Machine learning approaches for the study of protein surfaces
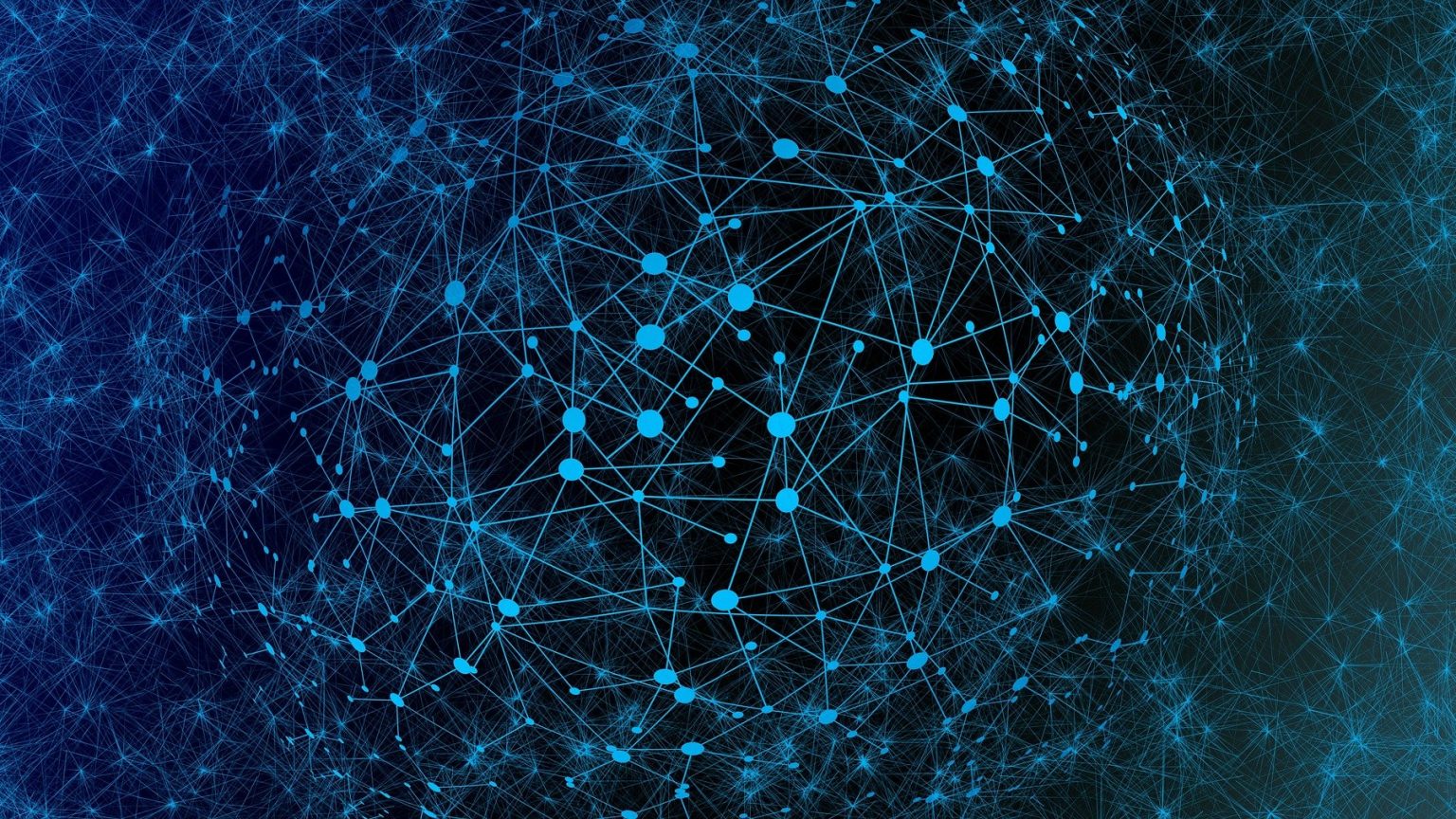
Predicting interactions between proteins and other biomolecules based solely on structure remains a challenge in biology. Protein molecular surfaces display patterns of chemical and geometric features that fingerprint a protein’s modes of interactions with other biomolecules. Our lab hypothesized that proteins participating in similar interactions may share common fingerprints, independent of their evolutionary history. Fingerprints could be learned from large-scale datasets and we therefore developed MaSIF (Molecular Surface Interaction Fingerprinting), a conceptual framework based on a geometric deep learning method to capture fingerprints that are important for specific biomolecular interactions. MaSIF’s proof-of-concept has been demonstrated with three prediction challenges: protein pocket-ligand prediction, protein–protein interaction (PPI) site prediction and ultrafast scanning of protein surfaces for prediction of protein–protein complexes.
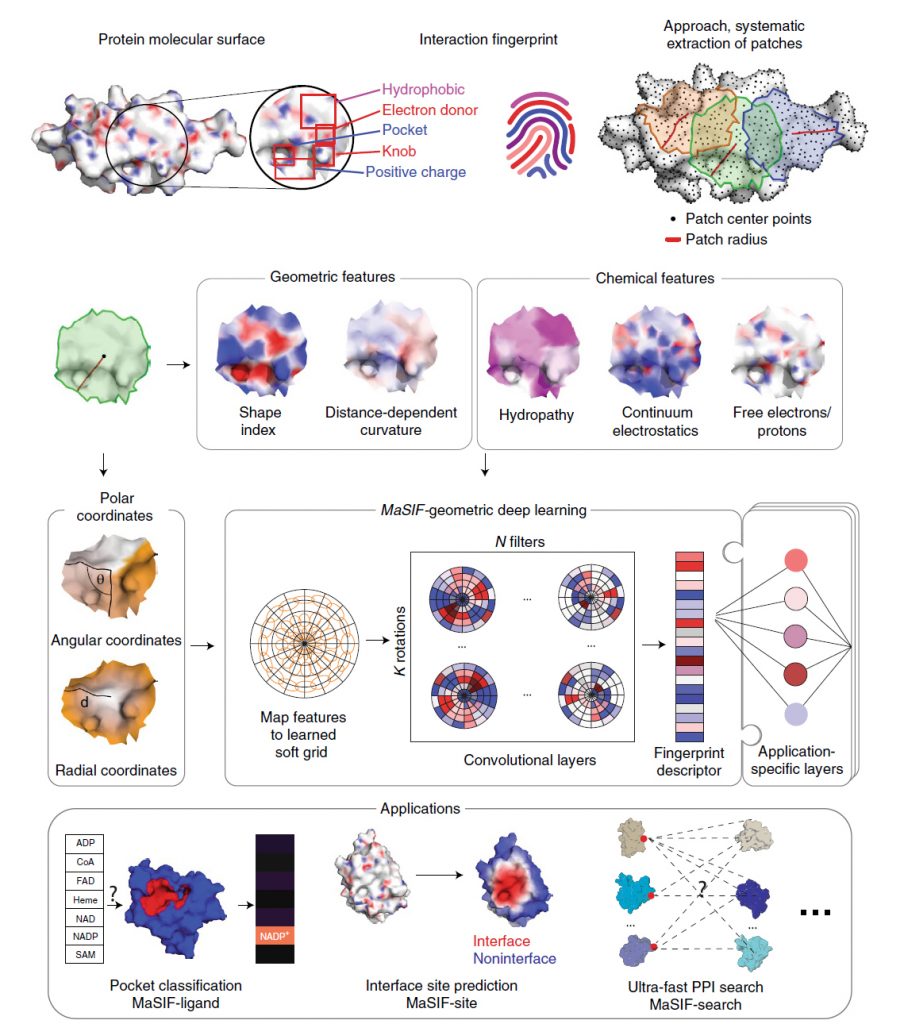
Although MaSIF demonstrated reliable predictions three to four orders of magnitude faster than other state-of-the-art algorithms, the main limitations stem from its reliance on pre-computed meshes and handcrafted features, as well as significant computational time and memory requirements. We therefore developed dMaSIF (differentiable molecular surface interaction fingerprinting), a new architecture free of any pre-computed features. With this new tool in hand, all computations are performed on-the-fly, with minimal memory requirements.
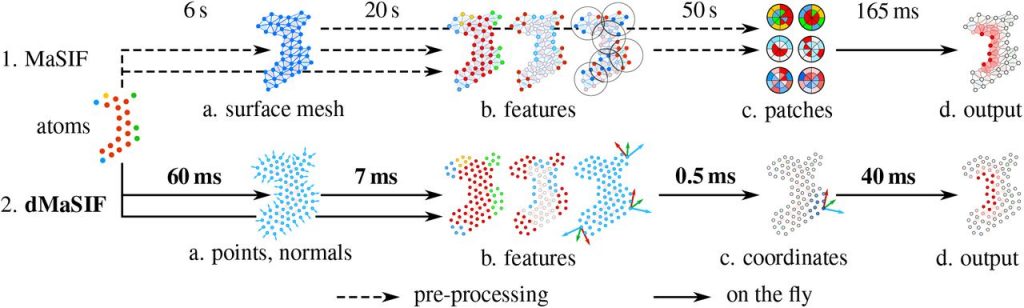
References : Gainza P. et al Nature methods (2020) ; Sverrisson F. et al BioRxiv(2020)