MiBMI: Miniaturized Brain-Machine Interfaces for Brain-to-Text Decoding
The MiBMI project focuses on developing low-complexity, high-performance machine learning algorithms and hardware capable of distinguishing brain-intended activities during brain-machine interface (BMI) tasks such as handwriting. These devices aim to restore lost motor or communication abilities for paralyzed patients by recording, processing, and decoding task-relevant neural activities. By integrating intelligent neural interfaces, we can significantly enhance prosthetic BMIs, improving their efficiency and miniaturization. We have implemented the MiBMI chipset using a 65nm TSMC process, enabling 31-class neural decoding and 192-channel neural recording. The MiBMI achieves software-comparable accuracy while occupying a compact area of 2.46mm² and consuming only 883μW. This progress paves the way for future-generation BMIs to transition from clinical settings into the daily lives of patients, offering them a means to communicate and interact with the world directly through their thoughts.

Neuromodulation Devices for Psychiatric Disorders
This project focuses on developing a closed-loop neuromodulation SoC that introduces novel stimulation paradigms, continuously monitoring and regulating neural connectivity within or across regions through phase-locked deep brain stimulation (DBS). We have implemented on-chip computation of connectivity metrics such as phase-amplitude coupling (PAC) and phase-locking value (PLV) by efficiently approximating the complex nonlinear functions for phase and amplitude computation, resulting in over 60% power savings compared to previous implementations without compromising accuracy. This SoC demonstrated the first-in-literature phase-locked neurostimulator, validated in vivo in rats within regions associated with fear and anxiety. Additionally, it supports multi-mode stimulation control through various combinations of phase-locking events and thresholded connectivity measures. Further advancements can be achieved by integrating an AI model to guide phase-locked DBS control and exploring new biomarkers and connectivity measures to improve detection accuracy and therapeutic efficacy.

Wireless Data Telemetry for Brain Implants
This project focuses on developing the implantable transmitter (TX) for high-density brain implants. To balance data rate, transmission range, implant size, and power consumption, we introduced a co-designed power amplifier and antenna interface that eliminates the need for a lossy and bulky matching network. An energy-efficient phase-locked loop was employed to generate the RF carrier in the UWB band. We also proposed an asymmetric pulse shaping technique to improve spectral efficiency and minimize intersymbol interference. The TX has been fabricated using a 65-nm TSMC process. The wireless module, including a custom-designed antenna, has a footprint of only 49.8mm². In-vitro measurements confirmed the TX can achieve over a 1-m transmission range at data rates up to 500 Mb/s across various implantation depths, highlighting its potential in scenarios requiring high data throughput and extended transmission range under strict power and size limits.

Wireless Power Transmission and Management Circuits for Implantable Neural Interfaces
Recognizing the critical importance of wireless powering medical implants, we focus on enabling seamless and efficient energy transfer to these devices. As medical implants advance and incorporate more sophisticated functionalities, it is crucial to keep their size to a minimum and enhance usability for patients. These implants require many blocks to function at various power and voltage levels, making efficient power management challenging. To address these needs, we specialise in wireless power transmission aiming for an efficient wireless link with a flexible transmission range. As part of our comprehensive approach to power management, we have developed and fabricated sophisticated circuit solutions to regulate rectified voltage using the 65-nm TSMC process. In our designs, we ensure optimal performance and efficiency in supporting these advanced medical devices and contributing to their ongoing development and improvement.

A Hardware-Efficient EMG Decoder for Next-Generation Robotic Prosthetic Hands (RPHs)
We develop hardware-efficient EMG decoders aimed at enhancing the functionality of next-generation Robotic Prosthetic Hands (RPHs). While current commercial RPHs offer limited on/off control, recent machine learning advancements enable precise finger movement decoding. However, the complexity of these models limits their use in portable devices. Future RPH designs must balance portability, low power consumption, and high decoding accuracy for practical use by individuals with disabilities. To this end, we introduce a novel attractor-based neural network to realize on-chip movement decoding for next-generation portable RPHs. We tested our model on healthy subjects, achieving a decoding performance that is higher or comparable to state-of-the-art models such as LSTM and CNN while being 50-120 times more compact. This architecture exhibits minimal hardware complexity and can be effectively integrated as a System-on-Chip, making it an ideal solution for advanced, portable prosthetic hand control.

Closed-loop AI-Enabled Neurostimulation for Neurological Disorders
We develop new ways of personalized monitoring and treatment for early detection and prevention of disabling neurological conditions using smart implantable and wearable technologies. In a closed-loop neural interface, such as a seizure control system, the neural signals recorded by an array of electrodes are amplified, filtered and digitized. A feature extraction processor is subsequently activated to extract the disease-associated biomarkers. Upon abnormality detection, a programmable neural stimulator is triggered to suppress the symptoms of disease (e.g. a seizure or migraine attack, Parkinson’s tremor, memory dysfunction, etc.) through periodic charge delivery to the tissue. For example, our latest NeuralTree chip can classify neural signals from 256 channels in the brain and perform symptom detection or motor decoding with an on-chip classifier. The SoC is versatile and can be used in different applications including epilepsy, movement disorders, and motor decoding in BCIs.

Machine Learning Hardware for Diagnostic Applications
We explore a class of simple, yet sufficiently accurate learning algorithms that allow full integration of processing circuitry with the sensor array in various biomedical applications. Our preliminary results on seizure onset detection suggest that these gradient-boosted ensembles of axis-parallel decision trees are excellent candidates for on-chip integration, with a simple hardware proposed here. Combined with the implemented feature extraction model, these classifiers quickly become competitive with more complex learning models, using only a small number of low-depth shallow trees. We have implemented this architecture in a 65nm TSMC process for epileptic seizure detection. The fabricated SoC was successfully tested on pre-recorded iEEG data (32-channel) from 20 patients, achieving a 41.2nJ/class energy efficiency in a total area of 1mm2, improving the SoA neural classifier by 30× in energy efficiency and 28× in area usage per channel.

Compressive Sensing for High-Density ECoG Recording
The application of compressive sensing (CS) theory has shown promising results for sub-Nyquist sampling of biological signals. However, the occupied die area of a CS system can be significantly larger than a typical acquisition system without compression. To solve this issue, we have proposed an efficient approach to compressive acquisition of cortical signals in wireless neural prostheses. We implement a multichannel CS scheme which exploits the spatial sparsity of the signals recorded from the electrodes of the sensor array. The proposed approach preserves the power efficiency while significantly reducing the area overhead. This design improves the power dissipation of the wireless transmission, and hence boosts the number of channels integrated on an implantable chip.

Closed-loop Neural Implants and Brain-Machine Interfaces
Millions worldwide suffer from motor-related injury or chronic neurological diseases that remain largely undertreated. As an emerging therapeutic alternative, neural devices and prostheses are increasingly being explored to detect neurological abnormalities in real-time, and trigger a therapy such as neurostimulation to restore normal function. Moreover, brain-machine interfaces (BMIs) hold the promise to ultimately restore motor function through cortical or spinal cord stimulation. Clinical studies currently require multiple computers and recording systems to function, resulting in bulky hardware that is not convenient for daily use. A bottleneck for local processing is the lack of low-power and area-efficient classifiers that can accurately predict the symptoms of disease or decode a motor task in real-time. We propose to employ state-of-the-art ML principles and efficient hardware architectures to enable real-time neural data processing on the implant. This project is funded by a 2018 Google Faculty Research Award in Machine Learning.

Migraine Diagnosis: Toward a Wearable Solution
Migraine is the most common neurological disorder, characterized by recurrent headache attacks. Despite its high prevalence, migraine diagnosis is still mainly based on clinical interviews and patient diaries. Automatic detection of migraine state using electrophysiological recordings may play a key role in migraine diagnosis using wearables and early treatment. Migraineurs are characterized by a deficit of habituation in cortical information processing, causing abnormal changes of somatosensory evoked potentials (SSEPs). We recently used an ML approach to utilize SSEP biomarkers for migraine state prediction in a noninvasive setting. Our analysis shows that high-frequency oscillations (HFOs) embedded in SSEP are important biomarkers for migraine state prediction. Using a set of relevant features, we successfully separated migraine patients during and between attacks from healthy controls with over 80% accuracy. This is a collaborative project with the Neurophysiology Department at IRCCS in Rome, Italy.
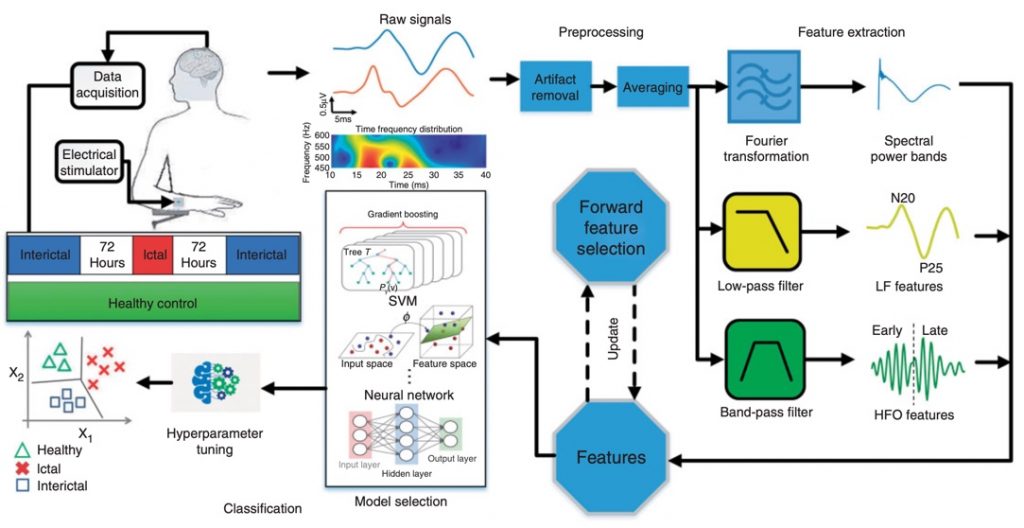
Parkinsonian Tremor Detection for Adaptive DBS
Parkinson patients treated by the conventional DBS may suffer from side effects such as speech impairment and psychiatric symptoms. Moreover, open-loop stimulation increases the energy consumption of the DBS device, which may require a surgical replacement procedure. Alternatively, the concept of adaptive DBS (aDBS) holds the promise to alleviate side effects and improve the efficacy of conventional open-loop DBS. However, current aDBS techniques are primarily based on single-feature thresholding, precluding an optimized delivery of stimulation and precise control of motor symptoms. We used multiple features of local field potentials (LFPs) recorded from STN, and combined it with machine learning and Kalman filtering to improve the accuracy of detecting resting tremor in Parkinson’s. We found that by optimal selection of only five features using a forward feature selection method, the system can achieve an average F1 score of up to 91.8%. This is a collaborative project with the Clinical Neuroscience Department at the University of Oxford.
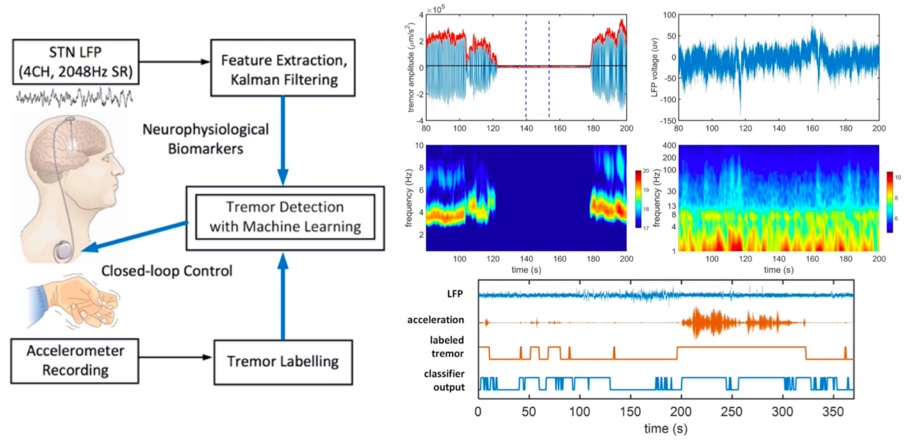