Wireless Imaging for Autonomous Systems
Achieving fully autonomous vehicles requires the ability to operate in severe weather conditions such as dense fog, smog, snowstorms, and sandstorms. Autonomous vehicles, today, mainly use cameras or LiDARs which suffer in low visibility conditions and bad weather. Our work demonstrated the ability to achieve high-resolution wireless 3D imaging and object detection for self-driving cars even in poor visibility conditions such as fog where cameras and LiDARs fail. The work consolidated recent advances in 5G mmWave hardware with deep neural networks to deliver a new wireless imaging primitive for self-driving cars. In this respect, we built and evaluated two systems: (1) HawkEye (Through Fog High-Resolution Imaging Using Millimeter Wave Radar): a radar imaging system that leverages a generative adversarial network (GAN) model to reconstruct high-resolution 3D images with high-frequency shapes from low-resolution radar heatmaps. (2) Radatron (Accurate Detection Using Multi-Resolution Cascaded MIMO Radar): a mmWave radar-based object detection system that can predict precise bounding boxes of vehicles in real-world autonomous driving scenarios using a novel hybrid radar processing and deep learning approach.
Projects
Radical – Bootstrapping Autonomous Radars with Self-Supervised Learning Accepted to CVPR’24
Congratulations, Yiduo, Sohrab, and the team!
Radatron: Accurate Detection Using Multi-Resolution Cascaded MIMO Radar [ECCV’22]
Millimeter wave (mmWave) radars are becoming a more popular sensing modality in self-driving cars due to their favorable characteristics in adverse weather. Yet, they currently lack sufficient spatial resolution for semantic scene understanding. In this paper, we present Radatron, a system capable of accurate object detection using mmWave radar as a stand-alone sensor. To enable (…)
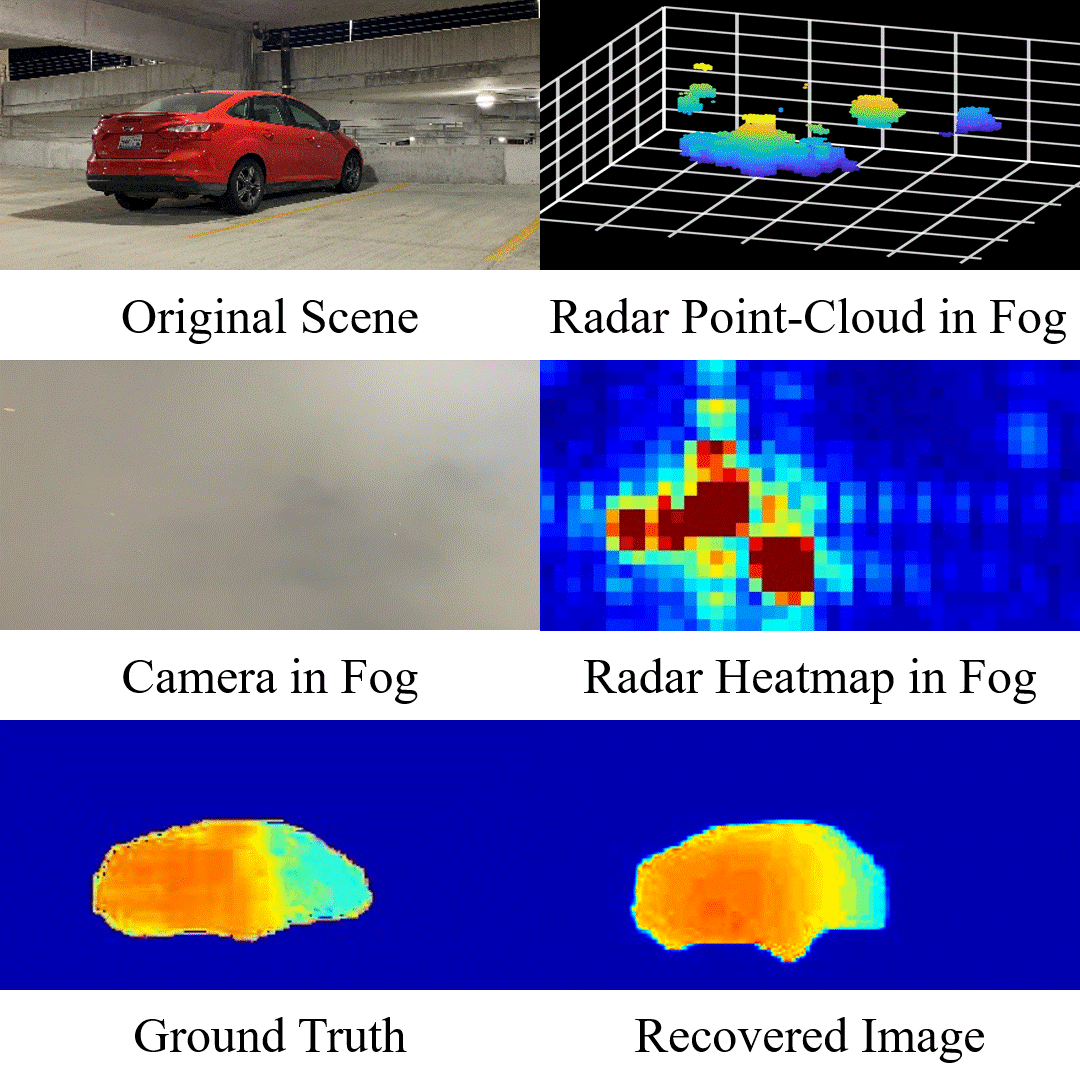
Through Fog High Resolution Imaging Using Millimeter Wave Radar [CVPR’20]
Abstract:This paper demonstrates high-resolution imaging using millimeter wave (mmWave) radars that can function even in dense fog. We leverage the fact that mmWave signals have favorable propagation characteristics in low visibility conditions, unlike optical sensors like cameras and LiDARs which cannot penetrate through dense fog. Millimeter wave radars, however, suffer from very low resolution, specularity, (…)