Student projects
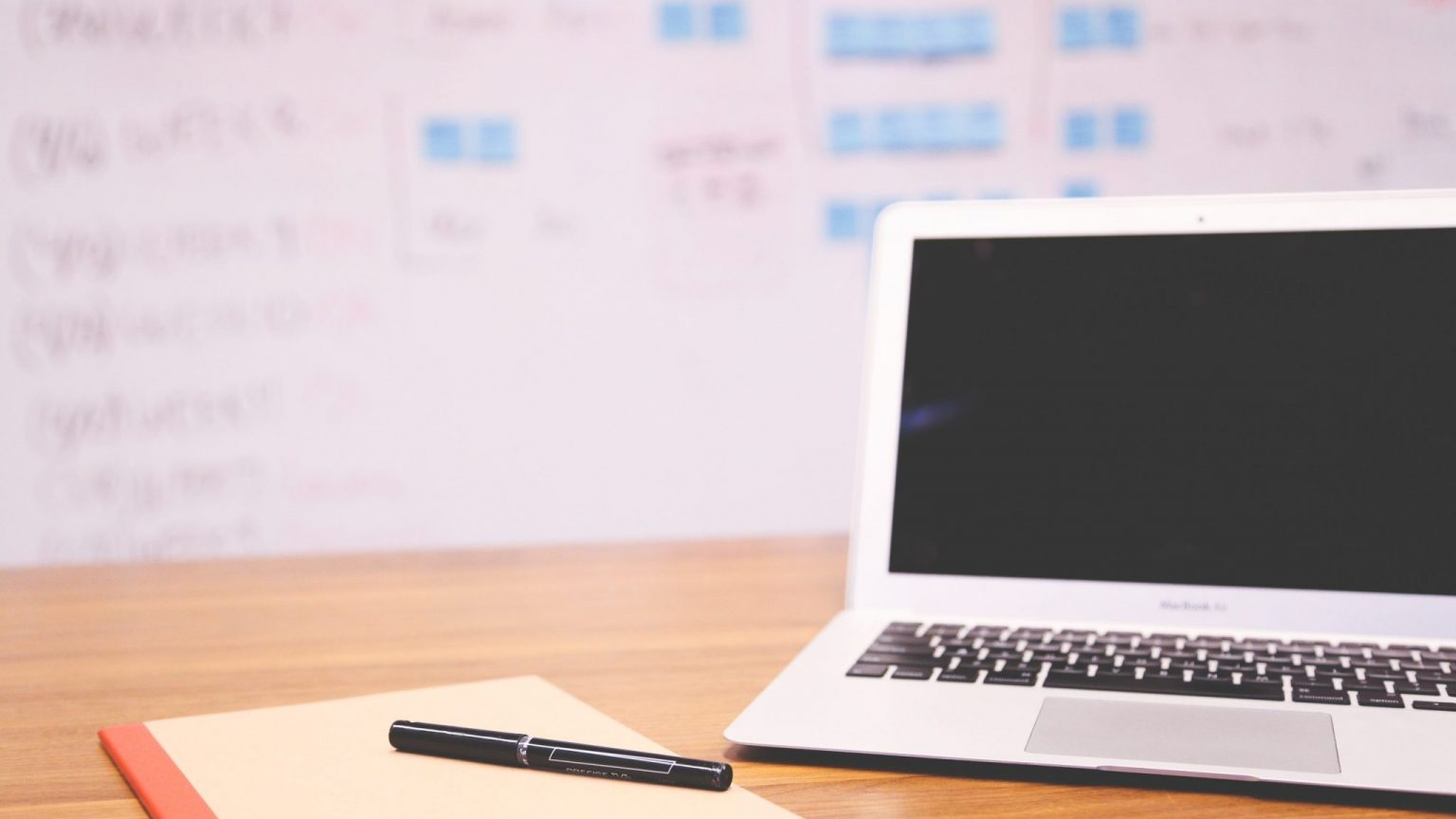
We divide our research into two synergistic theory thrusts: information scalable optimization and data acquisition, and learning theory and methods for low-dimensional signal models.
Our team of Postdocs and Phd students work on different aspects of these interesting and challenging problems, from theoretical analysis of methods and algorithms to efficient implementations to solve real-world problems.
Choosing our laboratory for your project will enable you to work in an exciting and stimulating environment, allow you to quickly learn state-of-the-art mathemathical and algorithmic tools and deliver an outstanding project.
Below, you can find some project that our team is currently working on and would appreciate your collaboration. You are also welcome to come with your own project topic that matches with our expertise and interests.
Please contact Prof. Volkan Cevher for further information on the projects.
Below projects are mainly reserved for Master students. In some cases they could also apply to Bachelor students. Please contact directly each of the projects’ supervisor for details. PhD students should consult LIONS open position page.
Open Projects
Quantum computers have a great potential to realize computationally efficient signal processing compared to traditional digital computers by exploiting quantum mechanism, e.g., superposition and entanglement. In the past few years, several vendors have successfully manufactured commercial quantum processing units (QPUs), including IBM’s 1121-qubit processors released in 2023. It is no longer far future when quantum computers will be widely used for various real applications. Recently, a number of modern machine learning frameworks have been introduced to quantum domain, e.g., quantum auto-encoder [1], graph neural network [2], generative adversarial network [3], and diffusion models [4].
This project is aimed at exploring the emerging quantum machine learning (QML) technologies for real-world applications. Specifically, we focus on integration of state-of-the-art deep learning framework with QML to realize novel computationally-efficient/memory-efficient architectures. This project is available for master students. It is encouraged to contact Toshiaki Koike-Akino and Francesco Tonin for details.
[1] J. Romero, J. P. Olson, and A. Aspuru-Guzik, “Quantum autoencoders for efficient compression of quantum data,” Quantum Science and Technology, vol. 2, no. 4, p. 045001, 2017.
[2] G. Verdon, T. McCourt, E. Luzhnica, V. Singh, S. Leichenauer, and J. Hidary, “Quantum graph neural networks,” arXiv preprint arXiv:1909.12264, 2019.
[3] S. Lloyd and C. Weedbrook, “Quantum generative adversarial learning,” Physical review letters, vol. 121, no. 4, p. 040502, 2018
[4] Zhang B, Xu P, Chen X, Zhuang Q. Generative quantum machine learning via denoising diffusion probabilistic models. Physical Review Letters. 2024 Mar 8;132(10):100602.
Can training samples be accurately recovered using only the weight matrices of a trained neural network (NN)? Recent works [1,2] have shown that accurate reconstruction can be achieved on image classification datasets by solving a relatively simple inverse problem defined by the model’s so-called Karush-Kuhn-Tucker (KKT) convergence conditions. This project aims to extend that technique to reconstruct training corpora from Large Language Models.
Such model inversion attacks pose serious privacy risks if sensitive data is contained within the training dataset. This work would analyze the ability to reconstruct personally identifying information or other private details memorized by Large Language Models (LLMs). Analyzing the limits of reconstruction will also provide insights into the degree to which language models memorize their training data versus truly learning generalizable representations. This has implications for understanding the capabilities and limitations of these powerful models.
As a starting point, a controlled setting would be devised where such reconstruction is theoretically predicted to be possible, and experiments will be conducted to understand how successful the reconstruction scheme might be on LLMs.
Beyond the initial reconstruction experiments, there are opportunities to develop defense mechanisms against such attacks. Future work could explore techniques for masking or obfuscating the model gradients to prevent reconstruction of private training inputs.This novel application at the intersection of NLP, privacy and adversarial machine learning would be well-suited for an interested master’s student. Please contact Dr. Noam Levi for more details on taking this research direction.
[1] Reconstructing Training Data from Trained Neural Networks
Niv Haim, Gal Vardi, Gilad Yehudai, Ohad Shamir, Michal Irani
[2] Deconstructing Data Reconstruction: Multiclass, Weight Decay and General Losses
Gon Buzaglo, Niv Haim, Gilad Yehudai, Gal Vardi, Yakir Oz, Yaniv Nikankin, Michal Irani
The projects listed below are in collaboration with Planny (https://planny.ch/), a young Geneva startup doing scheduling for nurses and doctors’ teams in the Swiss healthcare system.
Planny uses advanced improvisation algorithms to balance personnel’s vacation preferences, the needs of employers and patients and the constraints imposed by Swiss laws, for example making sure that enough rest is placed between night shift. The scheduling problem is modeled as a Mixed-Integer Programming problem (MIP). Since the general problem is NP complete, Planny has collected proprietary data and developed heuristics and algorithms specialized to its specific instantiation in the healthcare domain.
Synthetic Data for Scheduling Problems in the Healthcare Sector
This project focuses on developing a model and software framework for generating synthetic scheduling problems. The project has potentially high practical value, so internship/extra paid hours opportunities may be available as the project advances.
Project problem to target: The model created should be able to capture behaviours observable in the real data, but also be configurable enough to generate specialized scenarios and completely fabricated settings, in order to benchmark algorithms and provide environments which can be shared with students and other collaborators.It is especially suited for students interested in applied Data Science as would be done as part of an industrial product. Students will be able to “get their hands dirty”, possibly needing to dive deeper into open-source datasets, MIP solvers, general data augmentation techniques and other techniques that might be required for the final product.
The project can be tailored to either a Bachelor student project or a Bachelor thesis project and requires basic familiarity with python, numpy and pytorch.
References:
Sample deep learning algorithm that would leverage the generated data https://www.deepmind.com/publications/solving-mixed-integer-programs-using-neural-networks
Open-source datasets and frameworks the student will need to work with:
- https://www.ecole.ai/
- http://www.cs.nott.ac.uk/~pszrq/benchmarks.htm
- http://elib.zib.de/pub/mp-testdata/tsp/tsplib/tsp/
Modification Game: Navigate the Solutions of a medical MIPs problem
This project focuses on the creation of learning-based algorithms which can efficiently modify the solution of Mixed Integer Problems when constraints and preferences change.
The project has potentially high practical value to Planny, so internship/extra paid hours opportunities may be available as the project advances.
Project problem to target: Given the schedule of a team (think: solution of a MIP problem), constraints and preferences might change: someone gets sick and must be replaced. Ideally, Planny would like to supply the manager of the team with a great recommendation about whom to replace the sick person with; ideally, in real-time (at the click of a button in the app).
One way to model this problem is to think that the schedule is a board game (with states) and small changes in the schedule are moves in the game. The optimisation objectives (or other relevant metrics) are then rewards the players can collect. The players would have to learn to come up with a great strategy/policy, i.e. to replace the last minute absent people without breaking the rules of the game (too much).
The project can be tailored to Bachelor, Master semester or Master projects in terms of workload and requires good knowledge of python, numpy and pytorch. An interested student would also have to be familiar with or be able to quickly learn concepts in Combinatorial Optimization and Reinforcement Learning.
References:
- https://openreview.net/pdf?id=xEQhKANoVW
- https://arxiv.org/pdf/1811.06128.pdf
- https://www.ecole.ai/
- http://www.cs.nott.ac.uk/~pszrq/benchmarks.htm
- http://elib.zib.de/pub/mp-testdata/tsp/tsplib/tsp/
- https://www.deepmind.com/publications/solving-mixed-integer-programs-using-neural-networks
Infeasability Recourse: Almost Constraint Satisfaction for medical MIPs
This project focuses on the development of efficient algorithms which find the minimal constraint relaxation required to turn an infeasible Mixed Integer Problem into a feasible one, as measured by a domain specific metric.
The project has potentially high practical value, so internship/extra paid hours opportunities may be available as the project advances.
Project problem to target:: Planny often faces the situation that the various constraints cannot be fulfilled at the same time, yielding an infeasible scheduling problem. In such a situation, the users have to work with Planny to identify constraints which can be modified or dropped completely in an attempt to make the problem feasible. This is similar to the problem of “recourse” faced in the credit scoring industry. Here, a firm using automated decision making to deny a load must be able to offer the denied applicants an explanation and a feasible action with which to remedy the application so it might succeed, instead of a “black-box” refusal (see reference). This project investigates automated ways of doing the same, but instead of a single action offering a list of possible modifications of the combinatorial problem.
It can be tailored to a Bachelors thesis, Master semester project or Masters thesis in terms of workload and requires good knowledge of python, numpy and pytorch. An interested student would also have to be familiar with or be able to quickly learn concepts in Combinatorial Optimization, Graph Neural Networks and Counterfactual Causality in the Pearlian sense.
References:
- https://openreview.net/pdf?id=xEQhKANoVW
- https://arxiv.org/pdf/1811.06128.pdf
- https://www.ecole.ai/
- http://www.cs.nott.ac.uk/~pszrq/benchmarks.htm
- http://elib.zib.de/pub/mp-testdata/tsp/tsplib/tsp/
- https://www.deepmind.com/publications/solving-mixed-integer-programs-using-neural-networks
Robust Solutions to MIP: Scheduling against last minute changes!
This project is concerned with finding robust solutions to Mixed Integer Programming problems in the sense that a solution remains feasible under the constraints under the adversarial assignment of a subset of variables (e.g., a person becoming sick and being forced to recover)
The project has potentially high practical value, so internship/extra paid hours opportunities may be available as the project advances.
Project problem to target: This project is related to the recourse game but attempts to make the modifications easier by creating slack in the solutions found by the MIPs solver by finding the solution not of the original problem but one which has been perturbed in the worst possible way while remaining feasible.
The project can be tailored to Master semester project and Master theses in terms of workload and requires good knowledge of python, numpy and pytorch.
An interested student would also have to be familiar with or be able to quickly learn concepts in Combinatorial Optimization, Graph Neural Networks and Min-Max game theory.
References:
- https://openreview.net/pdf?id=xEQhKANoVW
- https://arxiv.org/pdf/1811.06128.pdf
- https://www.ecole.ai/
- http://www.cs.nott.ac.uk/~pszrq/benchmarks.htm
- http://elib.zib.de/pub/mp-testdata/tsp/tsplib/tsp/
- https://www.deepmind.com/publications/solving-mixed-integer-programs-using-neural-networks
If interested, please contact Igor Krawczuk
Many combinatorial optimization problems arising in real-world applications are known to be NP-hard and thus highly unlikely to admit efficient polynomial-time algorithms in the worst-case sense.
A fundamental heuristic used over the years to tackle such intractable combinatorial optimization problems is the Branch-and-Bound method (BB). This approach reduces the problem of interest to an instance of Integer Linear Programming (MIP) and then the derived (MIP) instance is solved through the (BB) method. The (BB) method keeps a set of partially-integral solutions that iteratively expands via setting a non-integral variable to an integral one. Despite the fact that (BB) method can take exponential-time to converge it is known that several variable expansion policies lead to competitive running time.
When solving combinatorial optimization problems, unsupervised learning-based methods can improve generalization by exploiting large-scale and unlabeled datasets [1]. However, there are significant challenges to apply unsupervised learning. In particular, continuous relaxations of objective functions in combinatorial problems typically lead to degenerate solutions and suffer from additional challenges to optimize. This makes choosing an appropriate loss a key factor to solve combinatorial problems using unsupervised learning. Even after solving a suitable relaxed problem, ensuring that a recovered discrete solution obtained by the soft assignments of a neural network satisfies the integer constraints is a challenging task [3].
Recently, Erdos’ probabilistic method has been used to solve combinatorial optimization on graphs which provides integer solutions with certified quality [2]. Karalias and Loukas have trained a GNN to produce a distribution over subsets of nodes of an input graph by minimizing a probabilistic penalty loss function. After training the GNN, they have used a well-known technique from randomized algorithms to sequentially and deterministically decode a valid solution from the learned distribution inspired by the method of conditional expectation. However, since this method is autoregressive, decoding suffers from high latency.
To extract the feasible solution efficiently, in this project, we plan to build on our preliminary work in Graph Generation, GG-GAN [4]. While [2], like the current State of the art Graph generation method rely on sequential decoding of an autoregressive model, GG-GAN allows efficient generation of graphs with a single forward pass.
[1] Saeed Amizadeh, Sergiy Matusevych, and Markus Weimer. Learning to solve circuit-sat: An unsupervised differentiable approach. In Proc. ICLR, 2019.
[2] Nikolaos Karalias and Andreas Loukas. Erdos goes neural: an unsupervised learning framework for combinatorial optimization on graphs. In Proc. NeurIPS, 2020.
[3] Daniel Selsam, Matthew Lamm, Benedikt Bünz, Percy Liang, David L. Dill, and Leonardo de Moura. Learning a SAT solver from single-bit supervision. In Proc. ICLR, 2019.
[4] Igor Krawczuk, Pedro Abranches, Andrej Janchevski, Andreas Loukas, and Volkan Cevher. GG-GAN: A geometric graph generative adversarial network. under review.
This project is available for a semester project or an MSc Thesis. If interested, please contact Igor Krawczuk , Stratis Skoulakis, for details. Students should be familiar with PyTorch/JAX. Familiarity with combinatorial optimization and graph neural networks is a plus.
It is widely known that training deep neural networks on huge datasets improves learning. However, huge datasets and deep neural networks can no longer be trained on a single machine. One common solution is to train using distributed systems. In addition to traditional data-centers, in federated learning (FL), multiple clients, e.g., a few hospitals and thousands of cellphones learn a model without sharing local data to prevent the potential privacy issues.
Several methods have been proposed to accelerate training for classical empirical risk minimization (ERM) in supervised learning such as gradient (or model update) compression, gradient sparsification, weight quantization/sparsification, and reducing the frequency of communication though multiple local updates. Unbiased vector quantization is in particular an interesting compression method due to both enjoying strong theoretical guarantees along with providing communication efficiency on the fly, i.e., it converges under the same hyperparameteres tuned for vanilla uncompressed SGD while providing substantial savings in terms of communication costs [1-3].
In this project, we investigate how to accelerate training deep neural networks in distributed reinforcement learning (DRL) [4-8]. In particular, our goal is to show: 1) How can we modify adaptive variants of unbiased quantization schemes tailored to general DRL problems; 2) Can we achieve optimal rate of convergence while establishing strong guarantees on the number of communication bits? 3) Do our new methods show strong empirical performance on deep neural networks and huge datasets, both in terms of performance measures and scalability?
[1] Dan Alistarh, Demjan Grubic, Jerry Z. Li, Ryota Tomioka, and Milan Vojnovic. QSGD: Communication-efficient SGD via gradient quantization and encoding. In Proc. NeurIPS, 2017.
[2] Fartash Faghri, Iman Tabrizian, Ilia Markov, Dan Alistarh, Daniel M. Roy, and Ali Ramezani-Kebrya. Adaptive gradient quantization for data-parallel SGD. In Proc. NeurIPS, 2020.
[3] Ali Ramezani-Kebrya, Fartash Faghri, Ilya Markov, Vitalii Aksenov, Dan Alistarh, and Daniel M. Roy. NUQSGD: Provably communication-efficient data-parallel SGD via nonuniform quantization. JMLR, 22(114):1–43, 2021.
[4] Drew Bagnell and Andrew Ng. On local rewards and scaling distributed reinforcement learning. In Proc. NeurIPS, 2005.
[5] Eric Liang, Richard Liaw, Robert Nishihara, Philipp Moritz, Roy Fox, Ken Goldberg, Joseph Gonzalez, Michael Jordan, Ion Stoica. RLlib: Abstractions for distributed reinforcement learning. In Proc. ICML, 2018.
[6] Xiaofeng Fan, Yining Ma, Zhongxiang Dai, Wei Jing, Cheston Tan, and Bryan Kian Hsiang Low. Fault-Tolerant Federated Reinforcement Learning with Theoretical Guarantee. In Proc. NeurIPS, 2021.
[7] Srivatsan Krishnan, Maximilian Lam, Sharad Chitlangia, Zishen Wan, Gabriel Barth-Maron, Aleksandra Faust, and Vijay Janapa Reddi. QuaRL: Quantization for sustainable reinforcement learning. arXiv:1910.01055, 2021.
[8] Srivatsan Krishnan, Maximilian Lam, Sharad Chitlangia, Zishen Wan, Gabriel Barth-Maron, Aleksandra Faust, and Vijay Janapa Reddi. Settling the communication complexity for distributed offline reinforcement learning. arXiv:1910.01055, 2022.
This project is available for a semester project or an MSc Thesis. If interested, please contact Igor Krawczuk for details. Students should be familiar with reinforcement learning, PyTorch/JAX, MPI, CUDA. Familiarity with distributed optimization is a plus.
Self-supervised learning (SSL) has attracted significant attention, which lets us learn a model without requiring labelled data. SOTA SSL methods for computer vision tasks include pretratining by Image Rotation [1], Bootstrap Your Own Latent (BYOL) [2], Barlow Twins [3]. In this project, we plan to find vulnerabilities and failure modes of SOTA SSL methods.
[1] Gidaris, Spyros, Praveer Singh, and Nikos Komodakis. “Unsupervised representation learning by predicting image rotations.” ICLR 2018.
[2] Grill, Jean-Bastien, et al. “Bootstrap your own latent-a new approach to self-supervised learning.” NeurIPS 2020.
[3] Zbontar, Jure, et al. “Barlow twins: Self-supervised learning via redundancy reduction.” ICML 2021.
The project can be for both a master thesis and a semester project students. Computer science, math, and data science students will be a good fit for the project. Max 1-2 students.
If interested please contact Thomas Pethick for details.
Even though significant progress has been made in the understanding of the generalization guarantees in the case of balanced data, the real-world is rarely so nicely formatted [1]. In fact, in our daily lives we do not encounter each object with uniform probability. The goal of this project is to focus on such examples closer to the real-world applications and study the generalization and the inductive bias of networks [2] trained on imbalanced data.
[1] Li, Bolian, et al. “Trustworthy Long-Tailed Classification.” Proceedings of the IEEE/CVF Conference on Computer Vision and Pattern Recognition. 2022.
[2] Zhu, Z., Liu, F., Chrysos, GG, Cevher, V. “Robustness in deep learning: The good (width), the bad (depth), and the ugly (initialization)”. NeurIPS, 2022.
Keywords: deep neural networks, long-tailed distribution, imbalance data.
If interested please contact Dr Grigorios Chrysos for details.
Neural networks have demonstrated they are prone to malicious attacks. Indeed, targeted attacks (e.g. by modifying the input with adversarial noise [1]) can be detrimental for the performance of networks. A number of works have recently focused on verifying the performance of neural networks [2-3], transformers [4] and polynomial nets [5] against such attacks. However, every one of the methods above works for a specific class of functions, while the network design is getting more diverse. The goal of this project is to certify the performance of a general class of functions. The proposed certification method is expected to be useful across popular network structures and pave the way for more generic verification methods.
[1] Szegedy, C., Zaremba, W., Sutskever, I., Bruna, J., Erhan, D., Goodfellow, I., & Fergus, R. (2014). “Intriguing properties of neural networks”. ICLR.
[2] Bastani, Osbert, et al. “Measuring neural net robustness with constraints.”, NeurIPS’16.
[3] Wang, Shiqi, et al. “Beta-crown: Efficient bound propagation with per-neuron split constraints for complete and incomplete neural network verification.”, NeurIPS’21.
[4] Bonaert, Gregory, et al. “Fast and precise certification of transformers.” Proceedings of the 42nd ACM SIGPLAN International Conference on Programming Language Design and Implementation. 2021.
[5] Rocamora, Elias Abad, Mehmet Fatih Sahin, Fanghui Liu, Grigorios G. Chrysos, and Volkan Cevher. “Sound and Complete Verification of Polynomial Networks.”, NeurIPS’22.
Prerequisites and goal: The student should be willing to learn the required tools rapidly. The goal is to lead this to a publication into a top ML conference.
This project is intended for a single student. It is available for computer science, communication systems and data science students. If interested please contact Dr Grigorios Chrysos for details.
Project goal: A model design that (i) learns high-order interactions between the elements of the input signal and (ii) captures minor discrepancies from the distribution of real images that indicate manipulated or synthetic content.
If interested please contact Dr Grigorios Chrysos for details.
[2] Choraria, Dadi, Chrysos, Mairal, Cevher. The Spectral Bias of Polynomial Neural Networks, ICLR’22.
[3] Sohn, Li, Yoon, Jin, Pfister. Learning and Evaluating Representations for Deep One-class Classification, ICLR’21.
[4] Rombach, et al. High-resolution image synthesis with latent diffusion models, CVPR’22.
High-degree polynomial expansions have been recently used for approximating functions with high-dimensional signals and have obtained state-of-the-art performance in challenging tasks, such as image generation and image classification [1, 2]. However, little is known about the robustness of polynomial networks. Targeted attacks (e.g. by modifying the input with adversarial noise [3]) can be detrimental for the performance of polynomial networks. In this project we are interested in providing guarantees against such attacks [4]. Specifically, the following components will be considered during the project:
- Constraint-based techniques: a system of constraints can be used to prove that polynomial networks satisfy some properties of interest with respect to its robustness.
- Approximate techniques: instead of relying on a single given input, we can use a large (or infinite) set of inputs to study the desirable robustness properties.
[1] “Deep Polynomial Neural Networks”, Grigorios Chrysos, et al., IEEE Transactions PAMI 2021.
[2] “Conditional Generation Using Polynomial Expansions”, Grigorios Chrysos, Markos Georgopoulos, Yannis Panagakis, NeurIPS 2021.
[3] “Intriguing properties of neural networks”, Christian Szegedy, et al., ICLR 2014.
[4] “Measuring Neural Net Robustness with Constraints”, Osbert Bastani, et al., NeurIPS 2016.
Prerequisites and goal: The student should have a theoretical background to participate in this project, and/or be willing to learn the required theoretical tools rapidly. The goal is to lead this to a publication into a top ML conference.
If interested please contact Dr Grigorios Chrysos for details.
In a distributed setting, gradients are compressed to reduce communication costs. Examples of compression methods are signSGD, QSGD, and NUQSGD. Over the network, transmitted bits (e.g., sign of each gradient coordinate sent by a compute node) can be flipped either due to network unreliability or due to manipulation by an adversary. The most basic scenario is a parameter-server setting where the uplink is noisy (the adversary changes some bits randomly). A fundamental question is how many extra bits (redundancy as a price for robustness) we should add to have a bounded variance in terms of aggregated updates considering two sources of randomness (mini-batch and uncertainty over networks).
This semester project is available for master students only. It is an individual project. A student should mainly specialise in Cyber security and Communication systems.
This semester project is available for Bachelor and/or Master student, Computer science, Cyber security, Communication systems and/or Data Science. It is intended for one student only.
Minimax settings have attracted significant attention due to the success of generative adversarial networks (GANs) and adversarial training. While the underlying optimization algorithms have been studied extensively in terms of their convergence (optimization error), their generalization to an unseen example, remained an open problem. Generalization error depends on the design of the learning algorithm and the characteristics of the loss function. Unlike generalization analyses which are based on the complexity of the hypothesis class, the stability analysis focuses on how the learning algorithm explores the hypothesis class. Two main stability notions in the literature are uniform and on-average stability. Developing stability bounds for minimax settings is an important problem in machine learning.
This semester project is available for master students only. It is an individual project. A student should mainly specialise in Computer Science but it could also be avaialble for other fields (Cyber security, Communication systems and/or Data Science).
Understanding over-parameterized models (e.g., deep neural networks) has recently attracted great attention in deep learning theory. Neural tangent kernel (NTK) [1] and mean field analysis [2] are two representative approaches to analyze over-parameterized models under different settings.
In this project, we start from the two-layer neural network, a good starting point, to understand its optimization and generalization properties. Previous work [3,4] incorporate NTK and mean field analysis under the unified framework. Albeit simple, there are several unanswered questions of the two-layer neural network in terms of its normalization, initialization, optimization dynamics (early stage vs. stable stage), and generalization.
Besides, there are several more fundamental issues behind this, including the relationship between the integral operator and the kernel matrix in high dimensions, the relationship between RKHS and its norm (e.g., NTK, Laplace kernels have the same RKHS. This does not mean their RKHS norm are the same), and how to precisely characterize the RKHS beyond eigenvalue decay.
[1] Jacot A, Hongler C, Gabriel F. Neural Tangent Kernel: Convergence and Generalization in Neural Networks. NeurIPS 2018.
[2] Mei S, Montanari A, Nguyen PM. A mean field view of the landscape of two-layer neural networks. PNAS 2018.
[3] Chen Z, Cao Y, Gu Q, Zhang T. A generalized neural tangent kernel analysis for two-layer neural networks. NeurIPS 2020.
[4] Luo T, Xu ZQ, Ma Z, Zhang Y. Phase diagram for two-layer ReLU neural networks at infinite-width limit. JMLR2021.
Prerequisites: There is no formal prerequisite. This is a relatively theoretical project and thus students should have a background in analysis, linear algebra, statistics and machine learning algorithms. Prior exposure to deep learning experience and optimization is helpful, but not required. If interested please contact Dr. Fanghui Liu or Zhenyu Zhu for details.
At LIONS, we have developed provably efficient Reinforcement Learning algorithms to solve zero-sum Markov Game. Many popular challenges like the game of Go fall in this category. Thus, we are interested in an empirical study of this algorithm.
The first part of the project will be devoted to the development of a function approximation extension of our algorithms ( previously studied in the tabular case) while the second one will address its efficiency in self-play training.
Prerequisites: you should ideally be at ease with Python and PyTorch and have some knowledge of actor critic algorithms.
In case of interest please contact Luca Viano for details.
Recent theoretical works of the lab have shown provable benefits of incorporate optimistic exploration ideas, originally developed in the context of adversarial MDP, into imitation learning algorithms. For the class of linear MDP, this idea lead to the best known sample complexity and to remarkable empirical performance in the case of finite states and actions or linear function approximation.
The goal of this project is to extend these ideas and study heuristics to apply approximately the optimistic exploration ideas in the case of neural network function approximation.
We will most likely test the algorithm in commonly used simulated robotic problems.
In case of interest please contact Luca Viano for details.
The project can be for both a bachelor or master thesis or for a semester project. A student should specialise in Computer science, Cyber security, Communication systems and/or Data Science.
This is part of a large scale project concerned with studying way to fully automate the design and layouting of ASICS. Possible projects touch on reinforcement learning, generative models, representation learning and theoretical work on how to represent and decompose action and state spaces.
If interested please contact Igor Krawczuk for details
Neuromorphic machine learning hardware uses device physics like the switching dynamics of memristive devices or the charge dynamics of a capacitor to perform computation. To estimate the efficacy of neuromorphic machine learning hardware, one needs to marry together two computationally intensive tasks: we need to run machine learning algorithms on problems representative of those faced in reality, while at the same time modeling the device physics used to perform the computations. This is somewhat unique workload since the total training time will be orders of magnitude longer than what is easily feasible in circuit simulations, while possibly depending on the interactions of varying subsets of individual devices. This means that we will need to mix both abstract and detailed models, since global abstraction into behavioral or probabilistic models is risky. To accommodate this special need, we are developing a multi-model simulation framework.
This framework is is intended to minimize the computational load for the physical simulations by properly adapting the model to the desired accuracy and scale well onto GPU and cluster environments. In this project you will contribute to this framework by collecting existing device models for memristive devices from the literature and implement them as compute kernels. The project complexity can be scaled to both Master and Semester projects. Estimated workload breakdown:
30 % literature survey
50 % model selection and implementation in Julia
20 % Integration into framework and verification
If interested please contact Igor Krawczuk for details
Closed projects
Transformers achieve powerful performance in various tasks but do not scale efficiently on long sequences due to the quadratic time and space complexity in (self)-attention structure. Many works have been proposed to linearize attention by approximating the soft-max function, including random features based algorithms [1,2], Nystrom approximation [3], see a survey for details [4].
In this project, we are interested in building on some recent lines of works aimed at attention linearization, such as developing quadrature based rules for linearization, integrating the relative positional encoding, or directly design a linear attention module.
[1] Choromanski, K. M., Likhosherstov, V., Dohan, D., Song, X., Gane, A., Sarlos, T., … & Weller, A. Rethinking Attention with Performers. ICLR2021.
[2] Peng, H., Pappas, N., Yogatama, D., Schwartz, R., Smith, N., & Kong, L. Random Feature Attention. ICLR 2021.
[3] Xiong, Y., Zeng, Z., Chakraborty, R., Tan, M., Fung, G., Li, Y., & Singh, V. Nyströmformer: A Nyström-based Algorithm for Approximating Self-Attention. AAAI 2021.
[4] Fournier, Q., Caron, G. M., & Aloise, D. A Practical Survey on Faster and Lighter Transformers. arXiv preprint arXiv:2103.14636, 2021.
Prerequisites: Students should have deep learning experience. If interested please contact Dr. Fanghui Liu for details.
Diffusion models like DALL-E 2 (https://arxiv.org/abs/2204.06125), Stable
Diffusion (https://github.com/CompVis/stable-diffusion), and many more
generative models, can create high-resolution images corresponding to natural language queries. As such, they have shown to be capable of replacing, in part, the job of digital artists. This poses a big ethical problem as such models are trained on images whose intelectual rights could be disputed at any time.Currently, there are no incentives for digital artists to provide their work for big companies that have the budget to train large generative models. On the other hand, there are risks for companies offering such services, as they are exposed to intellectual property lawsuits. Hence, it is urgent that we develop a fair method of compensating the training data owners. Otherwise this threatens both the availability of human-created training sets and services based on generative models.This problem is really difficult as a single generated image could have been
produced by the model, by drawing inspiration from thousands, if not all, the elements of the training dataset. In this work we will create a dataset and define a fair attribution challenge, that aims to provide a benchmark for the described problem. Alongside this foundation, we will develop scalable baseline solutions using image data as well as variables extracted from open-source diffusion models like Stable Difussion (https://arxiv.org/abs/2112.10752).
The project can be for both a master thesis and a semester project students. Computer science, mathematics, and data science students will be a good fit for the project. Max 1-2 students. Students should be familiar with Deep Learning tools like PyTorch or JAX. If interested please contact Fabian Latorre for details
Attention Mechanisms [1] obtain state-of-the-art performance across a range of tasks in Natural Language Processing, as well as in Computer Vision tasks [2]. However, their design principles are still poorly understood. Moreover, one major drawback of the vanilla Attention module is the quadratic complexity as a function of the sequence length, which hinders its applicability to long sequences.
The goals of this project are two-fold: first, to perform an ablation study of Attention, assessing how important is the presence of activation functions for their success in Language Models. For this a benchmark suite is available in Jax and Pytorch [3]. Second, to experimentally validate in the same context the feasibility of replacing the Attention mechanisms, with a generic third-degree Polynomial Network with similar or lower computational complexity. A preliminary work in [4] illustrates that this is possible in image-based tasks, however what we intend to do in this project should be applicable in both NLP and Vision.
[1] Attention is all you need. Ashish Vaswani, Noam Shazeer et al. [https://arxiv.org/abs/1706.03762]
[2] An Image is Worth 16×16 Words: Transformers for Image Recognition at Scale Alexey Dosovitskiy, Lucas Beyer et al. [https://arxiv.org/abs/2010.11929]
[3] Long Range Arena: A Benchmark for Efficient Transformers. Yi Tay, Mostafa Dehghani et al. [https://openreview.net/forum?id=qVyeW-grC2k]
Generative Adversarial Networks (GANs) [1] have been at the forefront of Deep Learning Research in recent years. This topic of study has found many impactful applications, based on the generation of synthetic but realistic visual data [2]. The methodology consists of finding an equilibrium of a zero-sum game between two players, represented by two neural networks referred to as the generator and the discriminator.
Nevertheless, their underlying workings are still obscure in the sense that it is not clear which variables allow their succesful training or improve the quality of their results [3]. In particular, Wasserstein GANs (WGANs) [4] appear to depend crucially on the regularization of the discriminator network.
In this work, we plan to study the feasibility of regularizing WGANs through a penalty on the Path-Norm [5] of the Discriminator Network. This is a principled choice as this measure upper bounds the Lipschitz constant of the Discriminator [6], which is a constraint in the WGANs formulation.
Path-Norm regularization through proximal methods is a highly difficult problem, but we have recently solved it for simple cases like shallow networks [6] and deep unit-width networks. However, our analysis does not extend to complex multilayer architectures with skip-connections or convolutional layers which are commonly used in practical applications [2]. For this reason we will focus on identifying strengths and weaknesses of Automatic Differentiation methods for Path-Norm regularization and incorporate it in a WGAN training framework that is applicable to common real-world settings, such as image generation with CIFAR10/ImageNet datasets.
[1] Generative Adversarial Networks. Ian J. Goodfellow, Jean Pouget-Abadie, Mehdi Mirza, Bing Xu, David Warde-Farley, Sherjil Ozair, Aaron Courville, Yoshua Bengio.
[2] Analyzing and Improving the Image Quality of StyleGAN. Tero Karras, Samuli Laine, Miika Aittala, Janne Hellsten, Jaakko Lehtinen, Timo Aila.
[3] Are GANs Created Equal? A Large-Scale Study Mario Lucic, Karol Kurach, Marcin Michalski, Sylvain Gelly, Olivier Bousquet.
[4] Wasserstein GAN Martin Arjovsky, Soumith Chintala, Léon Bottou.
[5] Norm-Based Capacity Control in Neural Networks Behnam Neyshabur, Ryota Tomioka, Nathan Srebro.
New optimization algorithms are being developed for Supervised Learning of Deep Neural Networks, that obtain better generalization errors using contemporary architectures like Residual Networks. One such recent example is Sharpness Aware Minimization (SAM) (https://arxiv.org/pdf/2010.01412.pdf) which tries to find parameters such that the training loss is robust to a bounded perturbation of the parameters.In this project we will work towards two related objectives: first, we will look to characterize mathematical properties of Networks trained with SAM, in order to better explain its success, that is, we want to improve the
interpretability of SAM. For example, we will evaluate how smooth are
SAM-trained networks compared to their vanilla SGD-trained counterparts. We will also explore the feasibility of upper bounds on the SAM loss, that avoid the saddle-point formulation, and instead solve a regularized minimization problem which might be easier to solve from an optimization perspective.As a secondary goal, we will develop alternative optimization algorithms for Sharpness Aware Minimization, that take into account its nonconvex-nonconcave saddle-point formulation.
The project can be for both a master thesis and a semester project students. Computer science, mathematics, and data science students will be a good fit for the project. Max 1-2 students. Students should be familiar with Deep Learning tools like PyTorch or JAX. If interested please contact Fabian Latorre for details.
Self-supervised learning (SSL) has forced a paradigm-shifting from supervised learning towards unsupervised learning. The principle is to use a number of pseudo-labels, e.g. colorization [1], contrastive learning [2-4], jigsaw puzzle reconstruction [5]. Despite the empirical success, we do not have a characterization over the impact of several parameters in SSL. In this project, we intend to study the impact of width, depth and initialization in the networks learned with SSL.
[1] Larsson, Gustav, Michael Maire, and Gregory Shakhnarovich. “Colorization as a proxy task for visual understanding.”, CVPR’17.
[2] Purushwalkam, Senthil, and Abhinav Gupta. “Demystifying contrastive self-supervised learning: Invariances, augmentations and dataset biases.”, NeurIPS’20.
[3] He, Kaiming, et al. “Momentum contrast for unsupervised visual representation learning.”, CVPR’ 20.
[4] Chen, Ting, et al. “A simple framework for contrastive learning of visual representations.”, ICML’20.
[5] Noroozi, Mehdi, and Paolo Favaro. “Unsupervised learning of visual representations by solving jigsaw puzzles.”, ECCV’16.
Prerequisites and goal: The student should be willing to learn the required tools rapidly. The goal is to lead this to a publication into a top ML conference.
This project is intended for a single student. It is available for computer science, communication systems and data science students. If interested please contact Dr Grigorios Chrysos for details.
Transformers [1] have demonstrated state-of-the-art behavior in image recognition [2] or image generation [3]. Simultaneously, a new paradigm of searching automatically for new architectures in neural networks has emerged. The idea in neural architecture search (NAS) [4] is to select a search space and then optimize not only over the weights, but also over candidate architectures from the search space. Despite the computational cost, NAS has demonstrated a state-of-the-art behavior. In this project we aim to extend NAS for transformers. Doing this naively can be a costly search, however we aim to extend efficient search for defining new transformer architectures.
[1] “Attention is all you need”, Ashish Vaswani, et al., NeurIPS 2017.
[2] “An Image is Worth 16×16 Words: Transformers for Image Recognition at Scale”, A. Dosovitskiy, et al., ICLR 2021.
[3] “STransGAN: An Empirical Study on Transformer in GANs”, R. Xu, et al.
[4] “Neural architecture search with reinforcement learning”, B. Zoph, Q. Le, ICLR 2017.
Prerequisites and goal: The project is available for a semester project or an MSc thesis. The student should be highly motivated, and have strong coding skills. The goal is to lead this to a publication into a top ML conference.
If interested please contact Dr Grigorios Chrysos for details.
Transformers and convolutional neural networks (CNNs) have been the two most important paradigms across a range of image-related tasks, such as image recognition [1, 2] or image generation [3]. Most of the progress so far has been focused on in-distribution performance. However, the performance of transformers and CNNs in unseen domains, i.e. out-of-distribution, is a critical advance required before deploying neural networks in real-world tasks. Building upon previous work comparing the performance of transformers and CNNs in out-of-distribution performance [4, 5], we will explore the relationship between in-distribution and out-of-distribution performance. Specifically, we are interested in exploring whether there is a consistent correlation between those two and how this can be predicted using domain adaptation theory [6].
[1] “Deep Residual Learning for Image Recognition”, Kaiming He, et al., CVPR 2016.
[2] “Attention is all you need”, Ashish Vaswani, et al., NeurIPS 2017.
[3] “Large scale GAN training for high fidelity natural image synthesis”, Andrew Brock, Jeff Donahue, and Karen Simonyan, ICLR 2019.
[4] “Oodformer: Out-of-distribution detection transformer”, Rajat Koner, et al., Arxiv 2021.
[5] “Exploring the limits of out-of-distribution detection”, Stanislav Fort, Jie Ren, and Balaji Lakshminarayanan, NeurIPS 2021.
[6] “A theory of learning from different domains”, Shai Ben-David,, et al. Machine learning 79.1 (2010): 151-175.
Prerequisites and goal: The project is available for an MSc thesis. The student should be highly motivated, and have either a theoretical background or strong coding skills. The goal is to lead this to a publication into a top ML conference.
If interested please contact Dr Grigorios Chrysos for details.
A wealth of datasets have been proposed for tackling challenging tasks, such as image recognition or image segmentation. However, real-world applications often suffer from domain shift, namely the testing data are sampled from a different distribution than the training data. Domain adaptation techniques [1] have been developed precisely for tackling the domain shift. In this project, we will use few-shot domain adaptation, in which we will assume that few samples from the target domain can be labelled. We intend to study it both in the standard classification setting [2, 3], as well as in the dense regression setting, where the output is a segmentation map or another dense task.
[1] “Semi-supervised domain adaptation via minimax entropy”, Kuniaki Saito, et al., ICCV 2019.
[2] “Contradictory Structure Learning for Semi-supervised Domain Adaptation”, Qin Can, et al., SIAM International Conference of data mining 2021.
[3] “Domain-Adaptive Few-Shot Learning”, An Zhao, WACV 2021.
This project is available for both a semester project and an MSc thesis. If interested please contact Dr Grigorios Chrysos for details.
The collection and annotation of massive datasets (e.g. Cityscapes, ImageNet) has enabled vast progress in the challenging tasks (e.g. semantic segmentation, classification). The collection of such datasets is a gargantuan task, which is even impossible in some cases. For instance, in autonomous driving it is impossible to collect a dataset with every scene that the underlying model will face. Instead, we can rely on (unsupervised) domain adaptation [1]. The idea is to find a `related’ dataset that can act as the source domain. Then, we can use the annotated source dataset to learn the labels for the target domain we are interested in. Then, we can use the labels provided by the source domain to label the data in the target domain and thus learn the downstream task (e.g. semantic segmentation). However, the downstream task is prone to noise [2] in both the source domain and more importantly the target domain. The goal of this project is to develop techniques for making the downstream task robust to different types of noise [3, 4].
[1] Wang, M., & Deng, W. (2018). “Deep visual domain adaptation”: A survey. Neurocomputing, 312, 135-153.
[2] Szegedy, C., Zaremba, W., Sutskever, I., Bruna, J., Erhan, D., Goodfellow, I., & Fergus, R. (2014). “Intriguing properties of neural networks”. ICLR.
[3] Chrysos, G. G., Kossaifi, J., & Zafeiriou, S. (2020). “RoCGAN: Robust Conditional GAN”. International Journal of Computer Vision, 128(10), 2665-2683.
[4] Laidlaw, C., Singla, S., & Feizi, S. (2021). “Perceptual adversarial robustness: Defense against unseen threat models”. ICLR.
The project can be for both a bachelor or master thesis or for a semester project. Max 1-2 people. A student should specialise in Computer science and/or Data science.
If interested please contact Dr Grigorios Chrysos for details.
Synthesizing high dimensional signals, such as image or audio, has been a long standing problem in machine learning. On one hand, tremendous progress has been observed on the task of image synthesis [1-3]. On the other hand, synthesizing different types of signals, e.g. audio, has received little attention in the last few years. Generative Adversarial Nets (GANs) [1], which have exhibited stellar performance on image generation [2, 3], have also been partially used for audio synthesis [4]. However, this work considers the time-frequency representation of the signal (e.g. Short Time Fourier Transform) collapses the inherently complex information into a two-channel image. Our goal in this work is instead to develop a method that works in the complex domain. The project will involve a fair amount of modelling; familiarity with both complex analysis and neural networks is required. This model is expected to generalise beyond audio synthesis in tasks that have inherently complex computations, e.g. tasks that rely on Fourier analysis.
[1] Goodfellow, I., et al. “Generative adversarial nets.”, NIPS 2014.
[2] Karras, T., et al. “A style-based generator architecture for generative adversarial networks.”, CVPR 2019.
[3] Chrysos, G., et al. “Deep Polynomial Neural Networks.”, T-PAMI 2021.
[4] Engel, Jesse, et al. “Gansynth: Adversarial neural audio synthesis.”, ICLR 2019.
The project can be for both a bachelor or master thesis or for a semester project. It is an individual project. A student should specialise in Computer science and/or Data science.
Neural networks have demonstrated they are prone to malicious attacks. Indeed, targeted attacks (e.g. by modifying the input with adversarial noise [1]) can be detrimental for the performance of networks, even under favorable hyper-parameter settings [2]. One of the most successful defenses on such attacks are the adversarial training (AT). However, despite the progress in building stronger defenses using heuristics, our understanding of the core ideas behind AT is still lacking. In this project, we intend to study the defense mechanisms and understand the role of the hyperparameters in AT.
[1] Szegedy, C., Zaremba, W., Sutskever, I., Bruna, J., Erhan, D., Goodfellow, I., & Fergus, R. (2014). “Intriguing properties of neural networks”. ICLR.
[2] Zhu, Z., Liu, F., Chrysos, GG, Cevher, V. (2022) “Robustness in deep learning: The good (width), the bad (depth), and the ugly (initialization)”. NeurIPS.
Prerequisites and goal: The student should be willing to learn the required tools rapidly. The goal is to lead this to a publication into a top ML conference.
This project is intended for a single student. It is available for computer science, communication systems, data science and cyber security students. If interested please contact Dr Grigorios Chrysos for details.
In domain adaptation, the typical assumption that the train and test data are drawn from the same distribution is relaxed [1]. In practice, due to e.g., non-stationarity of the environment, the train and test data come from different distributions. When dealing with multiple clients/users/nodes e.g., in a federated learning scenario, the training data of different clients are drawn from different distributions [2]. Such data heterogeneity is a major challenge to obtain a model that performs well at the test time for a client. In this project, we plan to use a domain adaptation approach and obtain the best personalized model in a federated setting.
[1] Masashi Sugiyama, Matthias Krauledat, and Klaus-Robert Muller. Covariate shift adaptation by importance weighted cross validation. Journal of Machine Learning Research, 8(5), 2007.
[2] P. Kairouz et al. Advances and open problems in federated learning. Foundations and Trends in Machine Learning, Vol 4, 2021.
The project can be for both a master thesis and a semester project students. Computer science, math, and data science students will be a good fit for the project. Max 1-2 students.
If interested please contact Thomas Pethick for details.
Two desired ethical concepts when learning a model are privacy and fairness. These concepts are more important in real-world federated settings with sensitive data. There are fundamental trade-offs between fairness and privacy. Intuitively, while guaranteeing fairness requires explicit knowledge about the membership of individuals in specific subgroups, privacy-preserving algorithms obscure such information to be revealed through the model. In other words, a model cannot be independent of individuals and has reasonable performance on minorities at the same time. Recent studies have shown that differentially-private algorithms may lead to having disparate impacts on specific subgroups, which mostly affect minorities [1,2]. One potential solution is to use personalization or updating the shared model on individual clients locally after optimizing a shared model. In this project, we plan to investigate personalization methods, design new optimisation frameworks, and use various notions of privacy to achieve both privacy and fairness.
References:
[1] E. Bagdasaryan, O. Poursaeed, and V. Shmatikov. Differential privacy has disparate impact on model accuracy. In Proc. NeurIPS, 2019.
[2] D. Pujol, R. McKenna, S. Kuppam, M. Hay, A. Machanavajjhala, and G. Miklau. Fair decision making using privacy-protected data. In Conference on Fairness, Accountability, and Transparency, 2020.
The project can be for both a master thesis and a semester project students. Computer science, math, and data science students will be a good fit for the project. Max 1-2 students.
If interested please contact Thomas Pethick for details.
Two major issues in federated learning are communication efficiency and data heterogeneity. In order to reduce communication costs, several techniques have been studied in the literature. One idea is to reduce the frequency of communication, e.g., in local SGD or FedAvg type algorithms [1]. In addition, various quantization and sparsification methods have been proposed to compress computed gradients or model updates before transmitting to another node. Among various quantisation schemes, unbiased quantization schemes are popular due to achieving full-precision accuracy and enjoying strong theoretical guarantees [2,3]. These communication-efficient schemes are often heuristic and fixed over the course of training. We can think of various parameters such as the number of local updates, quantization levels, and the number of quantization levels that can be optimized to further accelerate training. Recently, adaptive quantization schemes are proposed, where multiple workers update their compression schemes in parallel by efficiently computing sufficient statistics of a parametric distribution [3]. However, the adaptive methods in [3] do not address data heterogeneity issues. It is important to design new adaptive communication-efficient schemes taking into account data heterogeneity of clients suitable for use in federated settings.
References:
[1] H. B. McMahan, E. Moore, D. Ramage, S. Hampson, and B. A. y Arcas. Communication- efficient learning of deep networks from decentralized data. In Proc. AISTATS, 2017.
[2] D. Alistarh, D. Grubic, J. Z. Li, R. Tomioka, and M. Vojnovic. QSGD: Communication- efficient SGD via gradient quantization and encoding. In Proc. NeurIPS, 2017.
[3] F. Faghri, I. Tabrizian, I. Markov, D. Alistarh, D. M. Roy, and A. Ramezani-Kebrya. Adaptive gradient quantization for data-parallel SGD. In Proc. NeurIPS, 2020.
The project can be for both a master thesis and a semester project students. Computer science, math, and data science students will be a good fit for the project. Max 1-2 students.
If interested please contact Thomas Pethick for details.
Being immune to security risks due to Byzantine machines that might be compromised by an adversary, and thus vulnerable to data/model poisoning and tailored attacks is an important concern in federated learning [1]. While several communication-efficient and robust schemes have been developed to accelerate training and tackle security risks, guaranteeing fairness notions have typically not been considered in the current algorithmic designs. Recently, it has been shown that personalization can improve fairness and robustness simultaneously [2]. In this project, we plan to explore vulnerabilities of federated settings and design new aggregation schemes to improve both resilience and fairness in federated settings considering data heterogeneity and communication constraints.
References:
[1] M. Fang, X. Cao, J. Jia, and N. Gong. Local model poisoning attacks to byzantine-robust federated learning. In Proc. USENIX Security Symposium, 2020. [2] T. Li, S. Hu, A. Beirami, and V. Smith. Ditto: Fair and Robust Federated Learning Through Personalization. In Proc. ICML, 2021.
The project can be for both a master thesis and a semester project students. Computer science, math, and data science students will be a good fit for the project. Max 1-2 students.
If interested please contact Thomas Pethick for details.
Langevin dynamics is a first-order sampling algorithm that has gained a lot of attention in the last years. Its similarity to gradient descent in optimisation makes us wonder whether we can transpose existing technique in optimization to sampling. In particular, we would like to design an adaptive version of Langevin dynamics, in the similar fashion as AdaGrad, and prove convergence guarantees with similar improvement as in optimization.
If interested please contact Paul Rolland for details.
Existing federated learning literature focuses on the classical empirical risk minimization (ERM) paradigm and aims to obtain a global model with the implicit assumption that each client has the same training and test data distribution. However, this simplified setting ignores the specific requirements of each client. In this project, we rethink federated learning by focusing on the overall generalization performance on multiple clients and considering both intra-client and inter-client distribution shifts. We plan to modify the classical ERM and obtain an unbiased estimation of the target/true function under distribution shifts; develop an efficient density ratio estimation method for the modified ERM considering the stringent requirements of FL in terms of privacy, and obtain theoretical guarantees for our modified ERM. This is an exciting project at the intersection of statistical learning theory, domain adaptation, and federated learning.
The project can be for both a master thesis and a semester project students. Computer science, math, and data science students will be a good fit for the project. Max 1-2 students.
If interested please contact Dr Ali Ramezani for details.
The double descent phenomenon is well studied in classification and is largely explained away by the so called interpolation regime [1,2]. However, the role of the interpolation regime is less lucid in Generative Adversarial Networks (GANs) both because of the interaction due to the minmax structure but also the stochasticity of the latent variable. This project aims to empirically investigate the effect of the interpolation capacity of both the discriminator and generator. Familiarity with PyTorch and training neural networks is recommended.
[1] Reconciling modern machine learning practice and the bias-variance trade-off, Belkin et al. 2019
This semester project is available for master students only. It is an individual project. Student should specialise in Computer science, Cyber security, Communication systems and/or Data Science.
Federated Learning [1] tries to solve the problem of data privacy in Machine Learning, by training a model across multiple nodes in a network without sharing data. Instead, nodes only share updates to the parameters of the model. In the Decentralized Setting, there is no single main node collecting the updates produced by all worker nodes. Instead, nodes share updates only with their neighbors in the network. In a networked distributed system, decentralized algorithms are deployed when either processors cannot establish a fully connected topology due to communication barriers or the network suffers from high latency [2].
One of the main open problems in Federated Learning is to overcome sources of bias that arise due to the network topology and the heterogeneous nature of the data [3]. This is of great interest in contemporary Machine Learning research, as bias can lead to algorithmic decisions that are unfair, affecting negatively the outcomes for minority groups [4]. Precisely, the fact that nodes only share updates with their friends, can reinforce biases present in social circles, possibly leading to unfair outcomes for minorities, underrepresented clients and marginalized communities.
In this work, we will explore how to exploit structure in Social Networks for the development of unbiased and fair learning algorithms. The links in the network, which are usually public, allow the identification of communities [5] which usually have noticeable differences in the data they generate. Vanilla FL algorithms can introduce undesired biases when applied to this heterogeneous structure, as the learned models can differ vastly between communities.
We will explore the use of community-detection algorithms [6] to design decentralized federated learning algorithms that avoid such biases. We will also provide theoretical guarantees of fairness in a simplified scenario as well as experiments on real data.
[1] Federated Optimization: Distributed Optimization Beyond the Datacenter. Jakub Konečný, Brendan McMahan, Daniel Ramage. https://arxiv.org/abs/1511.03575
[2] Hanlin Tang, Shaoduo Gan, Ce Zhang, Tong Zhang, and Ji Liu. Communication compression for decentralized training. https://arxiv.org/abs/1803.06443
[3] Advances and Open Problems in Federated Learning. Peter Kairouz, H. Brendan McMahan et al. https://arxiv.org/abs/1912.04977
[4] Fairness and Machine Learning: Limitations and Opportunities. Solon Barocas, Moritz Hardt, Arvind Narayanan. https://fairmlbook.org
[5] Stochastic blockmodels: First steps. Paul W.Holland, Kathryn Blackmond Laskey, Samuel Leinhardt.https://www.sciencedirect.com/science/article/pii/0378873383900217?via%3Dihub
Recently the better generalisation capabilities of ensembles of independently trained networks were explained by the networks learning distinct high level features [1]. However, it remains an open problem whether training a single network can achieve the same accuracy without distillation. Dropout provides one method of implicitly training an ensemble but is insufficient in its vanilla stochastic variant. This project intends to investigate whether an adversarial dropout scheme, where adversarially picked features are dropped during training, can provide a stronger ensemble. This follows the growing line of work employing minmax dynamics to train more robust models. Familiarity with PyTorch and training neural networks is recommended.
[1] Towards Understanding Ensemble, Knowledge Distillation and Self-Distillation in Deep Learning, Allen-Zhu and Li 2020
This semester project is available for master students only. It is an individual project. A student should specialise in Computer science, Cyber security, Communication systems and/or Data Science.
Lottery ticket hypothesis [1] is a significant topic in pruning over-parametrized neural networks, leading to more efficient training [3, 4]. The lottery ticket hypothesis states that inside every larger (over-parametrized) network, there is a sub-network that when trained in isolation it provides almost the same performance, but with reduced parameters. Recently, the lottery ticket has been extended to adversarial perturbations [2]. The hypothesis is that there is a sub-network that is robust to adversarial noise. However, the question of whether the hypothesis generalizes to arbitrary tasks is still open. In other words, given an over-parametrized network, can we always find a sub-network that fulfills some predifined condition? Such a hypothesis would be particularly useful in various tasks, such as achieving differential privacy, detecting bias etc.
[1] ‘The Lottery Ticket Hypothesis: Finding Sparse, Trainable Neural Networks’, J Frankle, M Carbin, ICLR 2019.
[2] ‘The Search for Sparse, Robust Neural Networks’, J Cosentino, F Zaiter, D Pei, J Zhu.
[3] ‘Drawing Early-Bird Tickets: Towards more efficient training of deep networks’, H You, C Li, P Xu, Y Fu, Y Wang, X Chen, R G. Baraniuk, Z Wang, Y Lin, ICLR 2020.
[4] `Pruning neural networks without any data by iteratively conserving synaptic flow’, H Tanaka, D Kunin, D L. K. Yamins, S Ganguli, NeurIPS 2020.
This project is available for both a semester project and an MSc thesis. If interested please contact Dr Grigorios Chrysos for details.
Self-supervised learning relies on a simple idea: can we learn supervised tasks without labels? In particular, can we construct a ‘fake task’ using only unlabelled data such that we can learn useful representations for the supervised task we want? Given the fact that self-supervised learning relies only on unsupervised data, its success in the last few years [1-3] is remarkable (i.e., reaching performance close to supervised learning in classification). In this project, we would like to study self-supervised learning under a more realistic setting, where the data are more constrained, e.g., we have only limited data available, or the data are not uniform (which is a realistic hypothesis). The project will include the following two components:
- Design a new self-supervised task appropriate for the cases of such data constraints.
- Study the theoretical benefits of this task for various cases.
[1] “Bootstrap your own latent: A new approach to self-supervised Learning”, Jean-Bastien Grill , et al., NeurIPS 2020.
[2] “Self-Supervised Learning with Kernel Dependence Maximization”, Yazhe Li, et al., 2021.
[3] “Barlow Twins: Self-Supervised Learning via Redundancy Reduction”, Jure Zbontar, et al., ICML 2021.
Prerequisites and goal: The student should be highly motivated, and have either a theoretical background or strong coding skills. The goal is to lead this to a publication into a top ML conference.
If interested please contact Dr Grigorios Chrysos for details.
The project can be for both a bachelor or master thesis or for a semester project. A student should specialise in Computer science, Cyber security, Communication systems and/or Data Science.
LIONS is collaborating with Isochronic AG, developing a novel type of pick-and-place robot which is able to pick and place multiple objects in parallel via a new type of robotic arm.
The fact that multiple objects can be manipulated at once is at the same time a critical advantage as well as a key technical challenge. Specifically, the control strategy has a great impact on the total time of transport. For example, a bad strategy would be to pick the products at the top of the pick zone with the bottom rail and vice versa, because this would require unnecessary up-down movements of the carrier arm which represents the vast majority of the robot’s moving mass.
The goal of this project is to use to find the best sequence of pick and place movements in order to reduce the total time. To this end, Isochronic will provide a model of the robot’s kinematic and a set of customer applications.
The problem will involve working with reinforcement learning:
https://docs.ray.io/en/master/rllib-algorithms.html
https://www.mdpi.com/2076-3417/9/2/348
https://arxiv.org/abs/2001.03792
https://arxiv.org/abs/2102.04022
and unbalanced optimal transport:
http://proceedings.mlr.press/v119/pham20a.html
https://www.ams.org/journals/mcom/2018-87-314/S0025-5718-2018-03303-8/home.html
https://www.jmlr.org/papers/volume22/20-451/20-451.pdf
Prerequisites: familiarity with jax, pytorch or tensorflow 2+ is required.
If interested please contact Igor Krawczuk for details.
The project can be for both a bachelor or master thesis or for a semester project. A student should specialise in Computer science, Cyber security, Communication systems and/or Data Science.
The project can be for both a bachelor or master thesis or for a semester project. A student should specialise in Computer science, Cyber security, Communication systems and/or Data Science.
The project can be for both a bachelor or master thesis or for a semester project. A student should specialise in Computer science, Cyber security, Communication systems and/or Data Science.
Machine learning classifiers working on high dimensional data exhibit susceptibility to so called “adversarial examples”, wildly misidentifying inputs presented to them when modified with specifically crafted small perturbations. While part of this behavior has been explained by the concentration of measure phenomenon and types of features learned by the classifiers, there seem to be additional drivers of adversarial risk not yet captured by current frameworks. This project – which can be adapted to either Masters or Semester projects – investigates these drivers, aiming at mathematical analysis, the creation of toy problems and benchmarks which can be used to gauge the sources of adversarial susceptibility a given model contains, as well as their severity. This is a challenging project and for both master or semester projects, you should be comfortable with Julia, pytorch or tensorflow, as well as have general background on the field.
If interested please contact Igor Krawczuk for details
Characterising novel circuit elements and developing compact behavioural models is an important step before they can be used in circuit design. However creating and tuning these models can be a tedious process. This project is a first exploration of using a SPIRAL inspired algorithm for the synthesis of compact models by synthesising SPICE circuit models.
If interested please contact Igor Krawczuk for details
Neural network models are increasingly deployed in safety critical and data sensitive settings, for example in medical diagnostics. This project concerns the study of the interactions of various strategies to learn disentangled latent representations with interpretability and techniques meant to help user understand and reason about the features used by the network to give a certain output, their sensitivity to various forms of adversarial perturbations and distributional shifts as well as their susceptibility to attacks like the model inversion attack which is a concern when private data is used to train widely distributed models.
If interested please contact Igor Krawczuk for details
The project can be for both a bachelor or master thesis or for a semester project. A student should specialise in Computer science, Cyber security, Communication systems and/or Data Science.
The project can be for a master thesis or for a semester project.
Details of the project can be found here
If interested please contact Thomas Sanchez for details
The project can be for a master thesis or for a semester project.
Details of the project can be found here
If interested please contact Thomas Sanchez for details
[3] ‘Batch Normalization: Accelerating Deep Network Training by Reducing Internal Covariate Shift’, Sergey Ioffe, Christian Szegedy, ICML 2015.
If interested please contact Dr Grigorios Chrysos for details.
This projects aims to create a Python library that collects many real world variational inequality (saddle point problem) instances and some popular solvers.
If interested please contact Yurii Malitskyi for details
It has been empirically shown that carefully ordering the training examples improve the learning speed of SGD based algorithms. There very few works that attempt to provide some theoretical insights into this phenomenon. In this project, we aim to investigate (both theoretically and empirically) the curriculum strategies for adaptive optimization methods.
If interested please contact Parameswaran Kamalaruban for details.
This projects aims to find a robust optimization method that will perform well in many scenarious for deep learning. A student should be already familiar how to train neural networks.
If interested please contact Yurii Malitskyi for details
Bundle methods for non-smooth optimisation were widely used in the literature [1,2,3]. The main idea of this method is to construct a piecewise linear approximation to the objective function. This construction is based on the sub-gradient of the previous iteration.
The objective of this project is to develop stochastic bundle methods and investigate their convergence, for solving non-smooth optimization of minimizing the sum of two functions f+g, where f is proximable and g can be decomposition into the finite sum of g_i.
References:
[1] Yu Du, Andrzej Ruszczynski, Rate of Convergence of the Bundle Method
[2] Alexander J. Smola, S.V. N. Vishwanathan, Quoc V. Le, Bundle Methods for Machine Learning.
[3] C. H. Teo, S.V. N. Vishwanathanm A. Smola, Quoc. V.le, Bundle Methods for Regularized Risk Minimization, Journal of Machine Learning Research 11 (2010) , 311-365
The high-throughput screening of large databases of novel materials candidates constitutes a central goal of the recently awarded MARVEL NCCR grant. Given a training set of compounds with pre-calculated quantum mechanical properties, we seek to construct supervised machine learning models that accurately infer the corresponding properties for similar materials with correctness guarantees [1-4].
References
[1] A. P. Bartók, R. Kondor and G. Csányi , On representing chemical environments, Phys. Rev.., vol. 87, pp. 184115-1–184115-16, 2013.
[2] S. De, A. P. Bartók, G. Csányi and M. Ceriotti, Comparing molecules and solids across structural and alchemical space, Phys. Chem. Chem. Phys., vol. 20, pp. 13754–13769, 2016.
[3] Y. Nesterov, Introductory lectures on convex optimization. A basic course. Kluwer Academic Publishers,Boston, MA, 2004.
[4] M. Rupp, Machine Learning for Quantum Mechanics in a Nutshell, Quantum Chemistry, vol. 115(16), pp. 1058–1073, 2015.
We consider the problem of finding a global maximizer (or minimizer) of an unknown objective function from point evaluations. Bayesian optimization prescribes a prior belief over the possible objective functions and then sequentially refines this model as data are observed.
The student will develop new methods for Bayesian optimization that are both theoretically well founded and practically applicable.The methods will be tested on various real-world problems such as finding a good set of hyperparameters for deep neural networks or discovering molecules with good chemical properties.
Keywords: Bayesian optimization, Gaussian process, Multi-armed bandits
Requirements: Python, machine learning, familiarity with multi-armed bandits and submodular optimization is a plus.
References: [4], [1], [3], [2]
References
[1] Ilija Bogunovic, Jonathan Scarlett, and Volkan Cevher. Time-Varying Gaussian Process Bandit Optimization. In International Conference on Artifcial Intelligence and Statistics (AISTATS), 2016.
[2] Ilija Bogunovic, Jonathan Scarlett, Andreas Krause, and Volkan Cevher. Truncated Variance Reduction: A Uni_ed Approach to Bayesian Optimization and Level-Set Estimation. In Conference on Neural Information Processing Systems (NIPS), 2016.
[3] Josip Djolonga, Andreas Krause, and Volkan Cevher. High-dimensional gaussian process bandits. In C. J. C. Burges, L. Bottou, M. Welling, Z. Ghahramani, and K. Q. Weinberger, editors, Advances in Neural Information Processing Systems 26, pages 1025-1033. Curran Associates, Inc.,2013.
[4] Bobak Shahriari, Kevin Swersky, Ziyu Wang, Ryan P Adams, and Nando de Freitas. Taking the human out of the loop: A review of bayesian optimization. Proceedings of the IEEE, 104(1):148-175, 2016.
Submodular functions are set functions, i.e., functions defined on the power set 2V of a given ground set V,, that satisfy a diminishing return property. They occur naturally in several applications in computer vision, machine learning, economics and theoretical computer science. Submodular functions have very interesting links to convex analysis and can be minimized exactly and maximized approximately by efficient algorithms with provable guarantees.
The objective of this project is to develop methods to improve on the theoretical and/or practical efficiency of submodular minimization/maximization algorithms in offline or streaming settings.
Requirements: MATLAB, familiarity with Submodular and Convex Optimization, and Machine Learning.
References:
[1] Fujishige, Satoru. \Submodular functions and optimization”. Vol. 58. El-sevier, 2005.
[2] Bach, Francis. \Learning with submodular functions: A convex optimization perspective.” arXiv preprint arXiv:1111.6453 (2011).
[3] Iyer, Rishabh, and Je_ Bilmes. \Polyhedral aspects of Submodularity, Convexity and Concavity.” arXiv preprint arXiv:1506.07329 (2015).
- Low-power analog-to-digital conversion for multichannel neural recording
- Hardware-friendly selective feature extractors for neural classification tasks
- Ultra-wideband (UWB) wireless data transfer for neural recording implants
If interested, please submit your applications to [email protected] with your CV, statement of results and a short paragraph describing your motivation and background to undertake the project.
We are interested in reducing the scan times in Magnetic Resonance Imaging (MRI) using compressive sensing techniques. For this purpose, we will design sampling mechanisms via a new learning-based approach, which unifies learning theory and the sampling theory. We also consider extensions to multi-coil MRI and provide demonstrations with real scans.
Keywords: Compressive Sensing, Fourier Transform, Image Reconstruction.
Requirements: MATLAB, Signal/Image Processing knowledge, convex and combinatorial optimization
In the inverse reinforcement learning (IRL) setting, the reward of the environment is not defined. Instead, the agent is provided with a set of expert demonstrations, and the agent tries to recover a reward function such that the expert behavior is optimal. Typically in IRL, the transition dynamics of the environment are assumed to be known. And only a few recent works attempt to learn both the reward and the transition dynamics via little interaction with the environment or in a truly-batch manner. In this project, we revisit this problem more systematically in light of new advancements in model-based RL.
Person of contact was Parameswaran Kamalaruban
In the inverse reinforcement learning (IRL) setting, the reward of the environment is not defined. Instead, the agent is provided with a set of expert demonstrations, and the agent tries to recover a reward function such that the expert behavior is optimal. Consider a setting where expert demonstrations are provided for only a few environments. Based on these demonstrations, we aim to learn a policy that is robust against a larger class of environments (MDPs).
Person of contact was Parameswaran Kamalaruban
With the massive increase of available data for machine learning research, designing optimization algorithms that could handle large volumes of data is getting more and more important. For that purpose, we are interested in developing convex optimization algorithms that can be used in distributed environment.
In this project, the algorithms will be implemented and tested using Google’s machine learning library TensorFlow.
Keywords: convex optimization, distributed optimization, tensorflow
Requirements: Python, C++, familiarity with optimization is a plus
Details of the project can be found here: Neural Network Based Hardware Decoder Design
“Although this may seem a paradox, all exact science is dominated by the idea of approximation.”
–Bertrand Russell (1872–1970)
We are interested in developing approximation algorithms with provable estimation guarantees for structured sparse representations of spatial images and temporal data streams.
Students with experience in Hadoop MapReduce and/or Cuda programming are given priority.
Keywords: approximation guarantee, dynamic programming, greedy algorithm, submodularity, union-of-subspace models, randomized algorithms
Requirements: Matlab, C/C++
We are interested in developing algorithms that enable robust decompositions of large-scale data matrices for learning applications.
Keywords: randomized algorithms, fixed-rank approximation problem, approximation guarantees
Requirements: Matlab or C/C++
We are interested in studying non-iid, parametric and non-parametric prior distributions with almost sure compressibility properties. This project pays particular attention to exchangeable distributions defined over binary and quad-trees.
Keywords: compressible priors, order statistics, almost sure convergence, De Finetti’s theorem
Requirements: Matlab, C/C++
We are interested in the application of extremal combinatorial objects in compressive sensing problems, such as randomness extractors and expanders. This project pays particular attention to a recent development in information theory: polar codes.
Keywords: randomness extractors, expanders, polar codes, compressive sensing
Requirements: Matlab or C/C++
We are interested in characterizing the (set) restricted singular values of pseudo-random matrices.
Keywords: matrix martingales, tail bounds, concentration-of-measures
Requirements: Matlab, C/C++
We are interested in making inferences from biological data in order to understand the processes that cause a disease, potentially leading to the identification of therapies.
Within the machine learning framework of supervised linear regression, we want to exploit structured sparse models to both improve prediction performance and interpretability of the results. Structured sparse model encompass models such as hierarchical trees, group structures or union of subspaces model, which offer the possibility of estimating a linear model that can be more easily interpreted by clinicians.
Our aim is to develop and characterize new algorithms and structures to be applied to cancer data and neuroimaging data. This project had both theoretical and computational aspects.
In the classical Markowitz portfolio optimization, one is interested in allocating a fixed amount of capital among a set of assets so as to minimize the risk/variance of the portfolio return, subject to a target empirical return. Assuming that the true mean and covariance of the set of assets are known, the Markowitz portfolio problem has a closed form solution. In practice though, this assumption is unrealistic and we are usually restricted to some empirical estimates of the mean and the covariance from recorded data. Unfortunately, such compromise introduces several problems: in general, financial data are well-known to be non-stationary (which limits the amount of meaningful data in the estimates) while classic ML techniques are robust only in the asymptotic sense where a vast amount of data is required for a good estimate. We aim to improve on these approaches by exploiting other properties of the data, such as the sparsity in the covariance matrix or other low-dimensional assumptions.
During this project, the student learn modern techniques in sparse covariance matrix estimation as well as new scalable optimization algorithms. Our aim is to develop and characterize covariance estimators from a limited amount of data, leveraging sparse promoting and other low-dimensional models in the estimation. As part of the project, the student applies this knowledge to real world data and track progress on the performance of the designed portfolios. This project has both theoretical and computational aspects.
Recovering a large matrix from a small subset of its entries is a challenging problem arising in many real applications, such as recommender systems. Many existing approaches formulate this problem as a general low-rank matrix approximation problem, which may be used to impute unobserved entries. This approach leads to good performance even when very few are known.
We are interested in bandit optimization in this setting which introduces the exploration/exploitation tradeoff, where we actively decide on the sampling positions to optimize a given convex criteria.
We propose a dimensionality reducing matrix design based on training data with constraints on its Frobenius norm and number of rows. Our design criteria is aimed at preserving the distances between the data points in the dimensionality reduced space as much as possible relative to their distances in original data space. This approach can be considered as a deterministic Bi-Lipschitz embedding of the data points.
We introduce a scalable learning algorithm, dubbed AMUSE, and provide a rigorous estimation guarantee by leveraging game theoretic tools. We also provide a generalization characterization of our matrix based on our sample data. We use compressive sensing problems as an example application of our problem, where the Frobenius norm design constraint translates into the sensing energy.
We consider the problem of solving large-scale optimization problems with matrix decision variables. For such problems, classical convex optimization algorithms scale poorly even at moderate data sizes. On the other hand, through cleverly employing the structures of real-world problems, non-convex alternating minimization methods succeed at providing efficient and accurate solutions, albeit sometimes without theoretical guarantees.
In this project, the student will develop novel alternating minimization algorithms, and examine their theoretical convergence. The student will test the applicability of the new methods on various real-world problems including phase retrieval and matrix completion.
Keywords: Alternating minimization, Phase retrieval, Matrix completion.
Requirements: Matlab or Python, basic knowledge in optimization such as gradient descent and its convergence rate, familiarity with phase retrieval or matrix completion is a plus.
References: [1], [2], [3], [4].
References
[1] Emmanuel J Candes, Xiaodong Li, and Mahdi Soltanolkotabi. Phase retrieval via wirtinger ow: Theory and algorithms. IEEE Transactions on Information Theory, 61(4):1985-2007, 2015.
[2] David E Carlson, Volkan Cevher, and Lawrence Carin. Stochastic spectral descent for restricted boltzmann machines. In AISTATS, 2015.
[3] Moritz Hardt. Understanding alternating minimization for matrix completion. In Foundations of Computer Science (FOCS), 2014 IEEE 55th Annual Symposium on, pages 651-660. IEEE, 2014.
[4] Prateek Jain, Praneeth Netrapalli, and Sujay Sanghavi. Low-rank matrix completion using alternating minimization. In Proceedings of the forty-fifth annual ACM symposium on Theory of computing, pages 665-674. ACM, 2013.
Details of the project can be found here Learning Representations Via Weak Supervision Using Deep Learning