades of database community research to achieve scalability and performance effortless for the end user.
Query Approximation
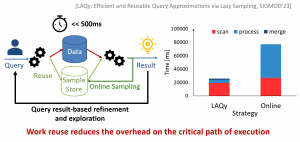
Modern analytical engines rely on Approximate Query Processing (AQP) to provide faster response times than the hardware allows for exact query answering. However, existing AQP methods impose steep performance penalties as workload unpredictability increases. Specifically, offline AQP relies on predictable workloads to create samples that match the queries in a priori to query execution, providing reductions in query response times when queries match the expected workload. As soon as workload predictability diminishes, existing online AQP methods create query-specific samples with little reuse across queries, producing significantly smaller gains in response times. As a result, existing approaches cannot fully exploit the benefits of sampling under increased unpredictability.
At DIAS we analyze sample creation and propose a framework for building, expanding, and merging samples to adapt to the changes in workload predicates. Our framework speeds up online sampling processing as a function of sample reuse, ranging from practically zero to full online sampling time and from 2.5x to 19.3x in a simulated exploratory workload.
Data Cleaning
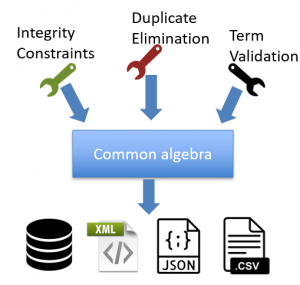
Data cleaning has become an indispensable part of data analysis due to the increasing amount of dirty data. Data scientists spend most of their time preparing dirty data before it can be used for data analysis. At the same time, the existing tools that attempt to automate the data cleaning procedure typically focus on a specific use case and operation and are unaware of the analysis that users perform. Thus, specialized tools exhibit long running times or fail to process large datasets. In this project, we focus on approaches that address the coverage and performance issues of data cleaning, while also integrating data cleaning tasks seamlessly into the data analysis process.
Elastic & Distributed Query Engines
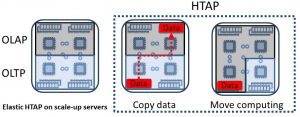
We build transactional and analytical engines that leverage native cloud functionality, such as elasticity and distribution. We provide fine-grained elasticity through cross-cutting system designs, spanning throughout the whole software virtualization stack, whereas we build our distributed query processing systems on top of Spark and other parallel frameworks.
Modern Storage
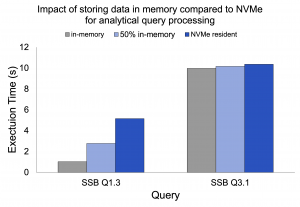
Storage hardware has improved dramatically in the past decade. It is now possible to have storage bandwidths in the hundreds of GB/s on a single server, approaching memory bandwidth. Conventional analytical engines rely on in-memory caching to avoid disk accesses and provide timely responses by keeping the most frequently accessed data in memory. However, high bandwidth storage performance is sufficiently close to memory bandwidth so that storing the input data on HBS can be as fast as full in-memory processing for many workloads. In this line of work we explore how high performance analytical systems must be redesigned for the high bandwidth era.
Query Accelerators
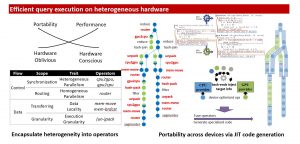
Traditionally, query engines are optimized for CPUs, but nowadays modern servers are becoming increasingly heterogeneous and equipped with multiple hardware accelerators, like GPUs. In this line of work, we investigate how different accelerators can be used by the query engine to increase its performance as well as provide isolation between queries. We design new hardware-conscious algorithms, study how existing ones perform across different micro-architectures and investigate multi-device query execution. Lastly, we provide engine designs that generalize device-specific approaches to achieve efficient heterogeneous-device execution through just-in-time code generation.