Prof. Felix Schürmann Google Scholar Profile
Fully Peer-Reviewed and Published Articles and Proceedings
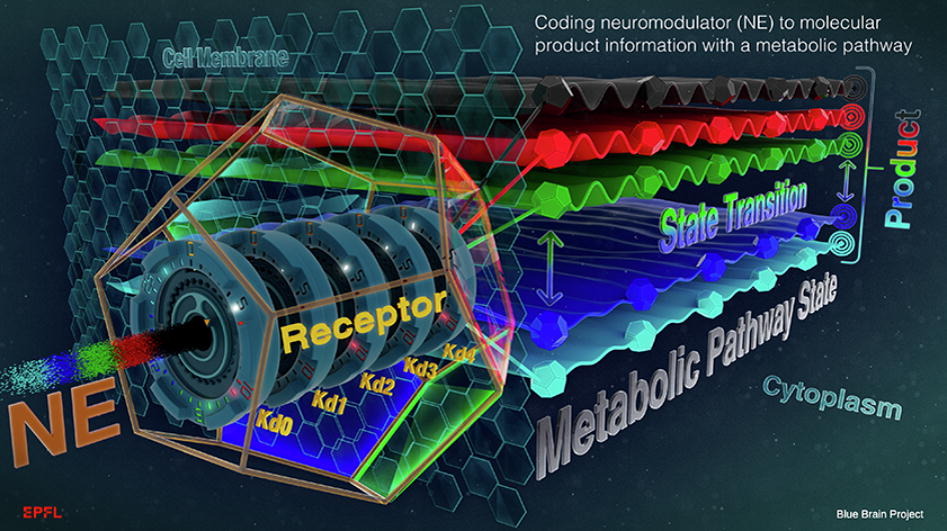
Coggan, J. S., Keller, D., Markram, H., Schürmann, F., & Magistretti, P. J. (2022). Representing stimulus information in an energy metabolism pathway. Journal of Theoretical Biology,540, 111090.
DOI: https://doi.org/10.1016/j.jtbi.2022.111090
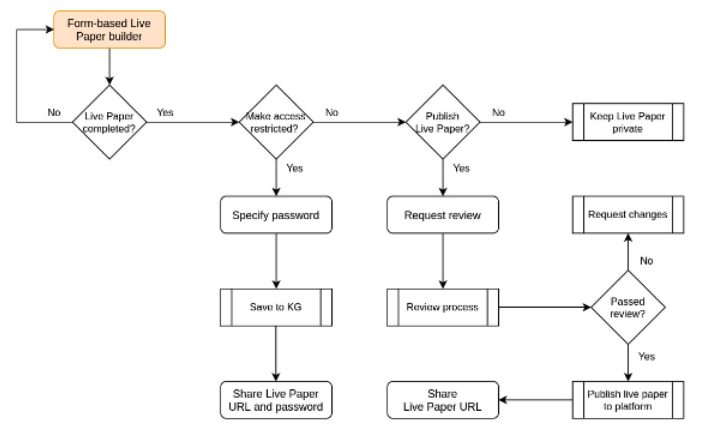
Appukuttan, S., Bologna, L. L., Migliore, M., Schürmann, F., & Davison, A. P. (2021). EBRAINS Live Papers—Interactive resource sheets for computational studies in neuroscience. OSF Preprint. 2 October 2021.
DOI: https://osf.io/4uvdy
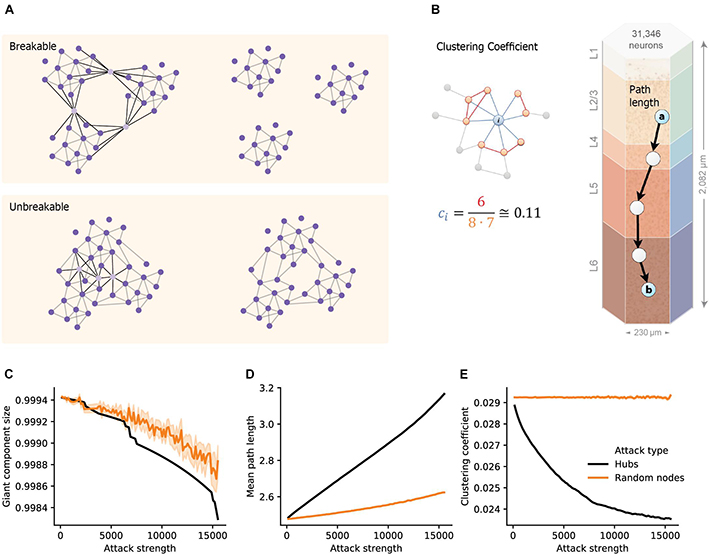
Gal, E., Amsalem, O., Schindel, A., London, M., Schürmann, F., Markram, H., & Segev, I. (2021). The role of hubneurons in modulating cortical dynamics. Frontiers in Neural Circuits,15, 96.
DOI: https://doi.org/10.3389/fncir.2021.718270
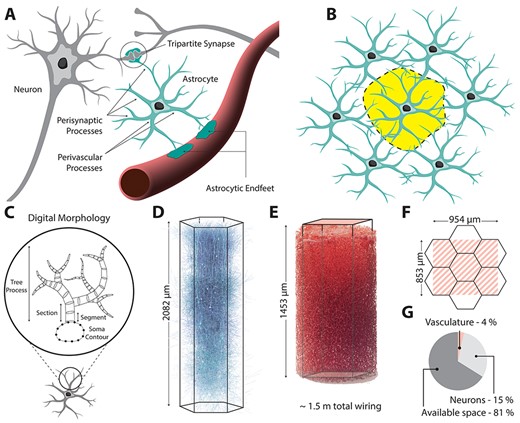
Zisis, E., Keller, D., Kanari, L., Arnaudon, A., Gevaert, M., Delemontex, T., Coste, B., Foni, A., Abdellah, M., Calì, C., Hess, K., Magistretti, P. J., Schürmann, F., & Markram, H. (2021). Digital reconstruction of the neuro-glia-vascular architecture. Cerebral Cortex,31(12), 5686–5703.
DOI: https://doi.org/10.1093/cercor/bhab254
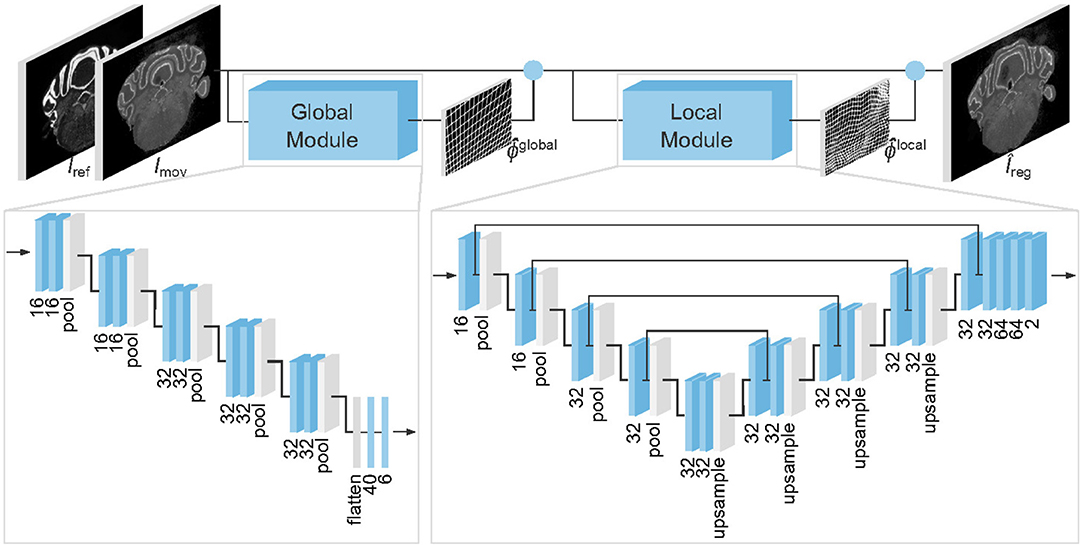
Krepl, J., Casalegno, F., Delattre, E., Erö, C., Lu, H., Keller, D., Rodarie, D., Markram, H., & Schürmann, F. (2021). Supervised learning with perceptual similarity for multimodal gene expression registration of a mouse brain atlas. Frontiers in Neuroinformatics,15, 37.
DOI: https://doi.org/10.3389/fninf.2021.691918
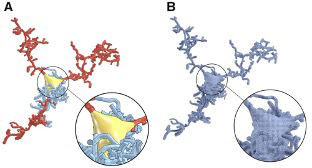
Abdellah, M., Foni, A., Zisis, E., Guerrero, N. R., Lapere, S., Coggan, J. S., Keller, D., Markram, H., & Schürmann, F. (2021). Metaball skinning of synthetic astroglial morphologies into realistic mesh models for visual analytics and in silico simulations. Bioinformatics,37(Supplement_1), i426–i433.
DOI: https://doi.org/10.1093/bioinformatics/btab280
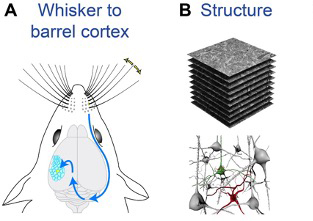
Petersen, C. C. H., Knott, G. W., Holtmaat, A., & Schürmann, F. (2021). Toward biophysical mechanisms of neocortical computation after 50 years of barrel cortex research. Function,2(1) zqaa046. Oxford Univ. Press for the American Physiological Society.
DOI: https://doi.org/10.1093/function/zqaa046
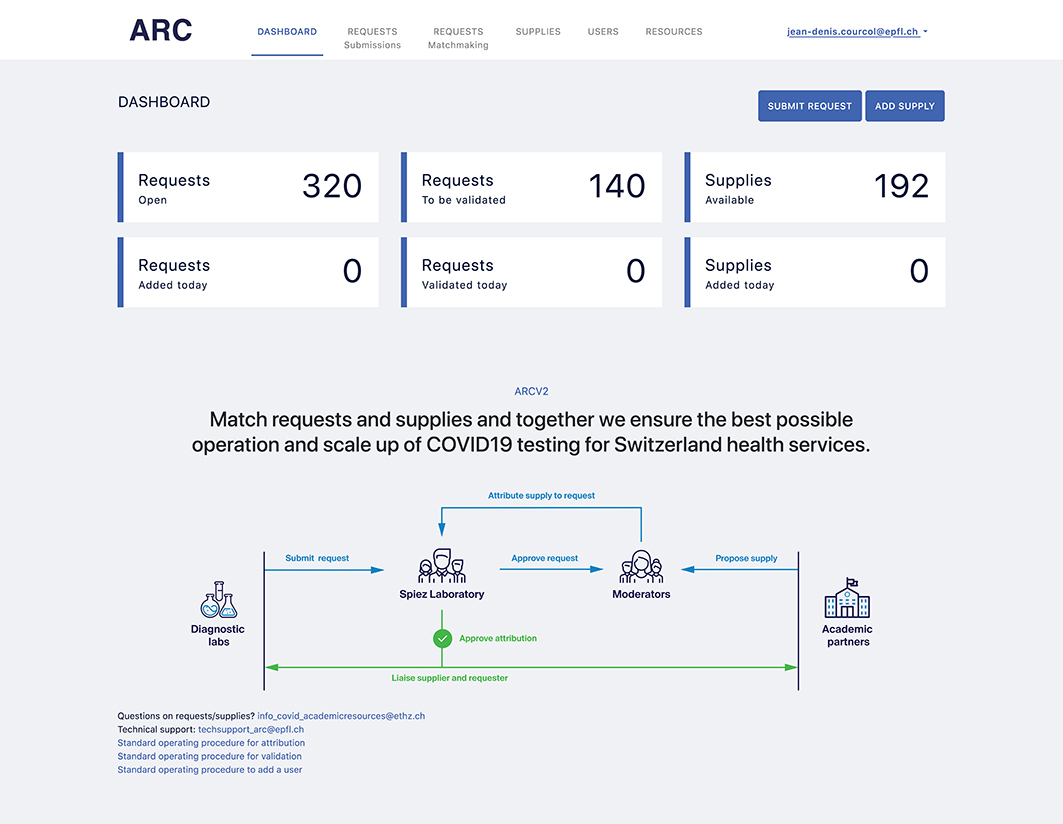
Courcol, J.-D., Invernizzi, C. F., Landry, Z. C., Minisini, M., Baumgartner, D. A., Bonhoeffer, S., Chabriw, B., Clerc, E. E., Daniels, M., Getta, P., Girod, M., Kazala, K., Markram, H., Pasqualini, A., Martínez-Pérez, C., Peaudecerf, F. J., Peaudecerf, M. S., Pfreundt, U., Roller, B. R. K., Słomka, J., Vasse, M., Wheeler, J.D., Metzger, C.M.J.A., Stocker, R., and Schürmann, F. (2021). ARC: An open web-platform for request/supply matching for a prioritized and controlled COVID-19 response. Frontiers in Public Health,9, 71.
DOI: https://doi.org/10.3389/fpubh.2021.607677
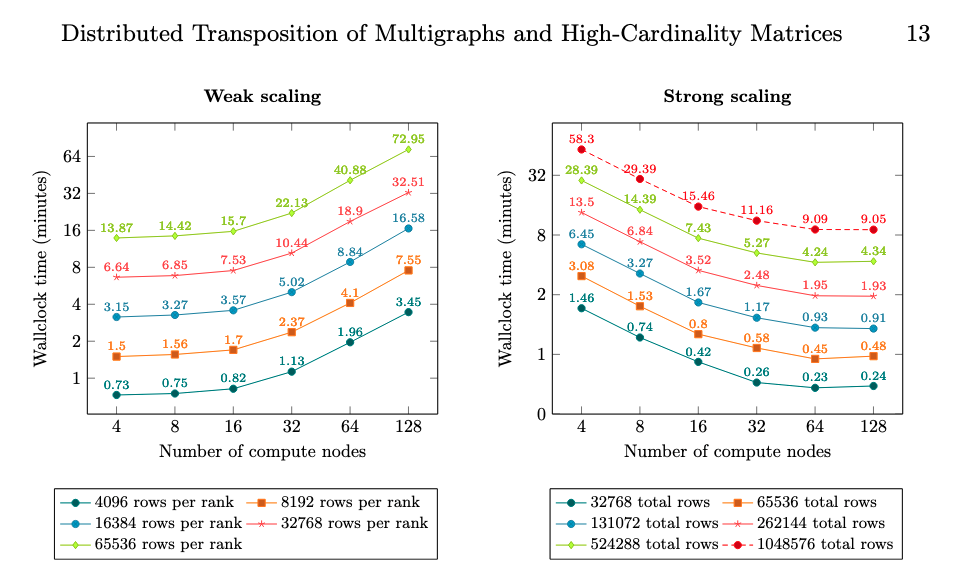
Magalhaes, B., & Schürmann, F. (2020). Efficient distributed transposition of large-scale multigraphs and high-cardinality sparse matrices. arXiv, 10 December2020.
DOI: http://arxiv.org/abs/2012.06012
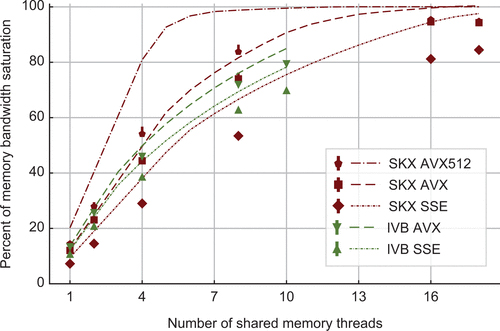
Ewart, T. Cremonesi, F. Delalondre, F. Schürmann, F. Polynomial evaluation on superscalar architecture, applied to the elementary function exp(x) Accepted with Transactions on Mathematical Software
DOI: https://doi.org/10.1145/3408893

Magalhaes, BRC. Hines, ML. Sterling, T. Schürmann, F. Fully-asynchronous fully-implicit variable-order variable-timestep simulation of neural networks In Krzhizhanovskaya, V. et al. (Eds.), ICCS 2020 Amsterdam: Lecture Notes in Computer Science, vol 12141. Springer, Cham.
https://arxiv.org/abs/1907.00670
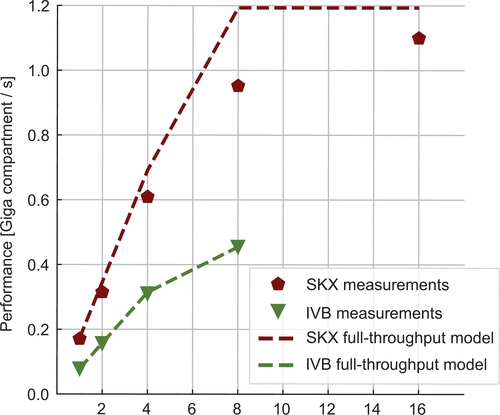
Cremonesi, F. Hager, G. Wellein, G. and Schürmann, F. Analytic Performance Modeling and Analysis of Detailed Neuron Simulations International Journal of High Performance Computing Applications. 2020. Volume: 34 issue: 4, page(s): 428-449.
DOI: https://doi.org/10.1177/1094342020912528
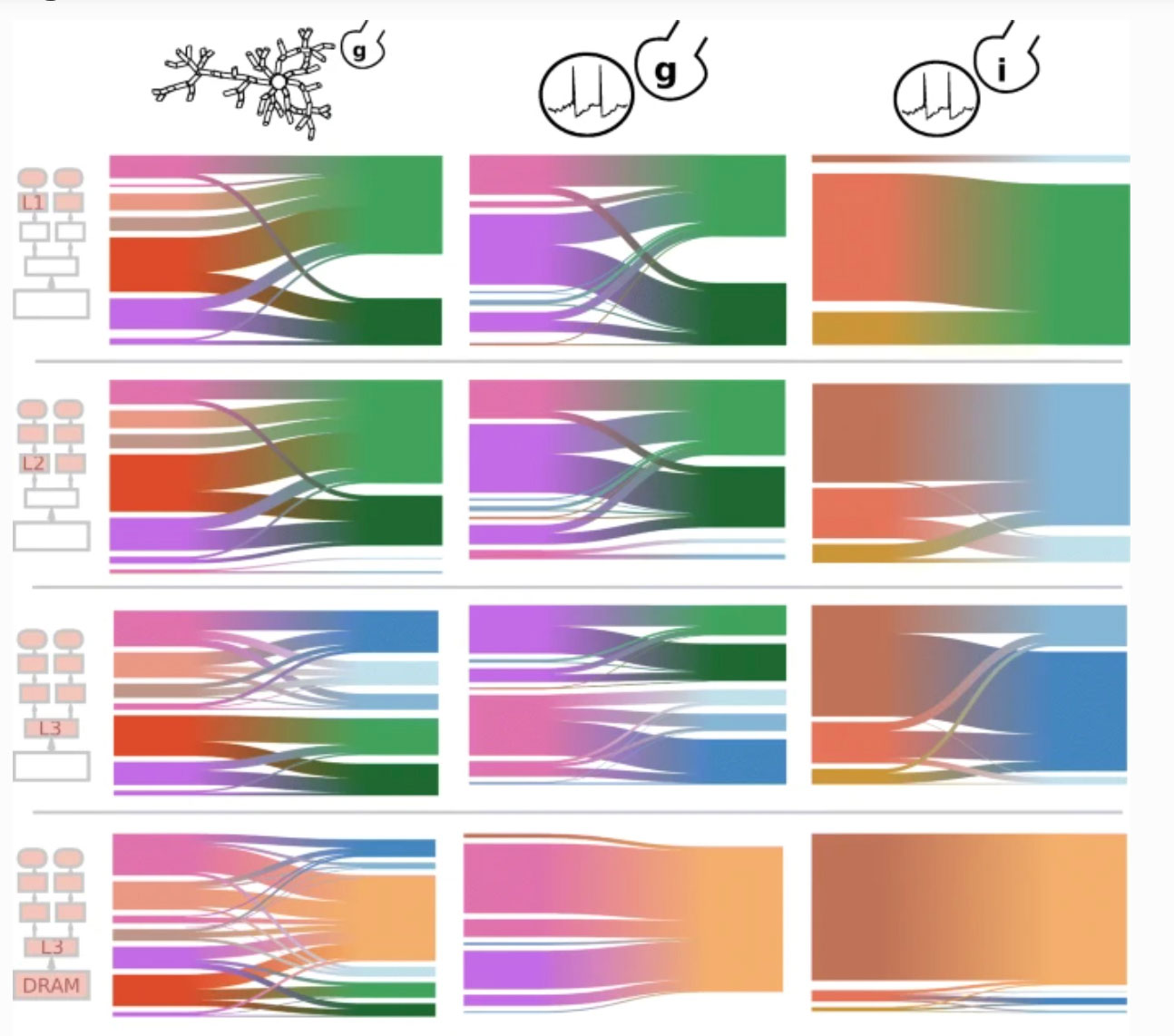
Cremonesi, F. and Schürmann, F. Understanding computational costs of cellular-level brain tissue simulations through analytical performance models. Neuroinform 18, pages 407–428 (2020).
DOI: https://doi.org/10.1007/s12021-019-09451-w
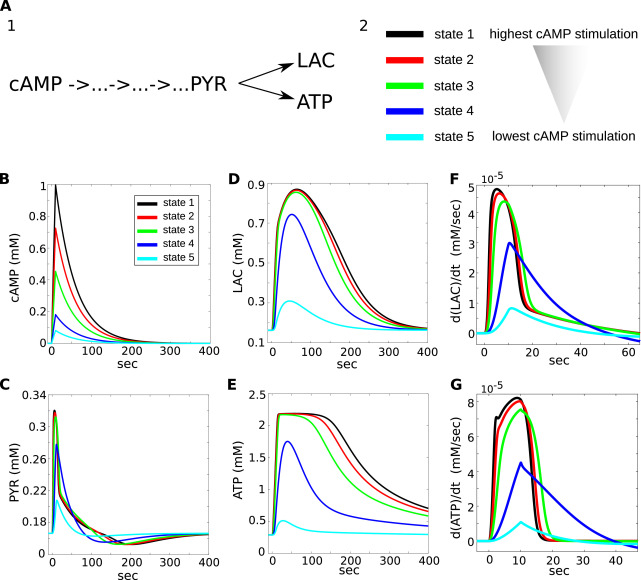
Coggan, JS. Keller, D. Markram, H. Schürmann, F. and Magistretti, PJ. Excitation states of metabolic networks predict dose-response fingerprinting and ligand pulse phase signalling. J Theor Biol. 2020 Feb 21;487:110123.
DOI: https://doi.org/10.1016/j.jtbi.2019.110123
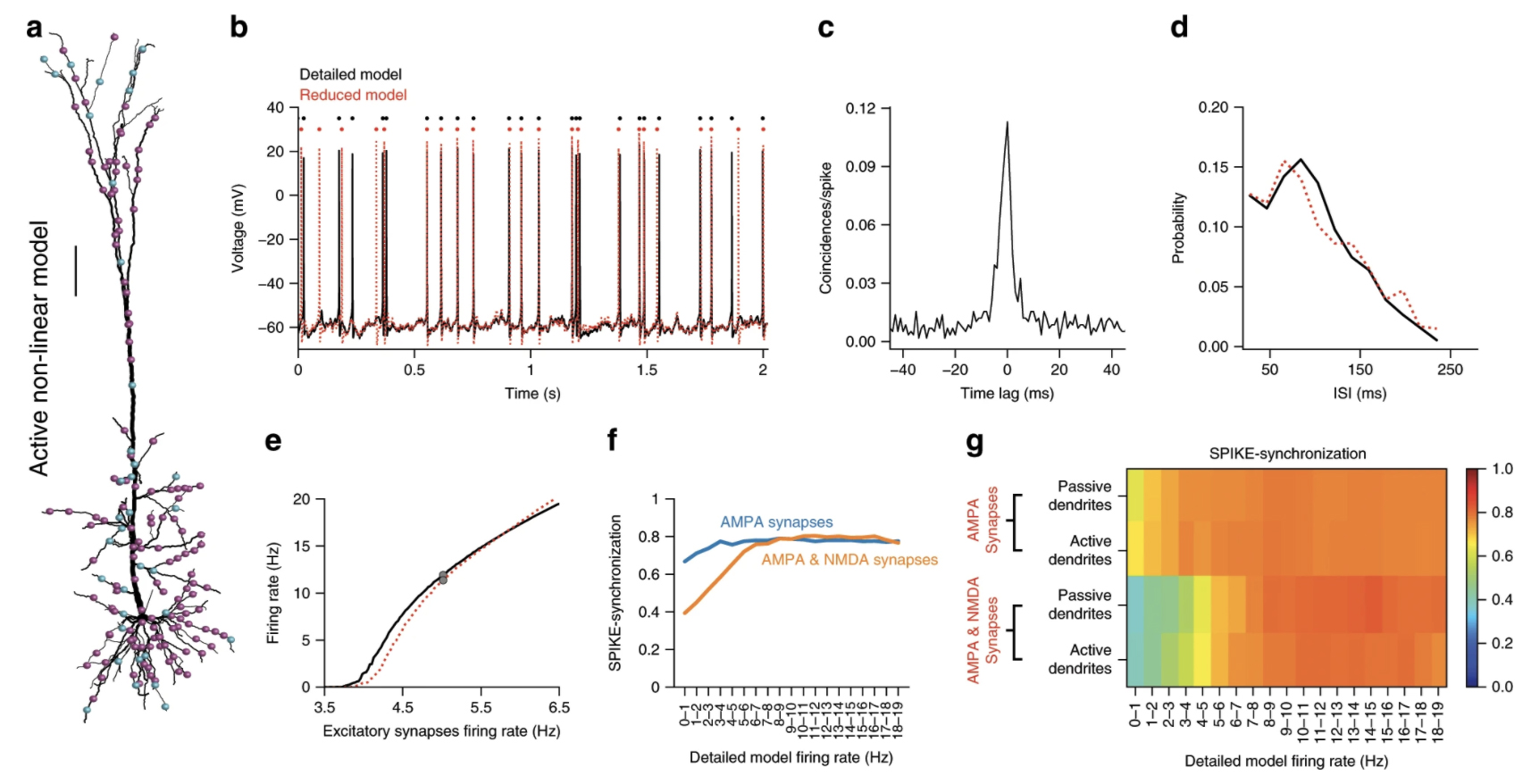
Amsalem, O. Eyal, G. Rogozinski, N. Gevaert, M. Kumbhar, P. Schürmann, F. Segev, I. An efficient analytical reduction of detailed nonlinear neuron models. Nat Commun 11, 288 (2020).
DOI: https://doi.org/10.1038/s41467-019-13932-6
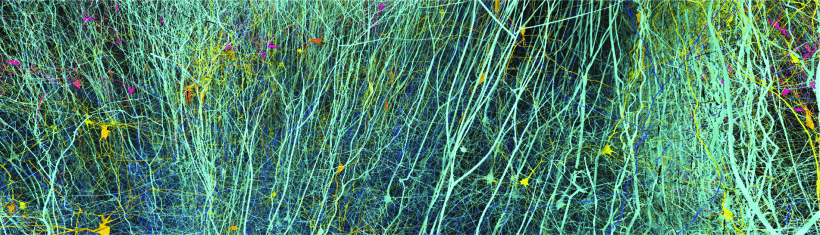
Ewart, T. Cremonesi, F. Delalondre, F. Schürmann, F. Polynomial evaluation on superscalar architecture, applied to the elementary function exp(x). Accepted with Transactions on Mathematical Software.
DOI: https://doi.org/10.1145/3408893
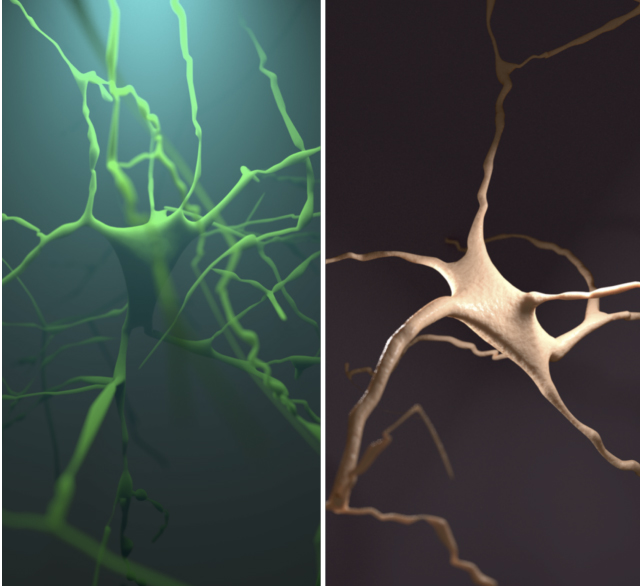
Abdellah, M. Favreau, C. Hernando, J. Lapere, S. Schürmann, F. Generating High Fidelity Surface Meshes of Neocortical Neurons using Skin Modifiers. In: Vidal FP et al. (eds) Computer Graphics and Visual Computing (CGVC). 2019. The Eurographics Association.
DOI: https://doi.org/10.2312/cgvc.20191257
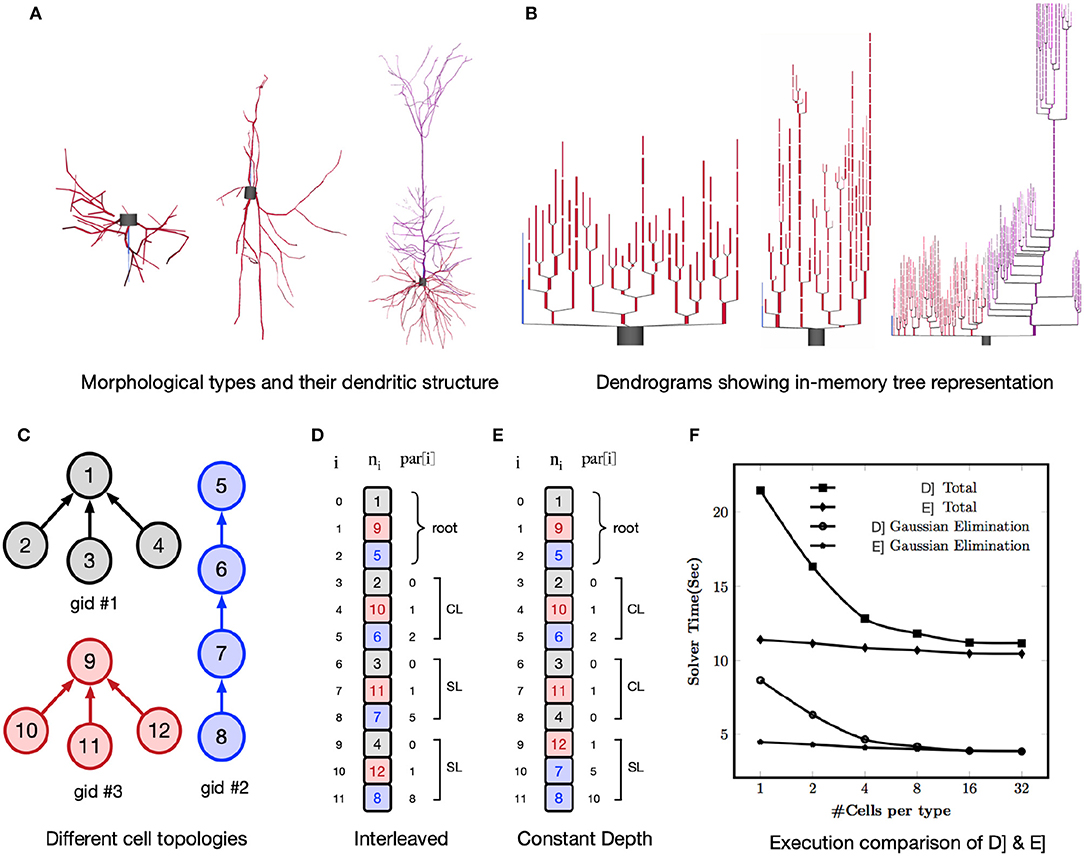
Kumbhar, PS. Delalondre, F. Hines, ML. Ovcharenkov, A. Schürmann, F. CoreNEURON – An Optimized Compute Engine for the NEURON Simulator. Front. Neuroinform., 2019;13:63.
DOI: https://doi.org/10.3389/fninf.2019.00063
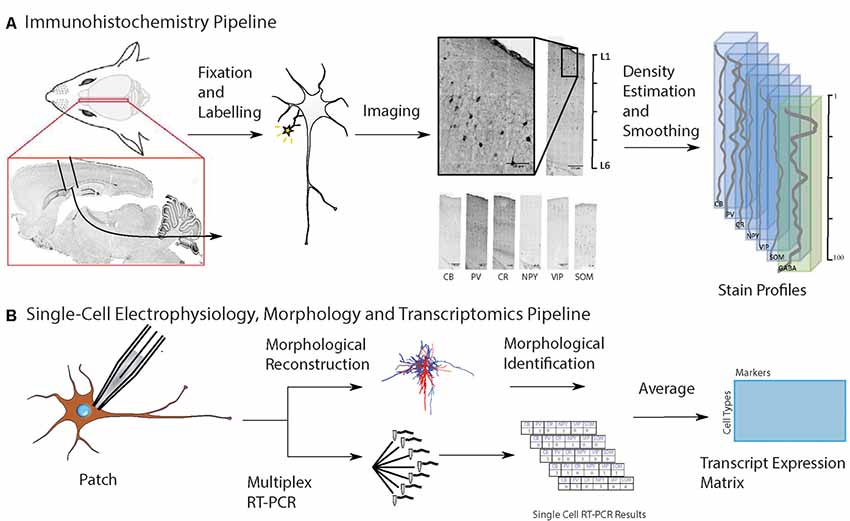
Keller, D. Meystre, J. Valiya Veettil, R. Burri, O. Guiet, R. Schürmann, F. Markram, H. A Derived Positional Mapping of Inhibitory Subtypes in the Somatosensory Cortex. Frontiers in Neuroanatomy. 2019;13:78.
DOI: https://doi.org/10.3389/fnana.2019.00078
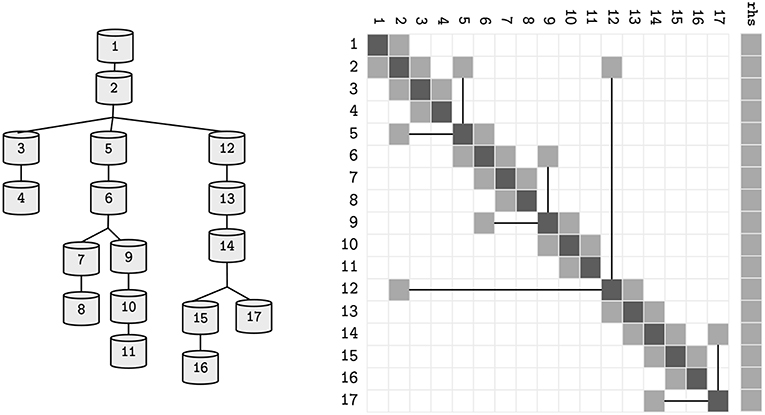
Magalhaes, BRC. Hines, ML. Sterling, T. Schürmann, F. Asynchronous SIMD-Enabled Branch-Parallelism of Morphologically-Detailed Neuron Models. Front. Neuroinform. 2019;13:54. Published 2019 Jul 23.
DOI: 10.3389/fninf.2019.00054
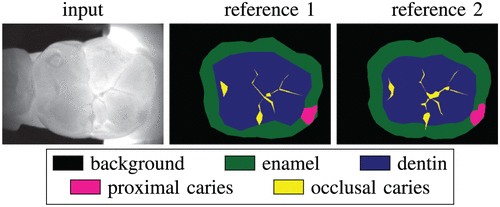
Casalegno, F. Newton, T. Daher, R. Abdelaziz, M. Lodi-Rizzini, A. Schürmann, F. Krejci, I. Markram, H. Caries Detection with Near-Infrared Transillumination Using Deep Learning. J Dent Res. 2019 Oct;98(11):1227-1233.
DOI: https://doi.org/10.1177/0022034519871884
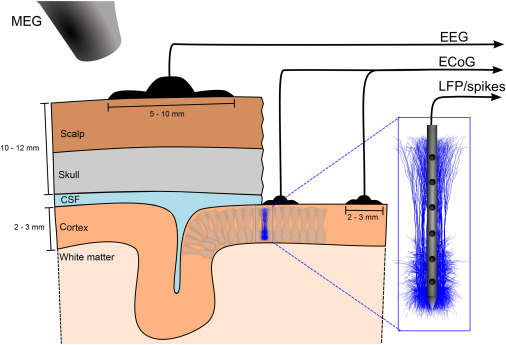
Einevoll, GT. Destexhe, A. Diesmann, M. Grün, S. Jirsa, V de Kamps, M. Migliore, M. Ness, TV. Plesser, HE. Schürmann, F. The scientific case for brain simulations. Neuron. 2019.
DOI: https://doi.org/10.1016/j.neuron.2019.03.027
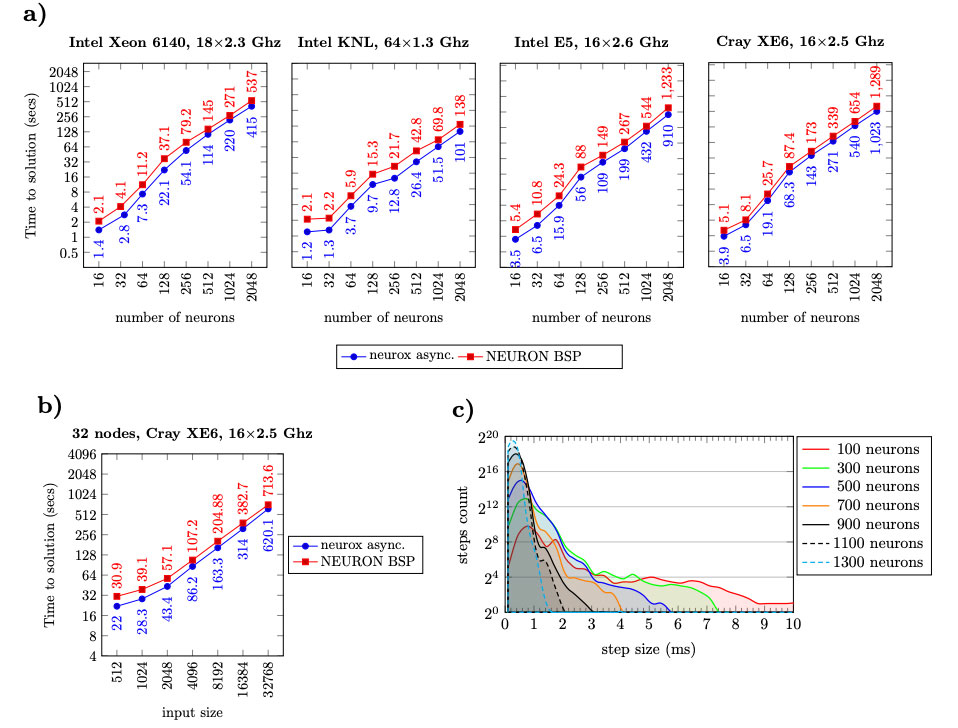
Magalhaes, BRC. Hines, ML. Sterling, T. Schürmann, F. Fully-Asynchronous Cache-Efficient Simulation of Detailed Neural Networks. International Conference on Computational Science (ICCS2019), Faro, Portugal, 12-14 June 2019
DOI; https://doi.org/10.1007/978-3-030-22744-9_33
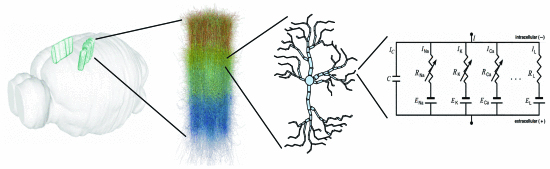
Magalhaes, BRC. Hines, ML. Sterling, T. Schürmann, F. Exploiting Flow Graph of System of ODEs to Accelerate the Simulation of Biologically-Detailed Neural Networks. International Parallel and Distributed Processing Symposium (IPDPS2019), Rio de Janeiro, Brazil, 20-24 May 2019
[leading Rank A conference for scientific computing with full papers and full peer review]
DOI: 10.1109/IPDPS.2019.00028

Planas, J. Delalondre, F. and Schürmann, F. Accelerating Data Analysis in Simulation Neuroscience with Big Data Technologies. In Computational Science – International Conference on Computational Science (ICCS 2018), Y. Shi, et al., eds. (Springer International Publishing), Lecture Notes in Computer Science book series (LNCS, volume 10860), 363–377.
[leading Rank A conference for scientific computing with full papers and peer review].
DOI: https://doi.org/10.1007/978-3-319-93698-7_28
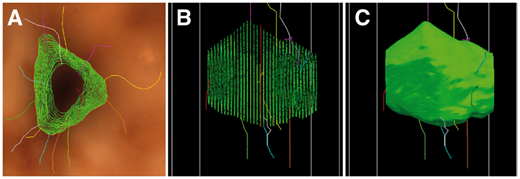
Abdellah, M. Hernando, J. Eilemann, S. Lapere, S. Antille, N. Markram, H. and Schürmann, F. NeuroMorphoVis: a collaborative framework for visualization and analysis of neuronal morphology skeletons reconstructed from microscopy stacks. Oxford Bioinformatics. 27 June 2018. doi.org/10.1093/bioinformatics/bty23.
DOI: https://doi.org/10.1093/bioinformatics/bty231
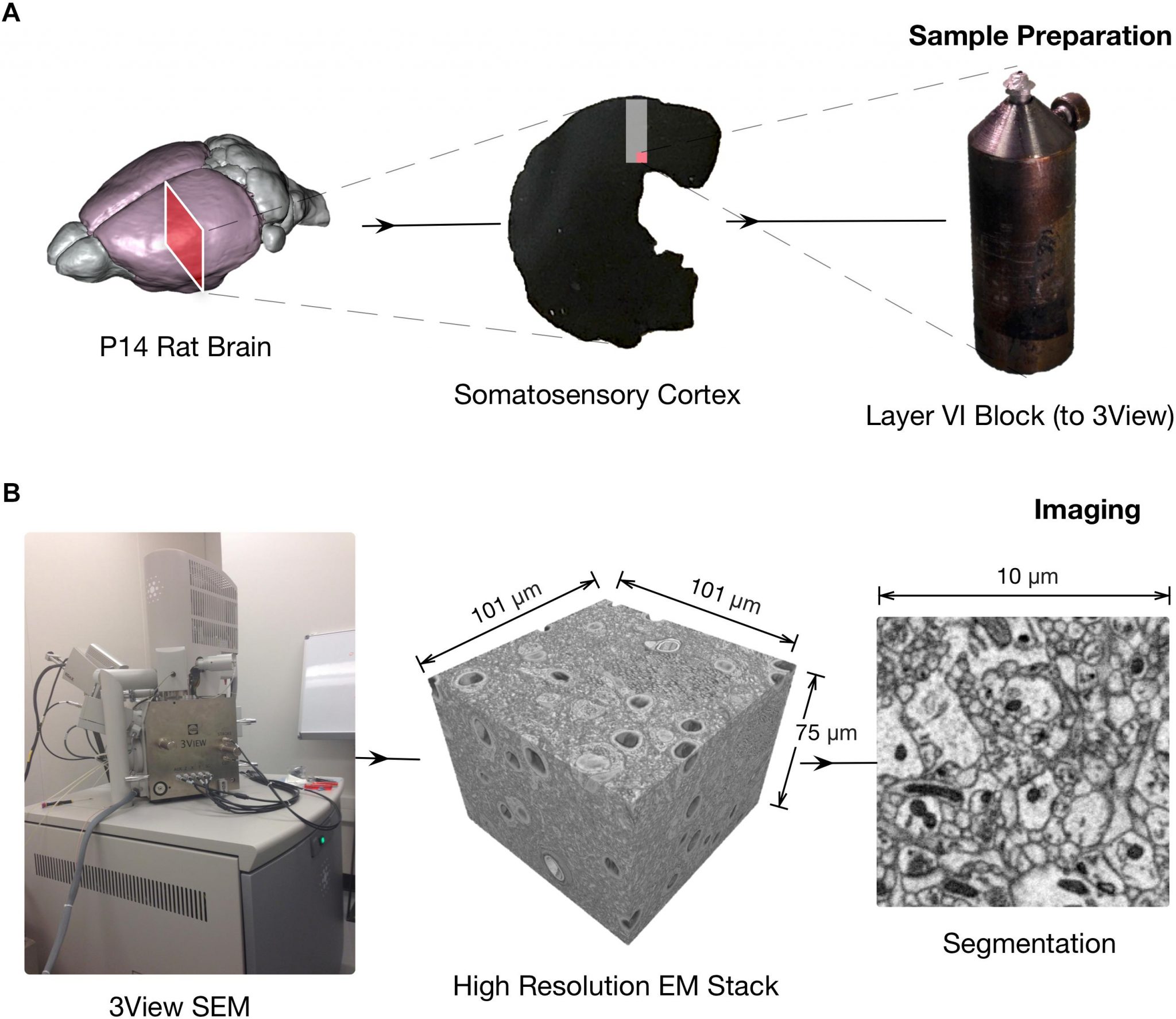
Coggan, JS. Calì, C. Keller, D. Agus, M. Boges, D. Abdellah, M. Kare, K. Lehväslaiho, H. Eilemann, S. Jolivet, RB. Hadwiger, M. Markram, H. Schürmann, F. Magistretti, PJ. A Process for Digitizing and Simulating Biologically Realistic Oligocellular Networks Demonstrated for the Neuro-Glio-Vascular Ensemble Frontiers in Neuroscience 12, 664.
DOI: 10.3389/fnins.2018.00664. 2018
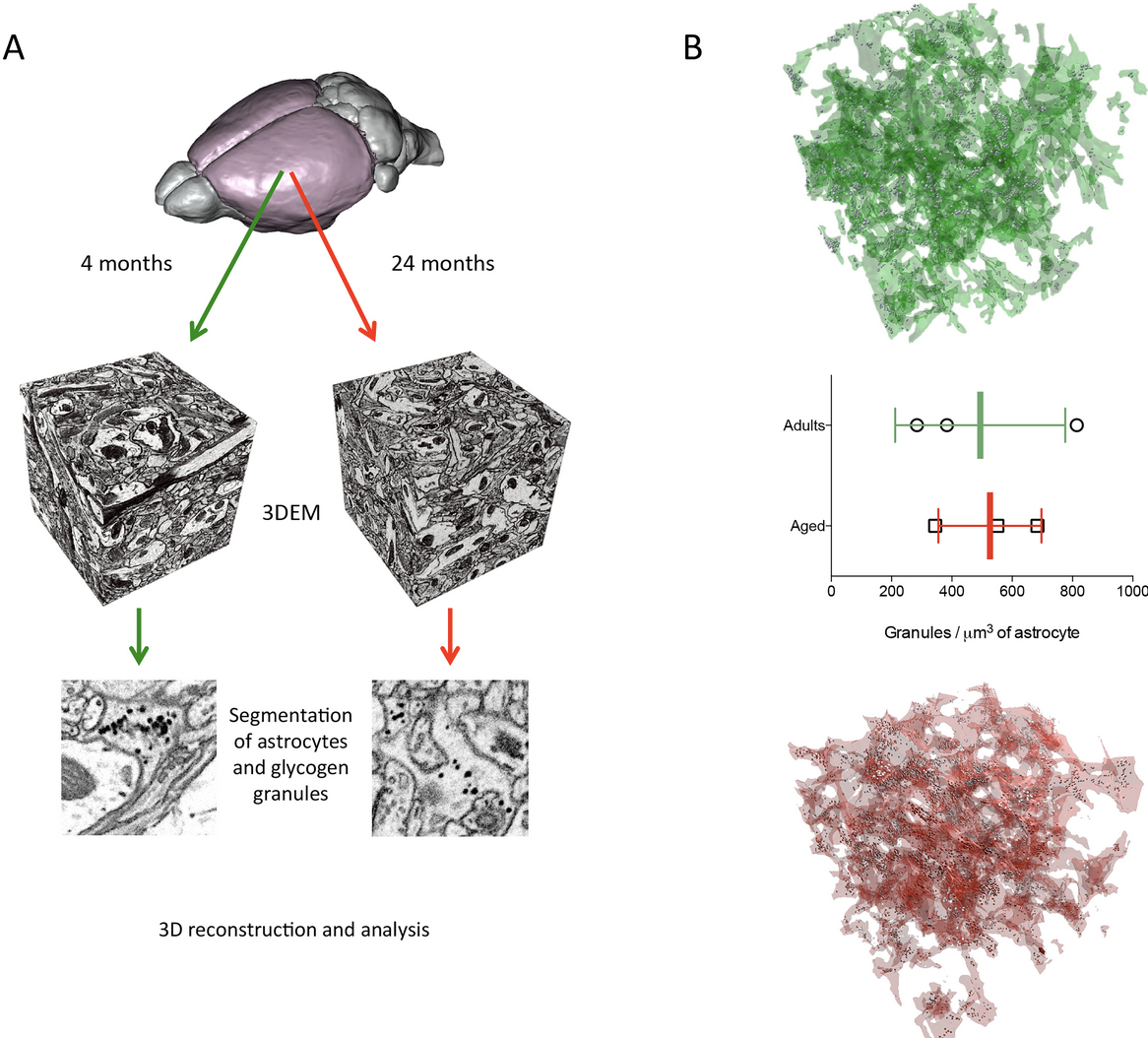
Coggan, JS. Keller, D. Calì, C. Lehväslaiho, H. Markram, H. Schürmann, F. and Magistretti, PJ. Norepinephrine stimulates glycogenolysis in astrocytes to fuel neurons with lactate PLOS Computational Biology 14(8).
DOI: 10.1371/journal.pcbi.1006392. 2018
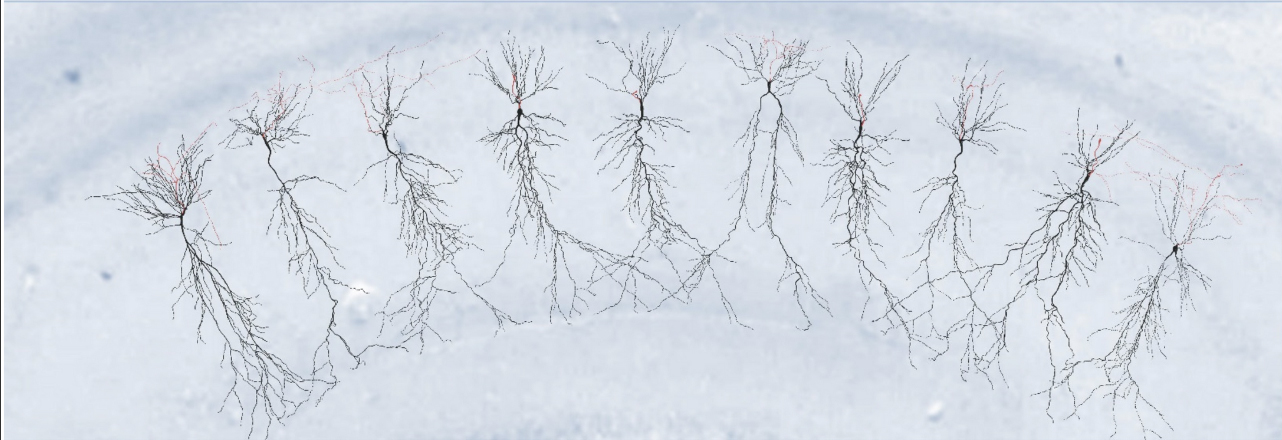
Migliore, R. Lupascu, CA, Bologna LL. Romani, A. Courcol, J-D. Antonel, S. Van Geit, WAH. Thomson, AM. Mercer, A. Lange, S. Falck, J. Roessert, CA. Freund, TF. Kali, S. Muller, EB. Schürmann, F. Markram, H. Migliore, M. The physiological variability of channel density in hippocampal CA1 pyramidal cells and interneurons explored using a unified data-driven modeling workflow PLOS Computational Biology 14(9).
DOI: 10.1371/journal.pcbi.1006423. 2018

Abdellah, M. Hernando, J. Antille, N. Eilemann, S. Markram, H. Schürmann, F. Reconstruction and visualization of large-scale volumetric models of neocortical circuits for physically-plausible in silico optical studies BMC Bioinformatics. 2017 18(Suppl 10):402.
DOI: 10.1186/s12859-017-1788-4
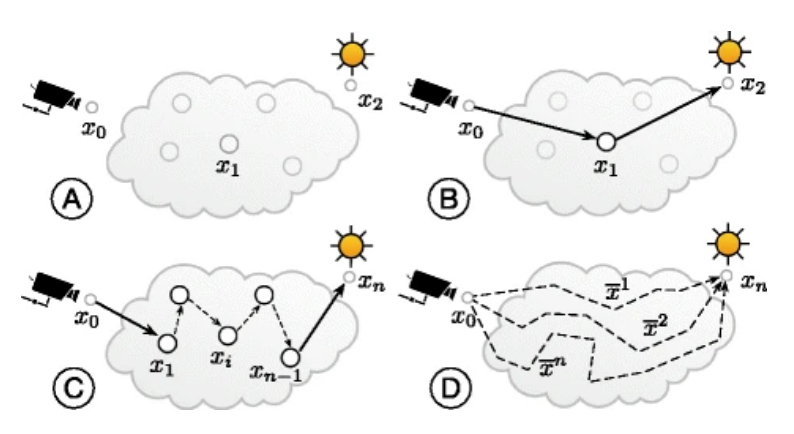
Abdellah, M. Bilgili, A. Eilemann, S. Shillcock, J. Markram, H. Schürmann, F. Bio-physically plausible visualization of highly scattering fluorescent neocortical models for in silico experimentation BMC Bioinformatics. 2017 Feb 15;18(Suppl 2):62.
DOI: 10.1186/s12859-016-1444-4.
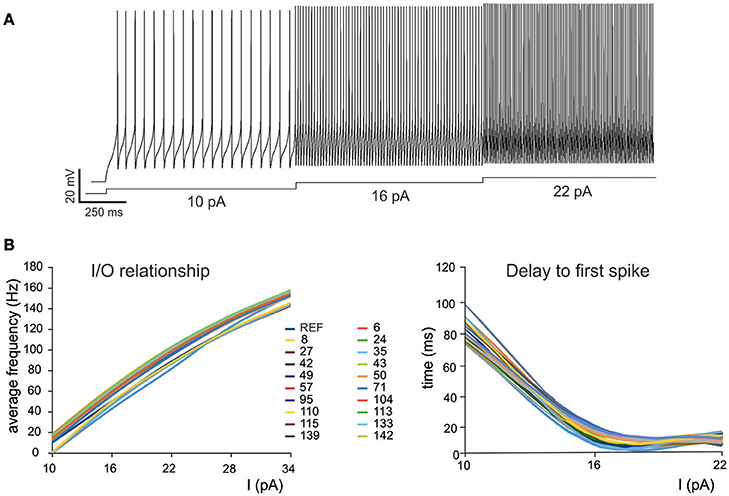
Masoli, S. Rizza, MF. Sgritta, M. van Geit, W. Schürmann, F. and D’Angelo, E. Single neuron optimization as a basis for accurate biophysical modelling: the case of cerebellar granule cells Front. Cell. Neurosci. 2017; 11:71.
DOI: 10.3389/fncel.2017.00071
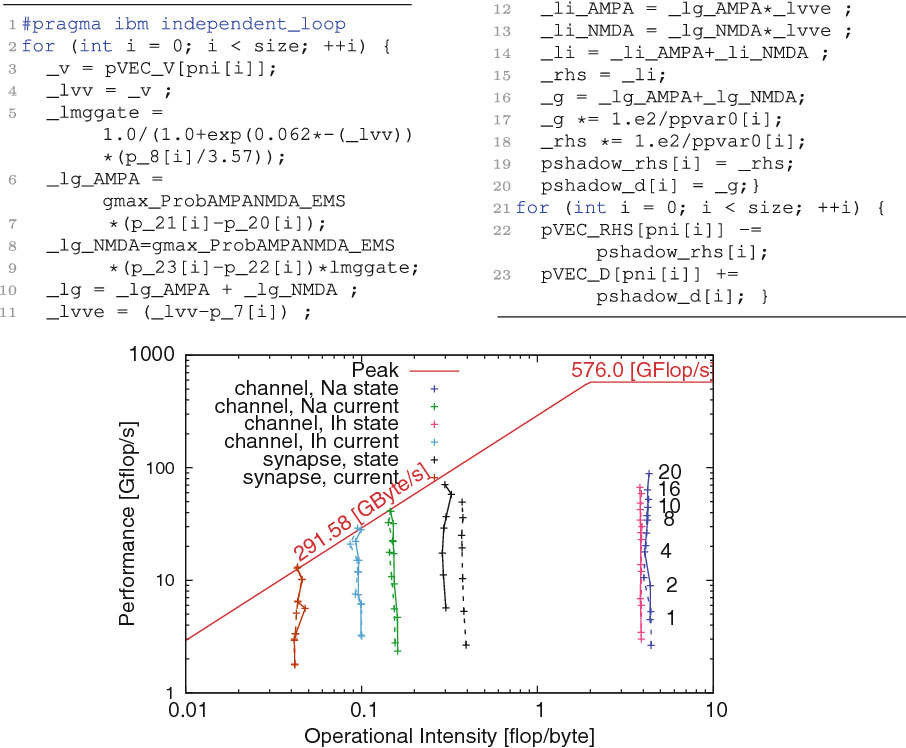
Ewart, T. Planas, J. Cremonesi, F. Langen, K. Schürmann, F. Delalondre, F. Neuromapp: A Mini-application Framework to Improve Neural Simulators In J.M. Kunkel et al. (eds.): ISC High Performance 2017, LNCS 10266, pp 181-198, 2017.
DOI: 10.1007/978-3-319-58667-0_10
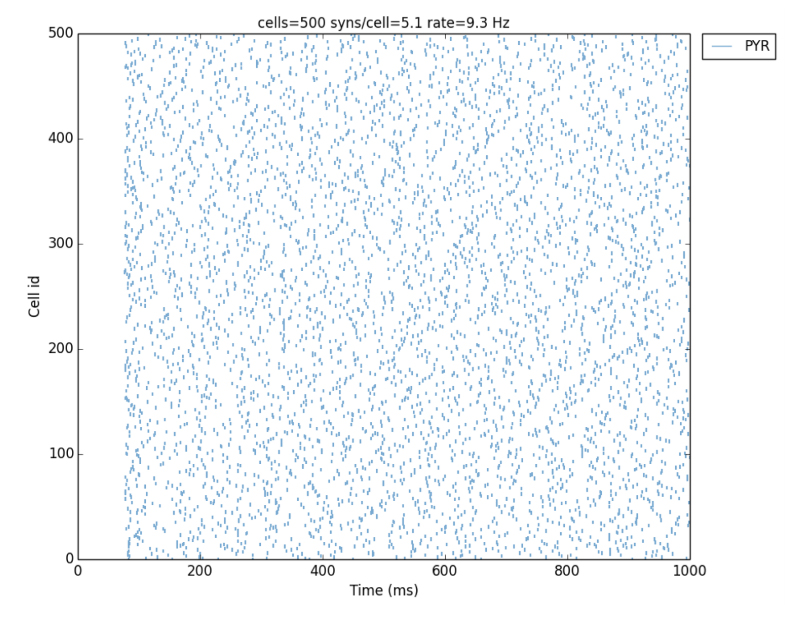
Lytton, WW. Seidenstein, AH. Dura-Bernal, S. McDougal, RA. Schürmann, F. and Hines, ML. Simulation neurotechnologies for advancing brain research: Parallelizing large networks in neuron Neural Comput. 2016 Oct;28(10):2063-90.
DOI: 10.1162/NECO_a_00876
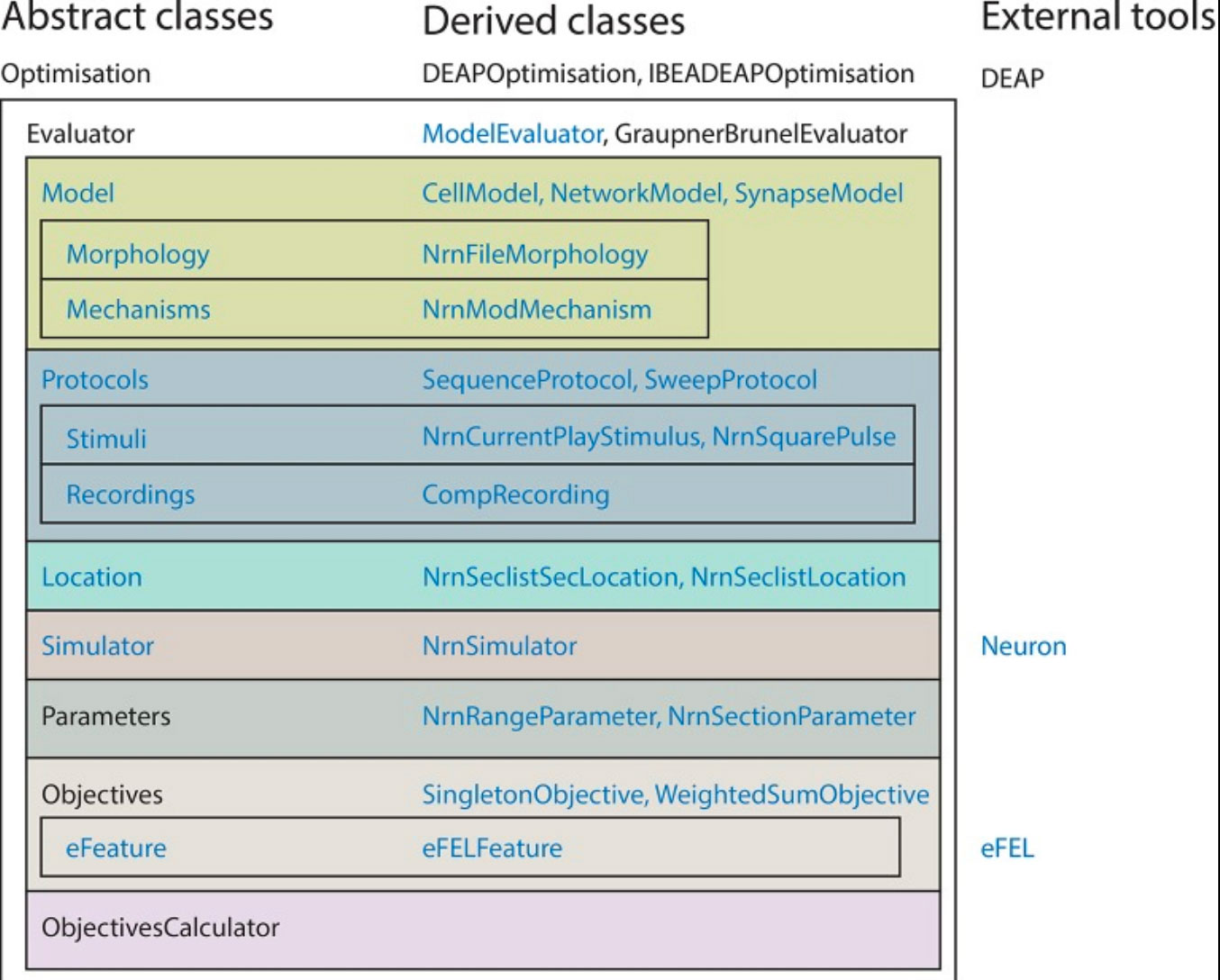
Van Geit, W. Gevaert, M. Chindemi, G. Rössert, C. Courcol, JD. Muller, EB. Schürmann, F. Segev, I. Markram, H. BluePyOpt: Leveraging Open Source Software and Cloud Infrastructure to Optimise Model Parameters in Neuroscience Front Neuroinform. 2016 Jun 7;10:17.
DOI: 10.3389/fninf.2016.00017. eCollection 2016
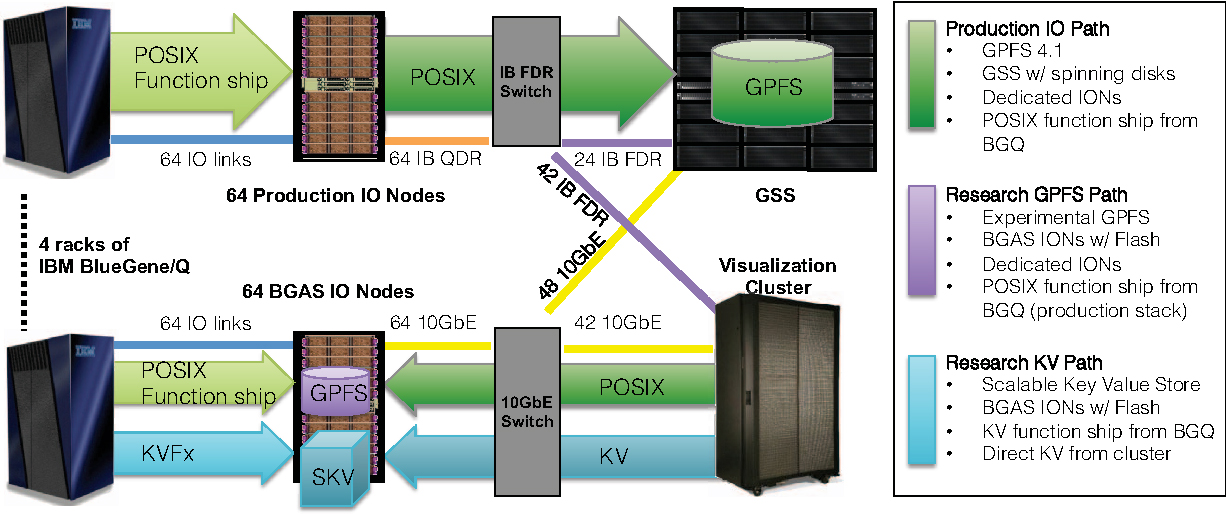
Eilemann, S. Delalondre, F. Bernard, J. Planas, J. Schürmann, F. Biddiscombe J. Bekas, C. Curioni, A. Metzler, B. Kaltstein, P. Morjan, P. Fenkes, J. Bellofatto, R. Schneidenbach, L. Ward ,TJC. Fitch, BG. Key-value Enabled Flash Memory Scientific Workflow with On-line Analysis and Visualization
In IEEE International Parallel and Distributed Processing Symposium (IPDPS); IEEE, 2016; pp 608–617.
DOI: 10.1109/IPDPS.2016.23
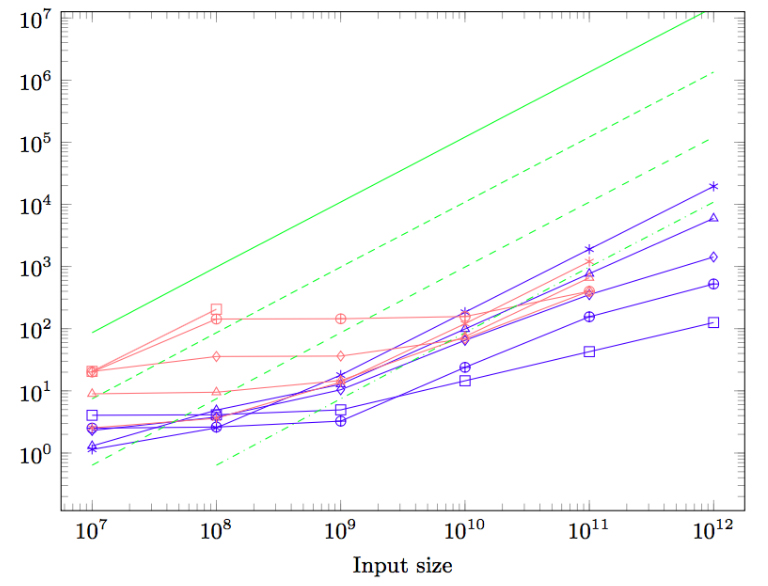
Magalhaes, B. Tauheed, F. Heinis, T. Ailamaki, A. Schürmann, F. An Efficient Parallel Load-balancing Framework for Orthogonal Decomposition of Geometrical Data In: Kunkel J., Balaji P., Dongarra J. (eds) High Performance Computing. ISC High Performance 2016. Lecture Notes in Computer Science, vol 9697, Pp. 81-97. Springer, Cham
DOI: 10.1007/978-3-319-41321-1_5
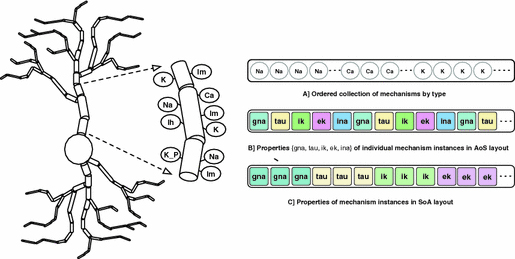
Kumbhar, P. Hines, ML. Ovcharenko, A. Mallon, DA. King, JG. Sainz, F. Schürmann, F. Delalondre, F. Leveraging a Cluster-Booster Architecture for Brain-Scale Simulations In: Kunkel J., Balaji P., Dongarra J. (eds) High Performance Computing. ISC High Performance 2016. Lecture Notes in Computer Science, vol 9697, Pp. 363–380. Springer, Cham
DOI: 10.1007/978-3-319-41321-1_19
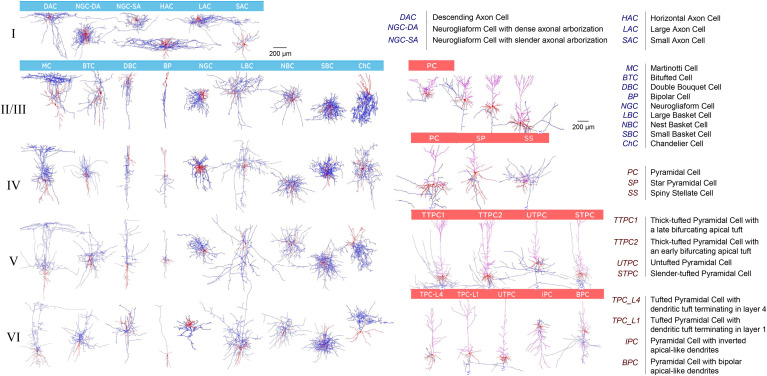
Markram, H. Muller, EB. Ramaswamy, S. Reimann, MW. Abdellah, M. Aguado Sanchez, C. Ailamaki, A. Alonso-Nanclares, L. Antille, N. Arsever, S. A. Antenekeng Kahou, GA. Berger, TK. Bilgili, A. Buncic, N. Chalimourda, A. Chindemi, G. Courcol, J-D. Delalondre, F. Delattre, V. Druckmann, S. Dumusc, R. Dynes, J. Eilemann, S. Gal, E. Gevaert, ME. Ghobril, J-P. Gidon, A. Graham, JW. Gupta, A. Haenel, V. Hay, E. Heinis, T. Hernando, JB. Hines, ML. Kanari, L. Keller, D. Kenyon, J. Khazen, G. Kim, Y. King, JG. Kisvarday, Z. Kumbhar, P. Lasserre, S. Le Bé, J-V. Magalhães, BRC. Merchán-Pérez, A. Meystre, J. Morrice, BR. Muller, J. Muñoz-Céspedes, A. Muralidhar, S. Muthurasa, K. Nachbaur, D. Newton, TH. Nolte, M. Ovcharenko, A. Palacios, J. Pastor, L. Perin, R. Ranjan, R. Riachi, I. Rodríguez, J-R. Riquelme, JL. Rössert, C. Sfyrakis, K. Shi, Y. Shillcock, JC. Silberberg, G. Silva, R. Tauheed, F. Telefont, M. Toledo-Rodriguez, M. Tränkler, T. Van Geit, W. Díaz, JV. Walker, R. Wang, Y. Zaninetta, SM. DeFelipe, J*. Hill, SL*. Segev, I*. and Schürmann, F. *Reconstruction and Simulation of Neocortical Microcircuitry
Cell 163, 2015, 456-492. [shared first author, *shared last author]
DOI: https://doi.org/10.1016/j.cell.2015.09.029
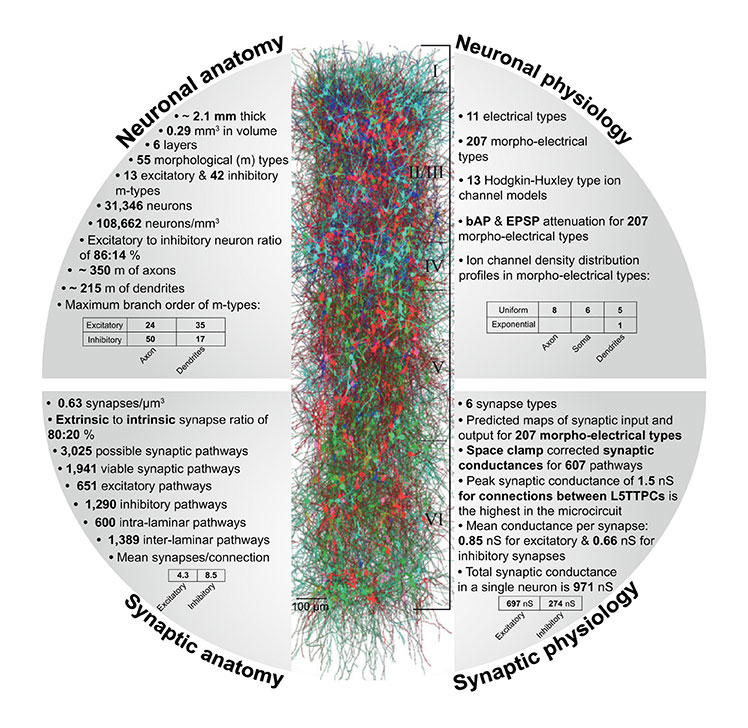
Ramaswamy, S. Courcol, J-D. Abdellah, M. Adaszewski, SR. Antille, N. Arsever, S. Atenekeng, G. Bilgili, A. Brukau, Y. Chalimourda, A. Chindemi, G. Delalondre, F. Dumusc, R. Eilemann, S. Gevaert, ME. Gleeson, P. Graham, JW. Hernando, JB. Kanari, L. Katkov, Y. Keller, D. King, JG, Ranjan, R. Reimann, MW. Rössert, C. Shi, Y. Shillcock, JC. Telefont, M. Van Geit, W. Villafranca Diaz, J. Walker, R. Wang, Y. Zaninetta, SM. DeFelipe, J. Hill, SL. Muller, J. Segev, I. Schürmann, F. Muller, EB. and Markram, H. The neocortical microcircuit collaboration portal: a resource for rat somatosensory cortex Front Neural Circuits 2015, 9, 44.
DOI: 10.3389/fncir.2015.00044

Abdellah, M. Bilgilli, A. Eilemann, S. Markram, H. Schürmann, F. Physically-based in silico light sheet microscopy for visualizing fluorescent brain models BMC Bioinformatics. 2015 Aug 13;16 Suppl 11:S8
DOI: 10.1186/1471-2105-16-S11-S8
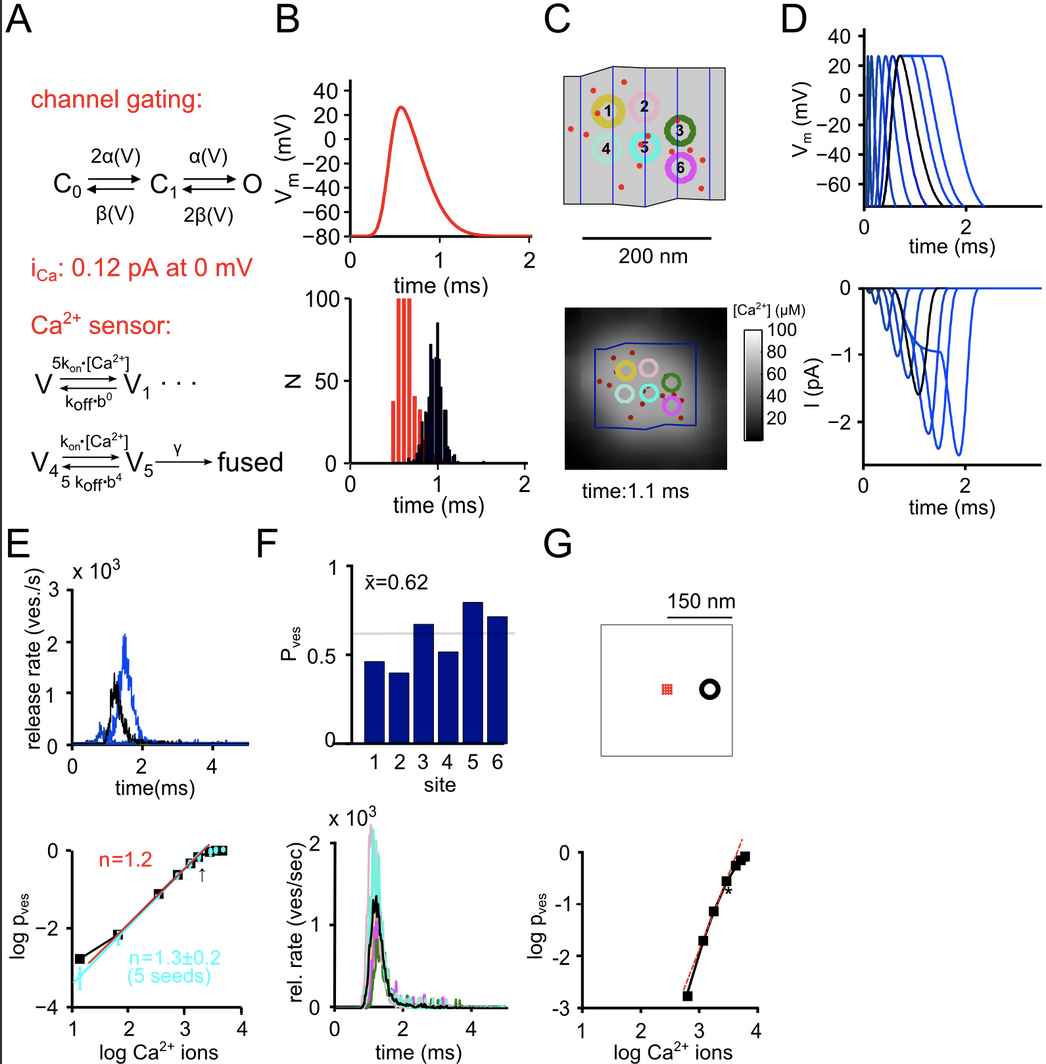
Keller, D. Babai, N. Kochubey, O. Han, Y. Markram, H. Schürmann, F. Schneggenburger, R. An Exclusion Zone for Ca2+ Channels around Docked Vesicles Explains Release Control by Multiple Channels at a CNS Synapse PLoS Comput Biol. 2015 May 7;11(5):e1004253.
DOI: 10.1371/journal.pcbi.1004253
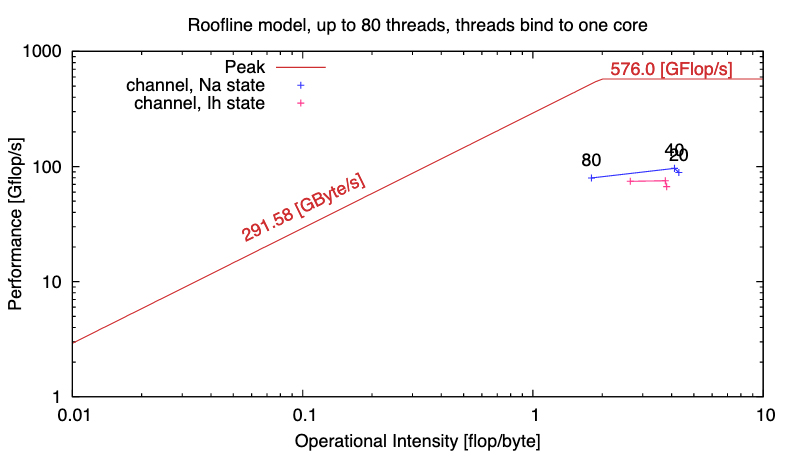
Ewart, T. Yates, S. Cremonesi, F. Kumbhar, P. Schürmann, F. Delalondre, F. Performance Evaluation of the IBM POWER8 system to Support Computational Neuroscientific Application Using Morphologically Detailed Neurons In: Proceedings of the 6th International Workshop on Performance Modeling, Benchmarking, and Simulation of High Performance Computing Systems. PMBS ’15. New York, NY, USA: ACM; 2015:1:1–1:11.
DOI: 10.1145/2832087.2832088
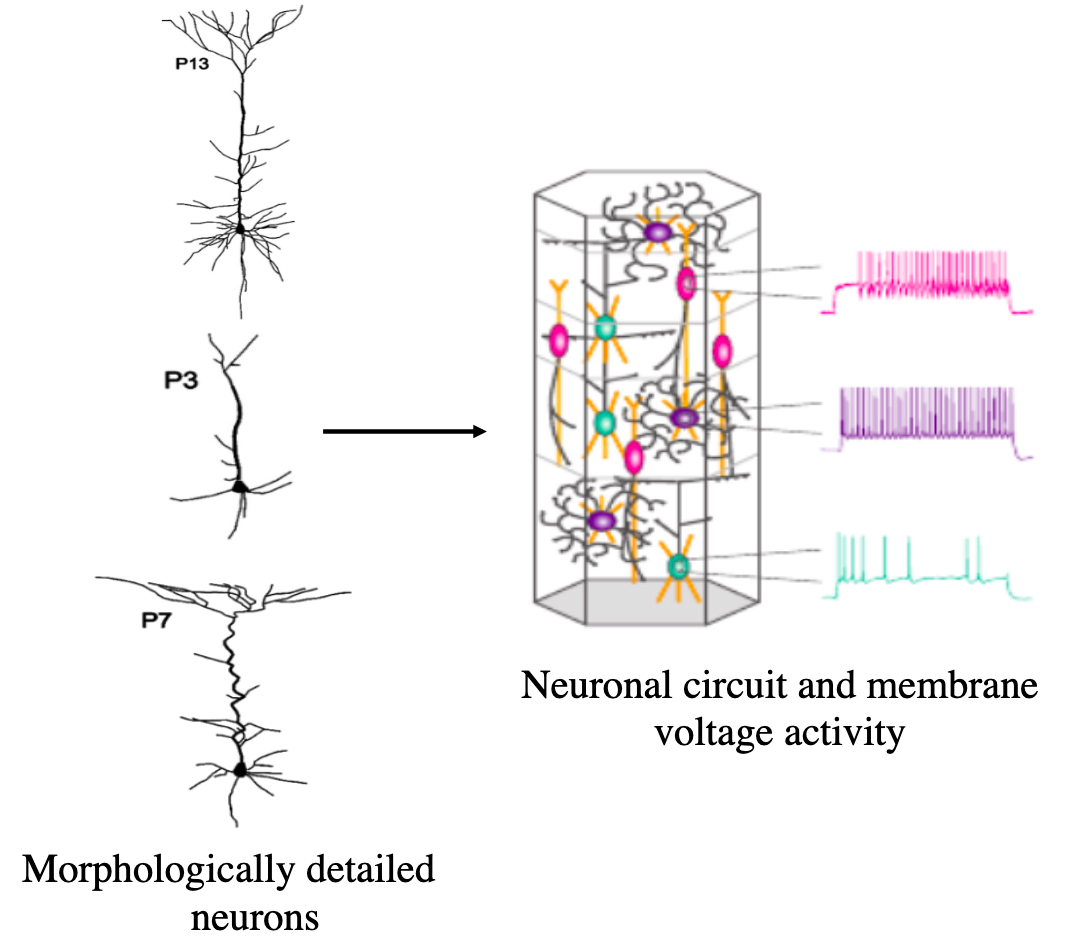
Ovcharenko, A. Kumbhar, P. Hines, M. Cremonesi, F. Ewart, T. Yates, S. Schürmann, F. Delalondre, F. Simulating Morphologically Detailed Neuronal Networks at Extreme Scale 2015 ParCo Conference, in “Parallel Computing: On the Road to Exascale” by IOS press,
ISBN print 978-1-61499-620-0, ISBN online 978-1-61499-621-7
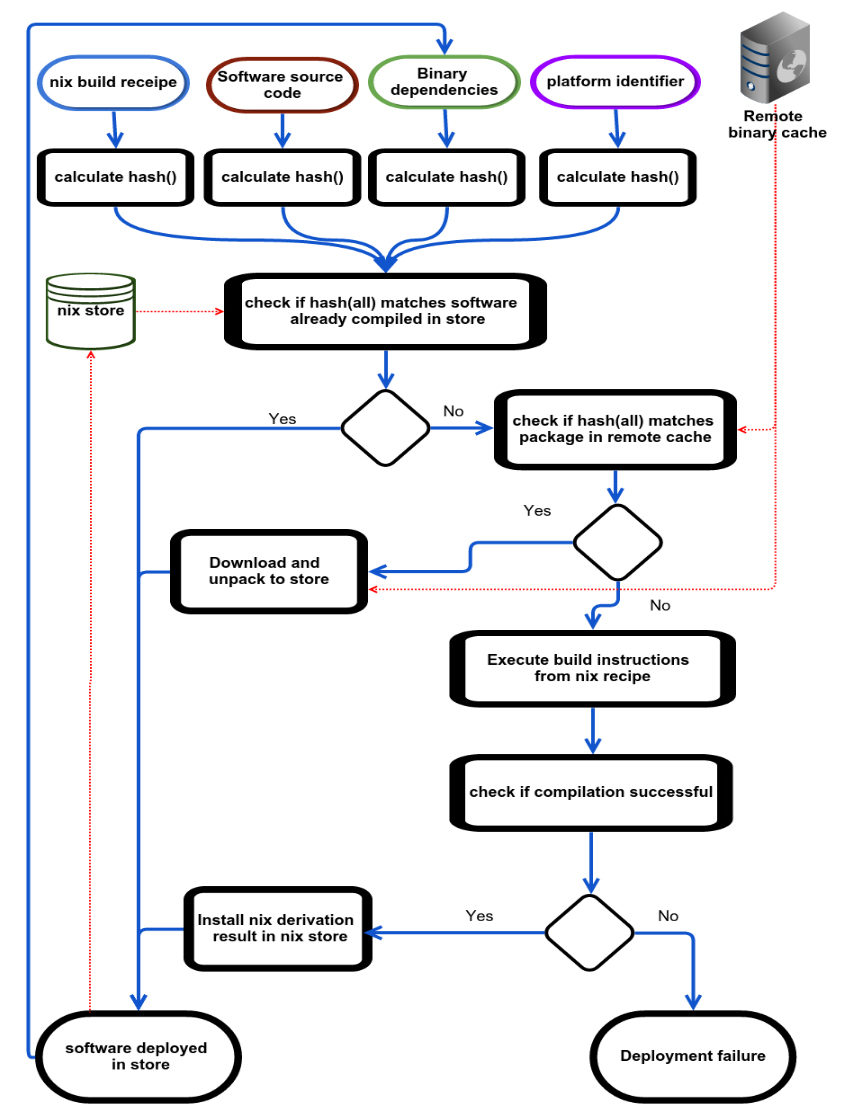
Devresse, A. Delalondre, F. Schürmann, F. Nix Based Fully Automated Workflows and Ecosystem to guarantee Scientific Result Reproducibility across Platforms, Software Environment and Systems In: Proceedings of the 3rd International Workshop on Software Engineering for High Performance Computing in Computational Science and Engineering. SE-HPCCSE ’15. New York, NY, USA: ACM; 2015:25–31.
DOI: 10.1145/2830168.2830172
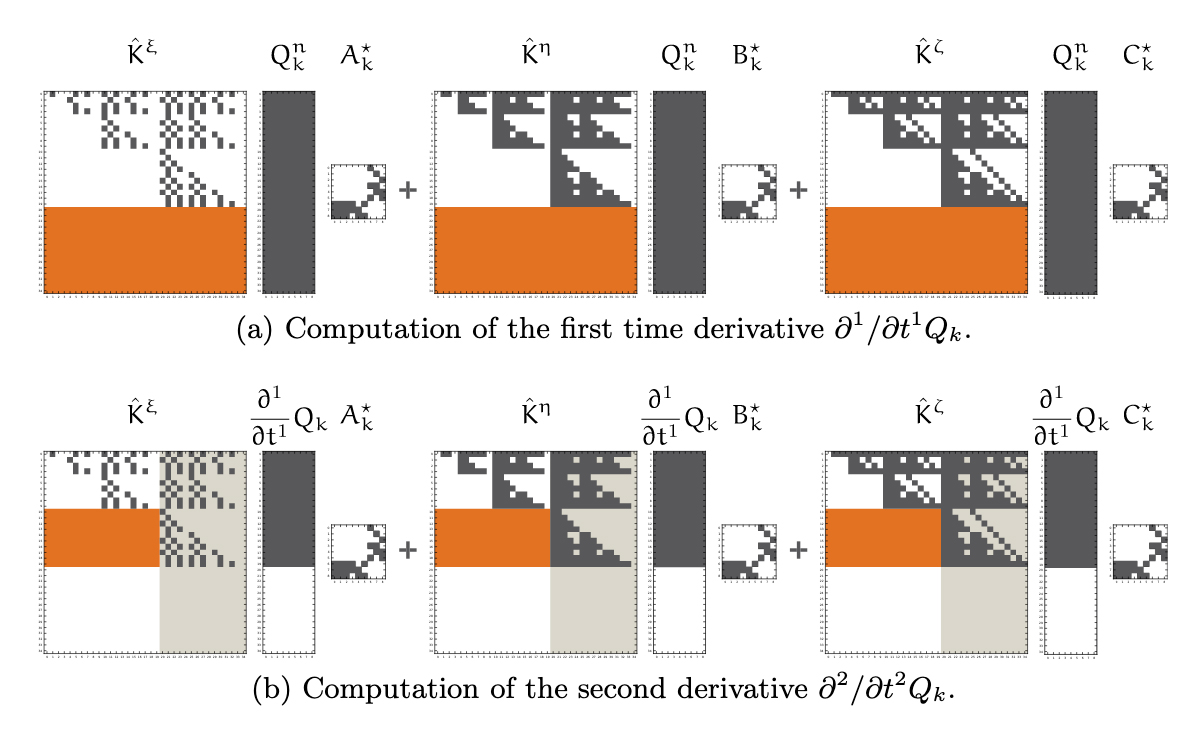
Schürmann, F. Delalondre, F. Kumbhar, PS. Biddiscombe, J. Gila, M. Tacchella, D. Curioni, A. Metzler, B. Morjan, P. Fenkes, J. Franceschini, MM. Germain, RS. Schneidenbach, L. Ward, TJC. Fitch, BG. Rebasing I/O for Scientific Computing: Leveraging Storage Class Memory in an IBM BlueGene/Q Supercomputer In: Kunkel JM, Ludwig T, Meuer HW, eds. Supercomputing. Springer International Publishing; 2014:331-347.
DOI: 10.1007/978-3-319-07518-1_21
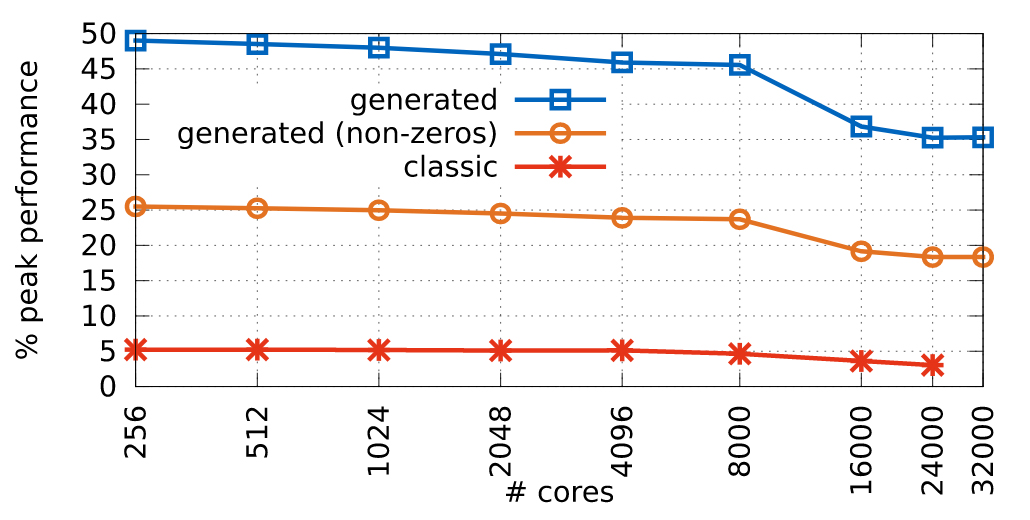
Ewart, T. Delalondre, F. Schürmann, F. Cyme: A Library Maximizing SIMD Computation on User-Defined Containers In: Kunkel JM, Ludwig T, Meuer HW, eds. Supercomputing. Springer International Publishing; 2014:440-449.
DOI: 10.1007/978-3-319-07518-1_29
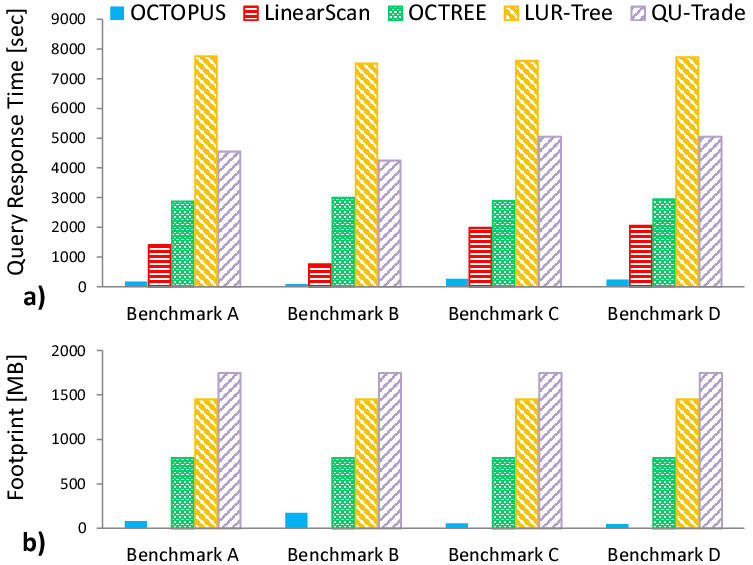
Tauheed, F. Heinis, T. Schürmann, F. Markram, H. Ailamaki, A. OCTOPUS: Efficient Query Execution on Dynamic Mesh Datasets
In IEEE 30th International Conference on Data Engineering; 2014; pp 1000–1011.
DOI: 10.1109/ICDE.2014.6816718
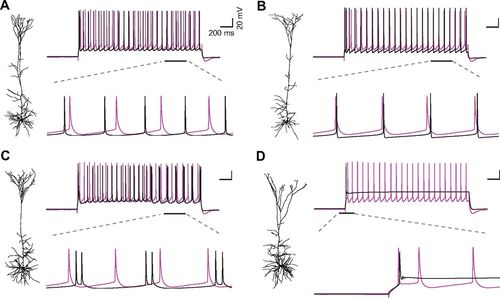
Hay, E. Schürmann, F. Markram, H. Segev, I. Preserving axosomatic spiking features despite diverse dendritic morphology
J Neurophysiol., 2013 Jun;109(12):2972-81. Epub 2013 Mar 27
DOI: 10.1152/jn.00048.2013
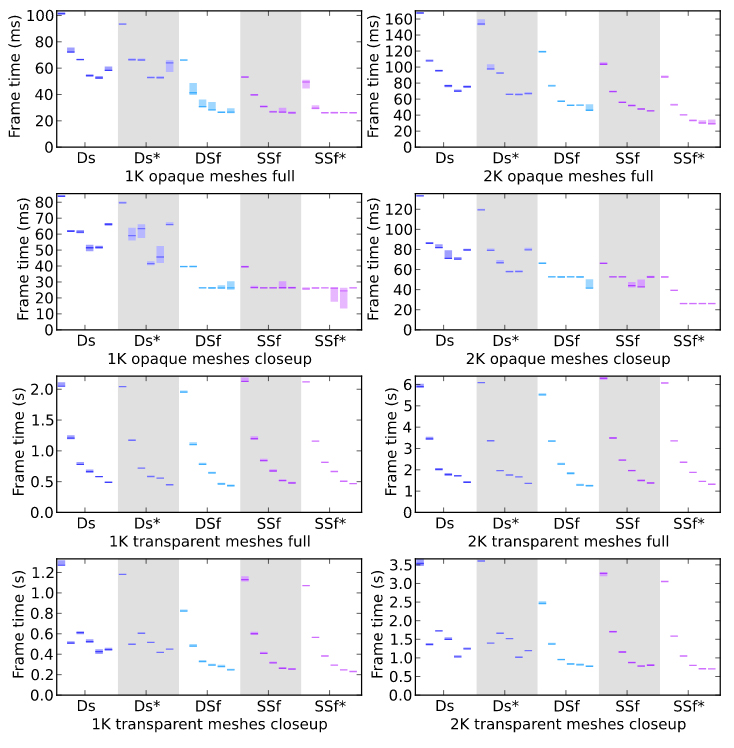
Hernando, JB. Biddiscombe, J. Bohara, B. Eilemann, S. Schürmann, F. Practical parallel rendering of detailed neuron simulations
In Proceedings of the 13th Eurographics Symposium on Parallel Graphics and Visualization; EGPGV ’13; Eurographics Association: Aire-la-Ville, Switzerland, Switzerland, 2013; pp 49–56.
DOI: 10.2312/EGPGV/EGPGV13/049-056
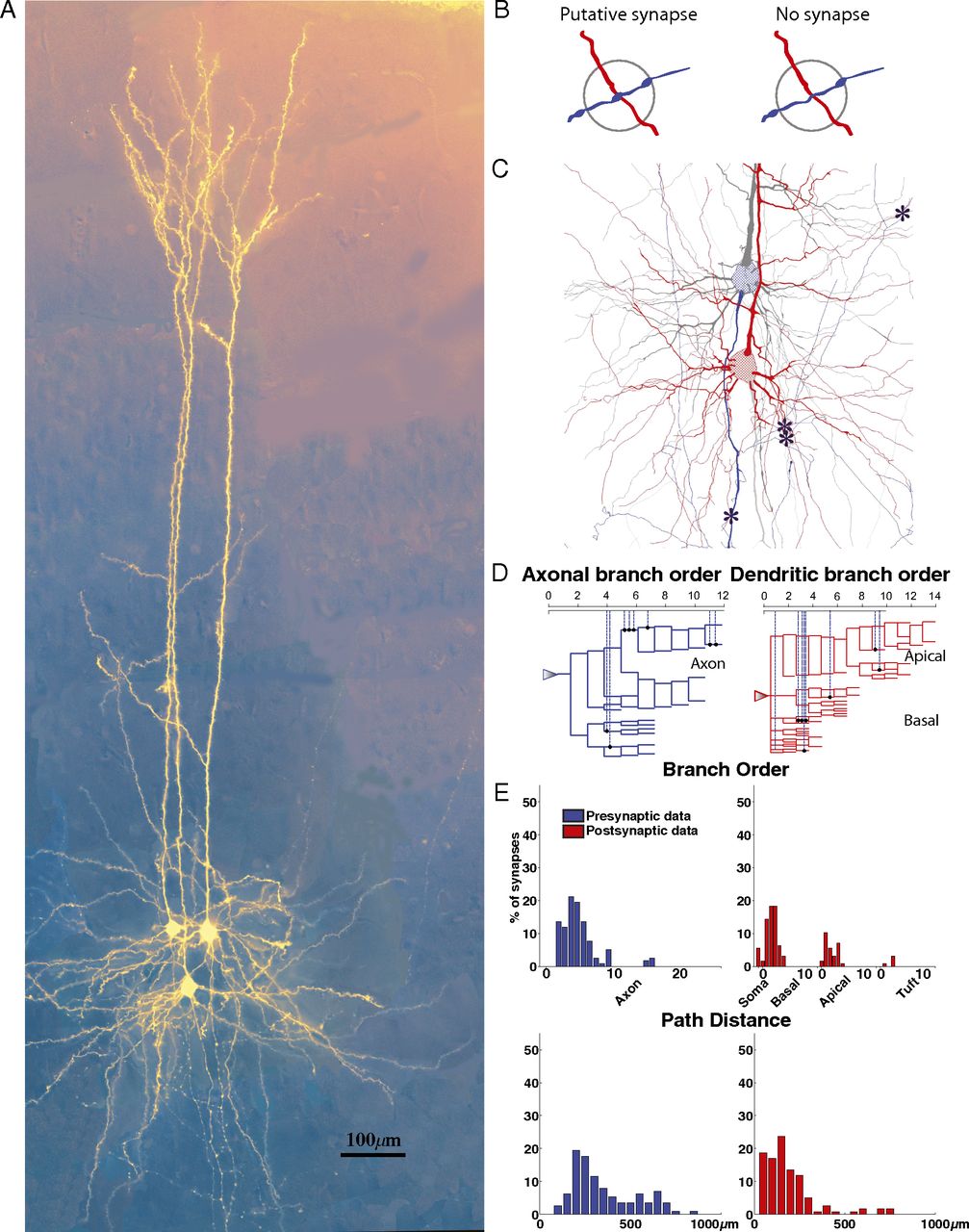
Hill, SL. Wang, Y. Riachi, I. Schürmann, F. Markram, H. Statistical connectivity provides a sufficient foundation for specific functional connectivity in neocortical neural microcircuits PNAS, 2012 Oct 16;109(42):E2885-94.
DOI: 10.1073/pnas.1202128109. Epub 2012 Sep 18
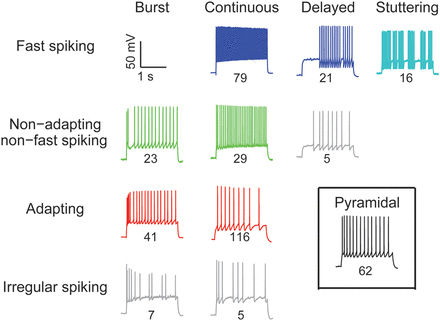
Druckmann, S. Hill, SL. Schürmann, F. Markram, H. Segev, I. A Hierarchical Structure of Cortical Interneuron Electrical Diversity Revealed by Automated Statistical Analysis Cereb. Cortex 2013, 23 (12), 2994–3006.
DOI: 10.1093/cercor/bhs290
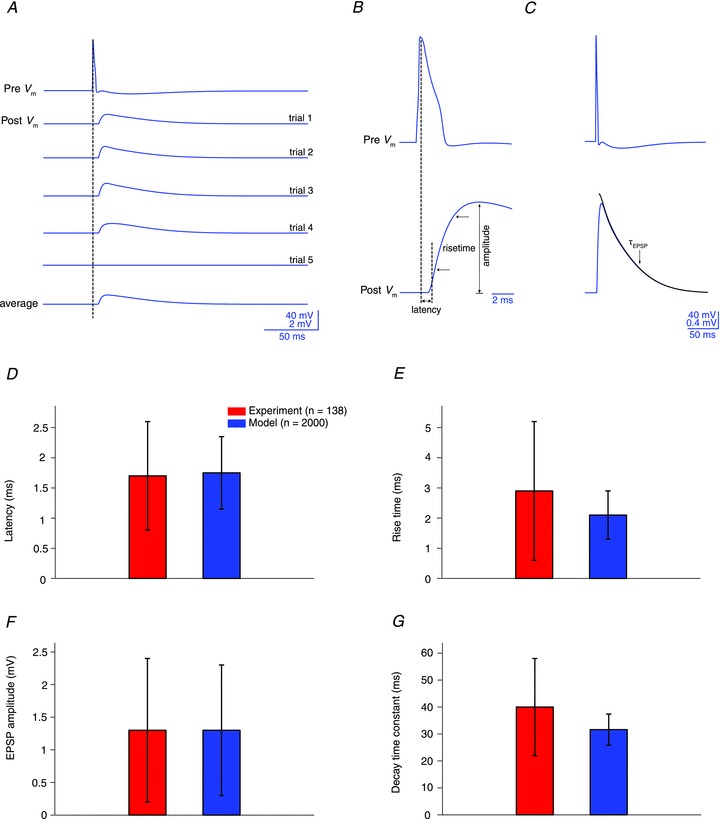
Ramaswamy, S. Hill, SL. King, JG. Schürmann, F. Wang, Y. and Markram, H. Intrinsic Morphological Diversity of Thick-tufted Layer 5 Pyramidal Neurons Ensures Robust and Invariant Properties of in silico Synaptic Connections J Physiol. 2012 Feb 15;590(Pt 4):737-52. Epub 2011 Nov 14
DOI: 10.1113/jphysiol.2011.219576. Epub 2011 Nov 14
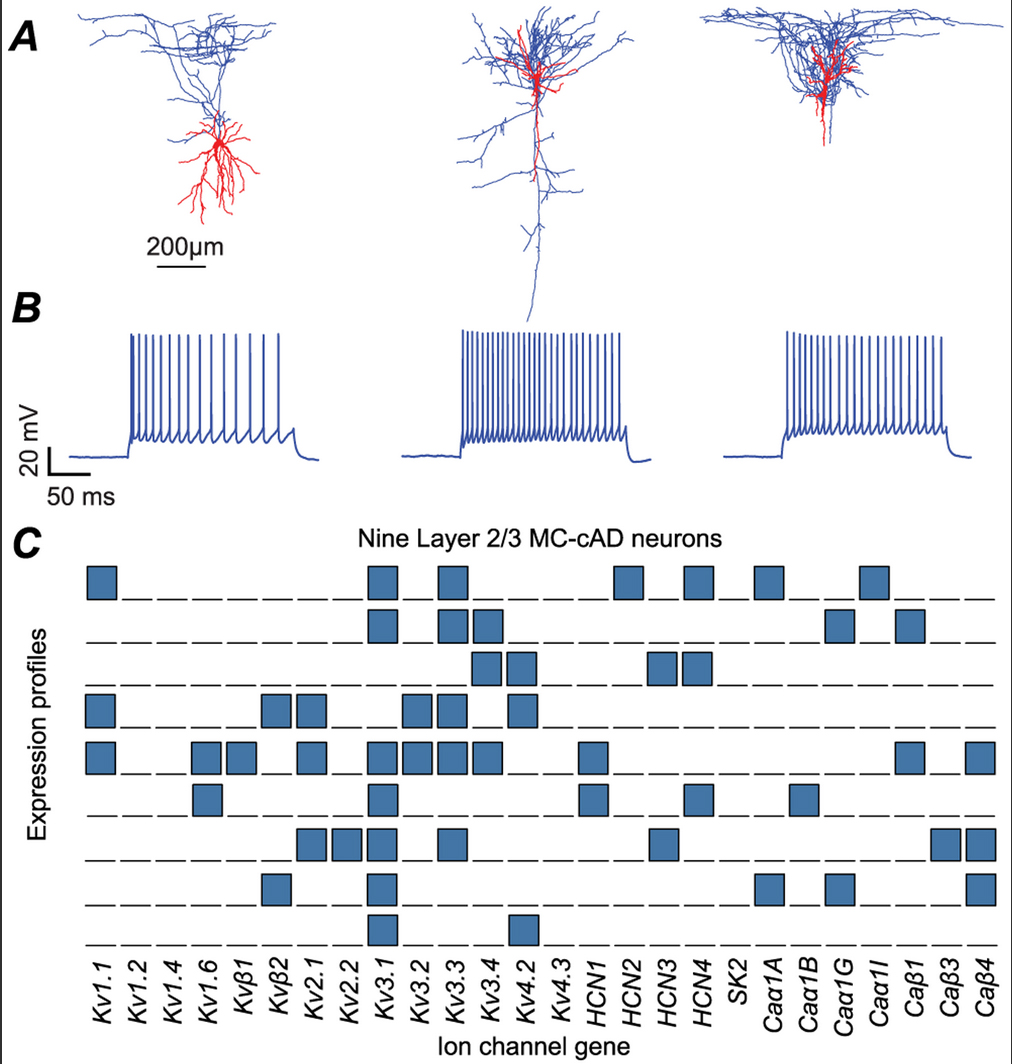
Khazen, G. Hill, SL. Schürmann, F. Markram, H. Combinatorial Expression Rules of Ion Channel Genes in Juvenile Rat (Rattus norvegicus) Neocortical Neurons. PLoS ONE 2012, 7 (4), e34786.
DOI: 10.1371/journal.pone.0034786
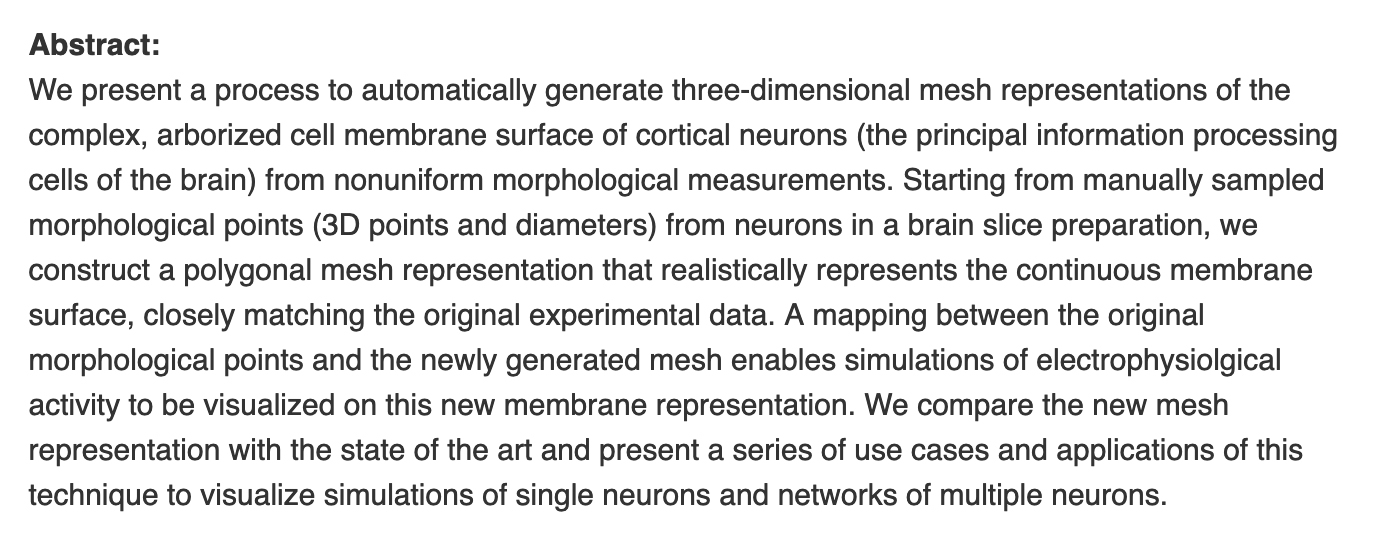
Lasserre, S. Hernando, J. Hill, SL. Schürmann, F. Anasagasti, PM. Abou-Jaoudé, G. Markram, H. A Neuron Membrane Mesh Representation for Visualization of Electrophysiological Simulations IEEE Trans Vis Comput Graph 2012, 18 (2), 214–227.
DOI: 10.1109/TVCG.2011.55
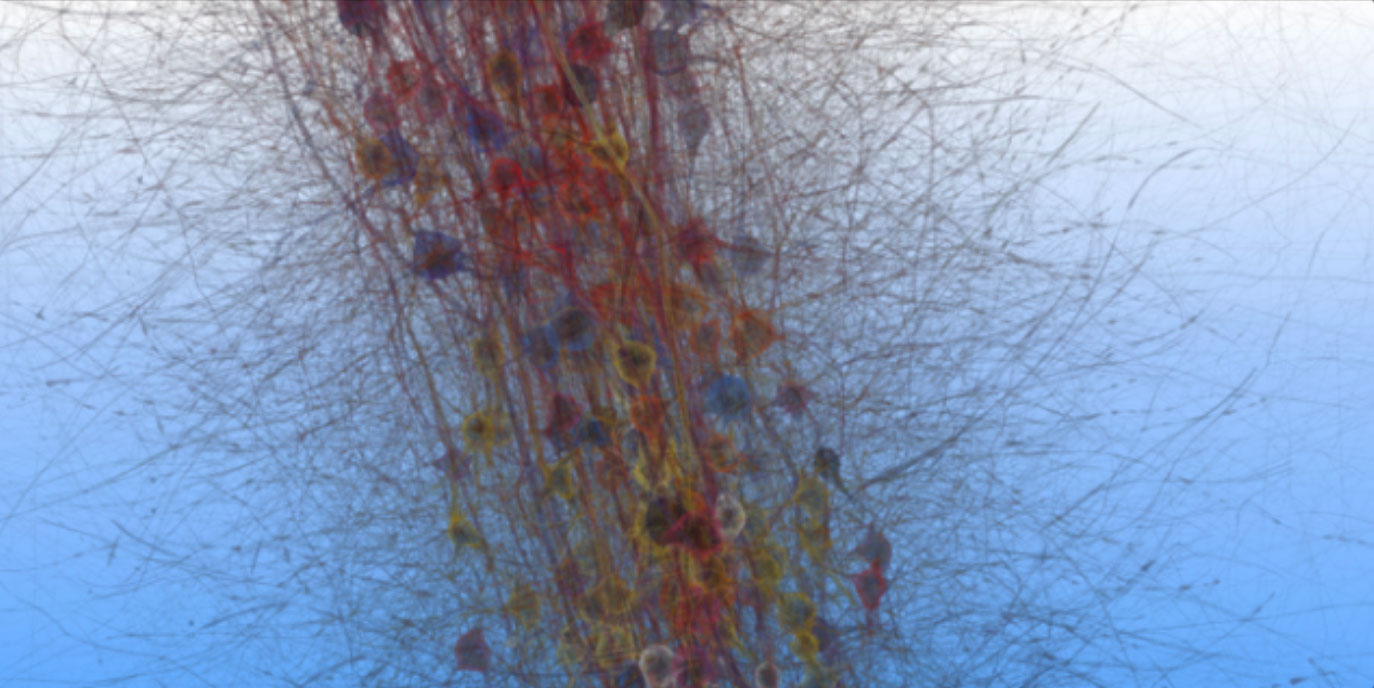
Eilemann, S. Bilgili, A. Abdellah, M. Hernando, J. Makhinya, M. Pajarola, R. and Schürmann, F. (2012). Parallel Rendering on Hybrid Multi-GPU Clusters In Eurographics Symposium on Parallel Graphics and Visualization ; Childs, H., Kuhlen, T., Eds.; The Eurographics Association, 2012; pp 109–117. DOI: 10.2312/EGPGV/EGPGV12/109-117
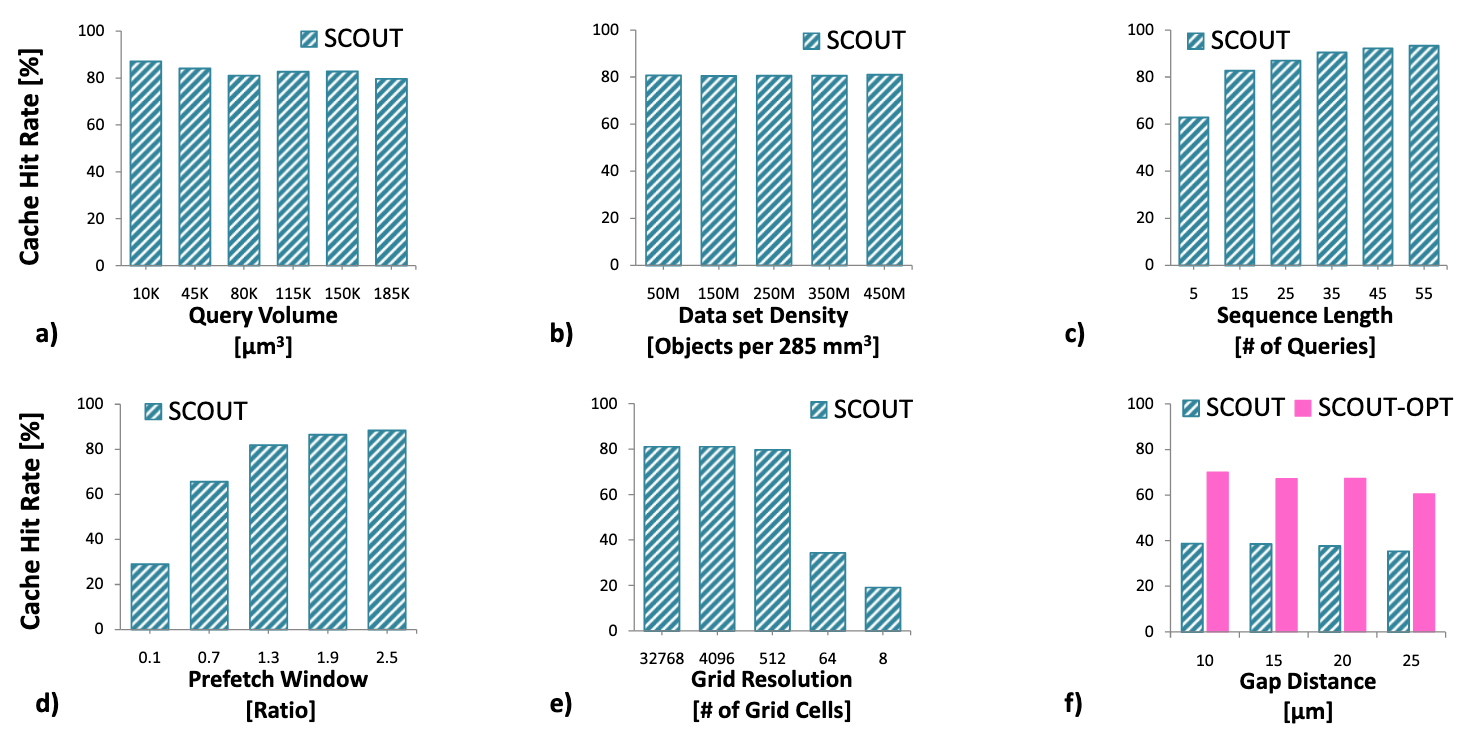
Tauheed, F. Heinis, T. Schürmann, F. Markram, H. Ailamaki, A. SCOUT: Prefetching of Latent Structure Following Queries Proc VLDB Endow 2012, 5 (11), 1531–1542.
DOI: 10.14778/2350229.2350267

Hernando, J. Schürmann, F. Pastor, L. Towards real-time visualization of detailed neural tissue models: view frustum culling for parallel rendering In IEEE Symposium on Biological Data Visualization (BioVis); IEEE, 2012; pp 25–32.
DOI: 10.1109/BioVis.2012.6378589
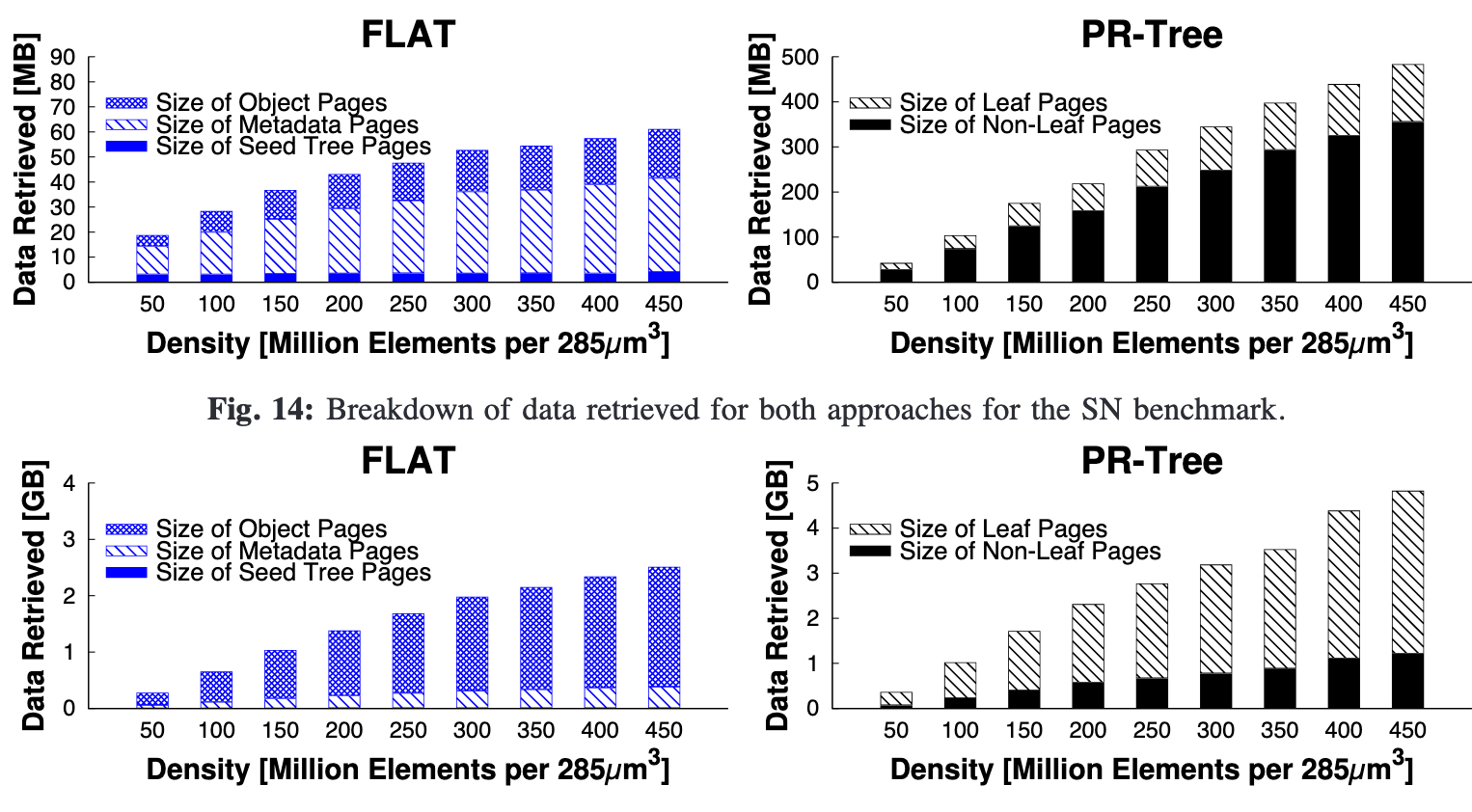
Tauheed, F. Biveinis, L. Heinis, T. Schürmann, F. Markram, H. Ailamaki, A. Accelerating range queries for brain simulations In IEEE, Proceedings of the 2012 28th International Conference on Data Engineering; ICDE ’12; IEEE Computer Society: Washington, DC, USA, 2012; pp 941–952.
DOI: 10.1109/ICDE.2012.56
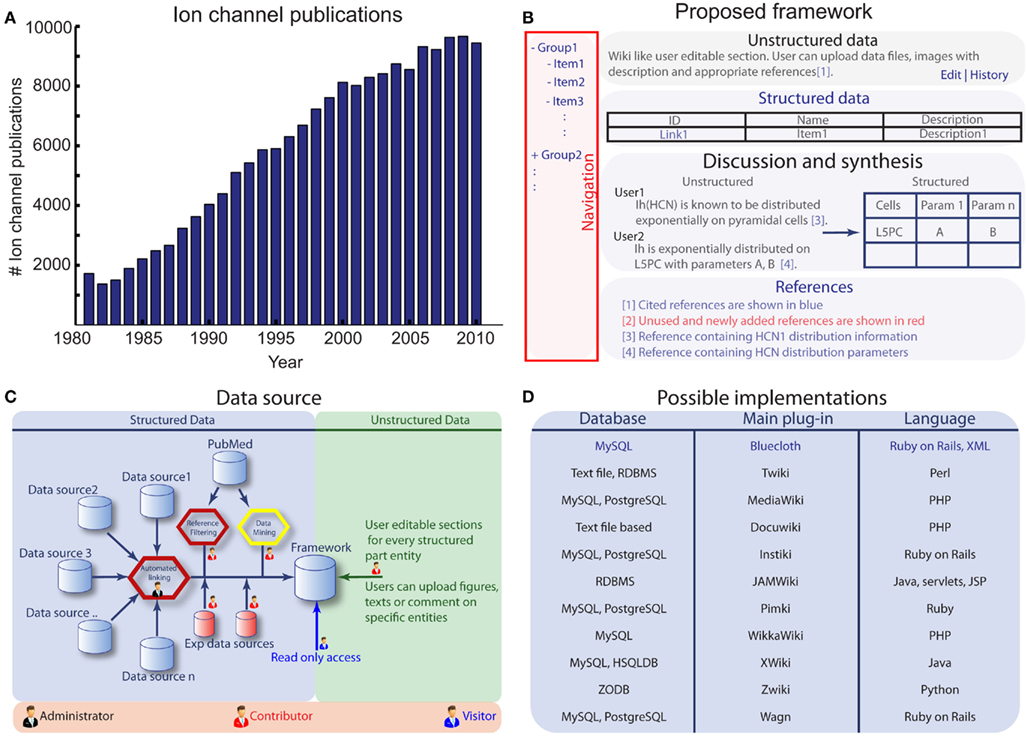
Ranjan, R. Khazen, G. Gambazzi, L. Ramaswamy R, Hill, SL. Schürmann, F. and Markram, H. Channelpedia: an integrative and interactive database for ion channels Front. Neuroinform. 2011; 5:36.
DOI: 10.3389/fninf.2011.00036
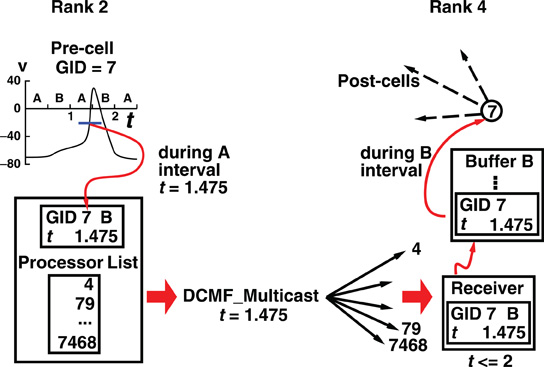
Hines, M. Kumar, S. and Schürmann, F. Comparison of neuronal spike exchange methods on a Blue Gene/P supercomputer Front. Comput. Neurosci 2011. 5:49.
DOI: 10.3389/fncom.2011.00049
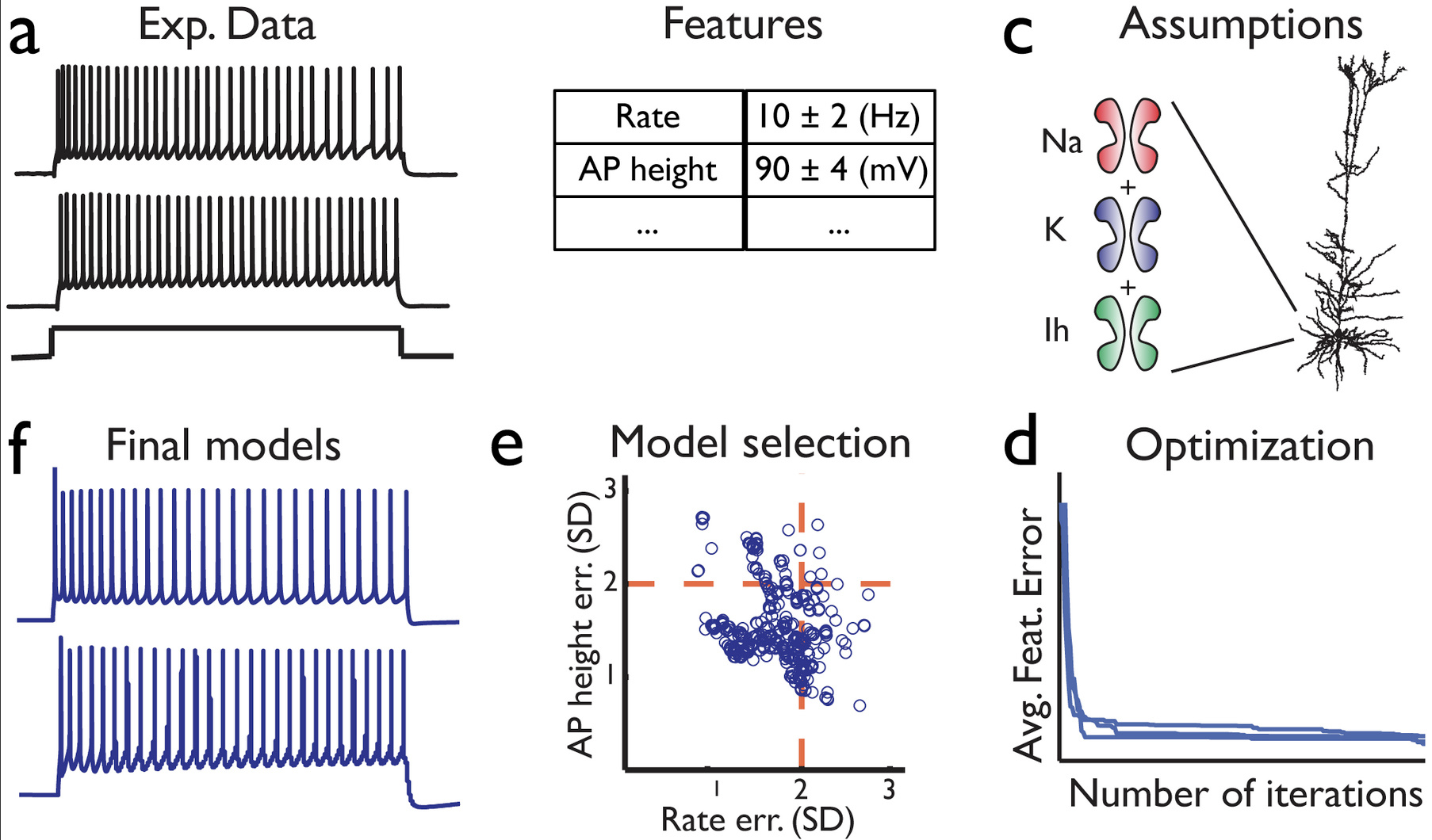
Druckmann, S. Berger, T. Schürmann, F. Hill, S. Markram, H. and Segev, I. Effective Stimuli for Constructing Reliable Neuron Models PLoS Comp Biol, 7(8): e1002133.
DOI: 10.1371/ journal.pcbi.1002133
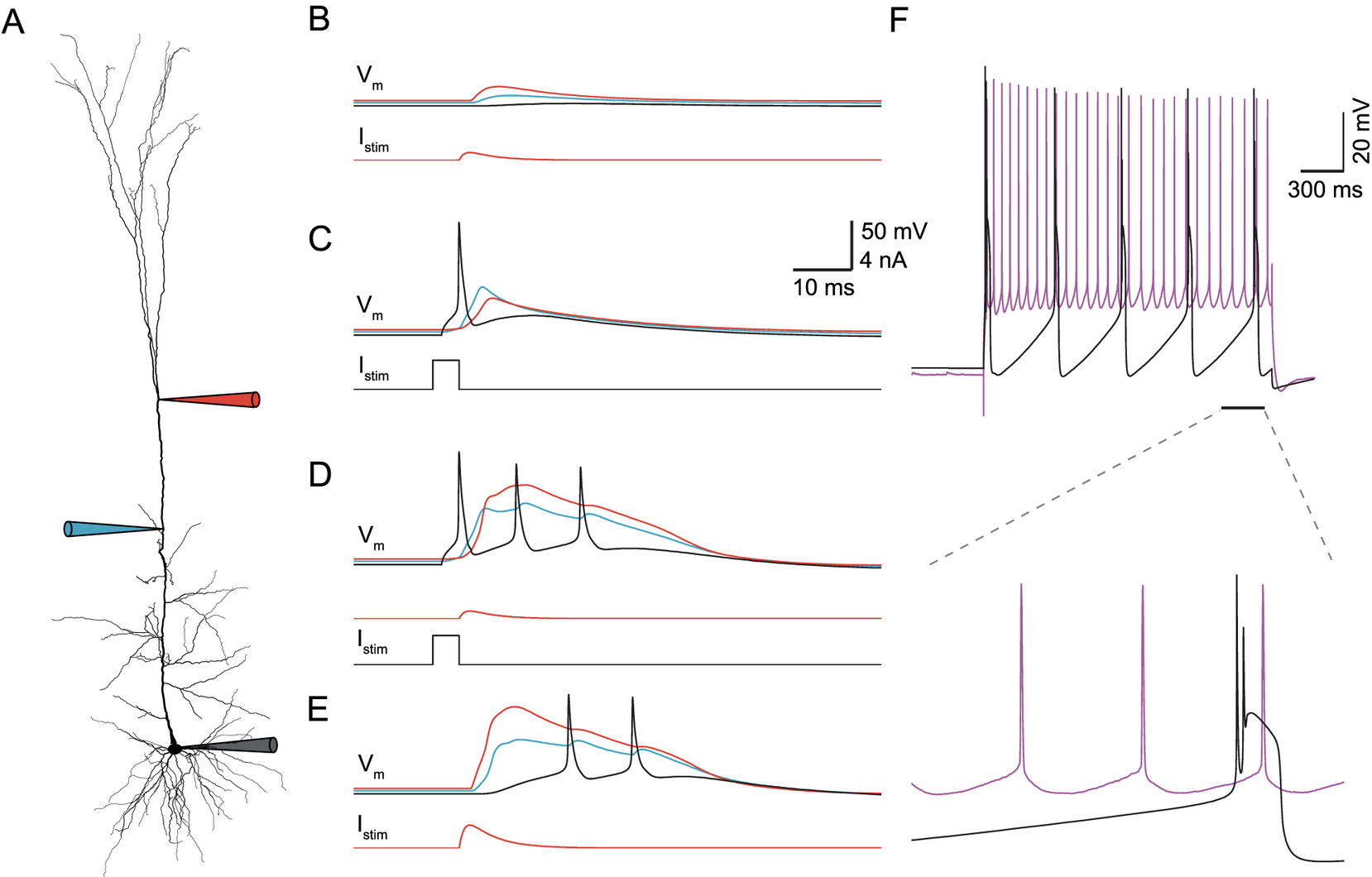
Hay, E. Hill, S. Schürmann, F. Markram, H. and Segev, I. Models of Neocortical Layer 5b Pyramidal Cells Capturing a Wide Range of Dendritic and Perisomatic Active Properties PLoS Comp Biol, 7(7): e1002107.
DOI: 10.1371/journal.pcbi.1002107
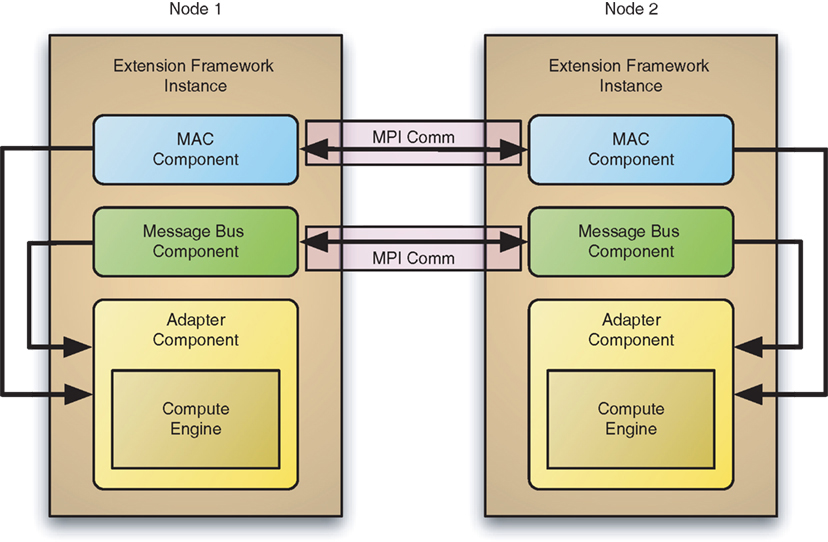
King, JG. Hines, M. Hill, S. Goodman, PH. Markram, H. Schürmann, F. A component-based extension framework for large-scale parallel simulations in NEURON Frontiers in Neuroinformatics, 3:10,
DOI: 10.3389/neuro.11.010.2009
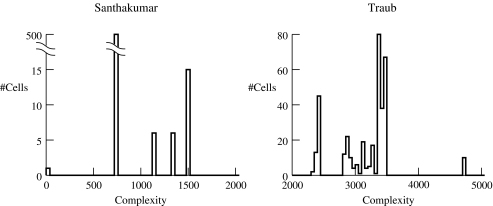
Hines, M. Eichner, H. Schürmann, F. Neuron splitting in compute-bound parallel network simulations enables runtime scaling with twice as many processors J. Comput. Neurosci., 25(1):203-10, 2008
DOI: 10.1007/s10827-007-0073-3
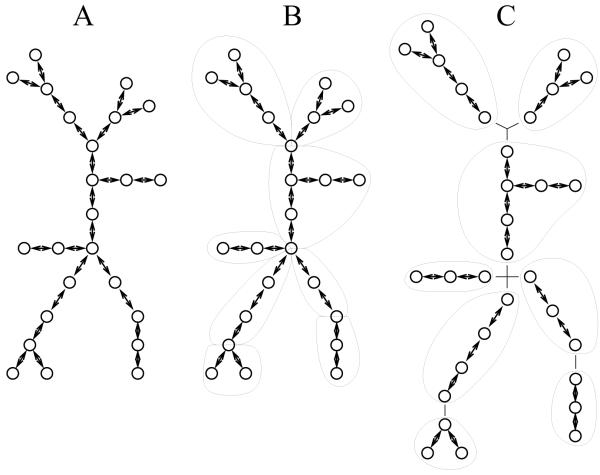
Hines, M. Markram, H. and Schürmann, F. Fully Implicit Parallel Simulation of Single Neurons J. Computational Neuroscience, 2008 Volume 25, Number 3, 439-448.
DOI: 10.1007/s10827-008-0087-5
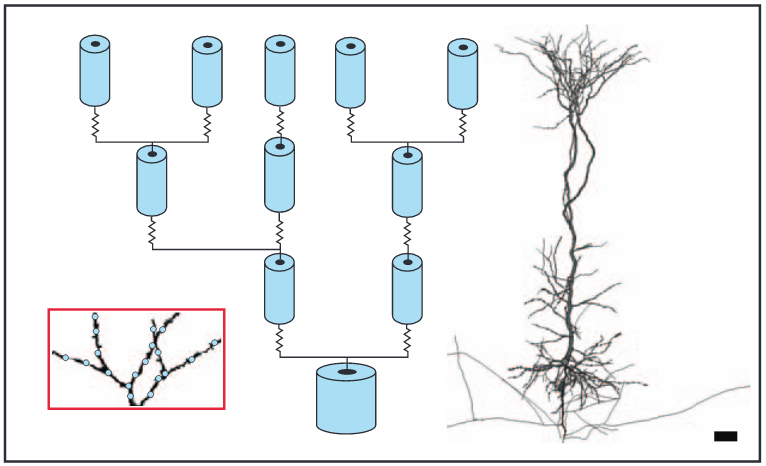
Kozloski, J. Sfyrakis, K. Hill, S. Schürmann, F. Peck, C. Markram, H. Identifying, tabulating, and analyzing contacts between branched neuron morphologies IBM Journal of Research and Development 2008, 52 (1.2), 43–55.
DOI: 10.1147/rd.521.0043
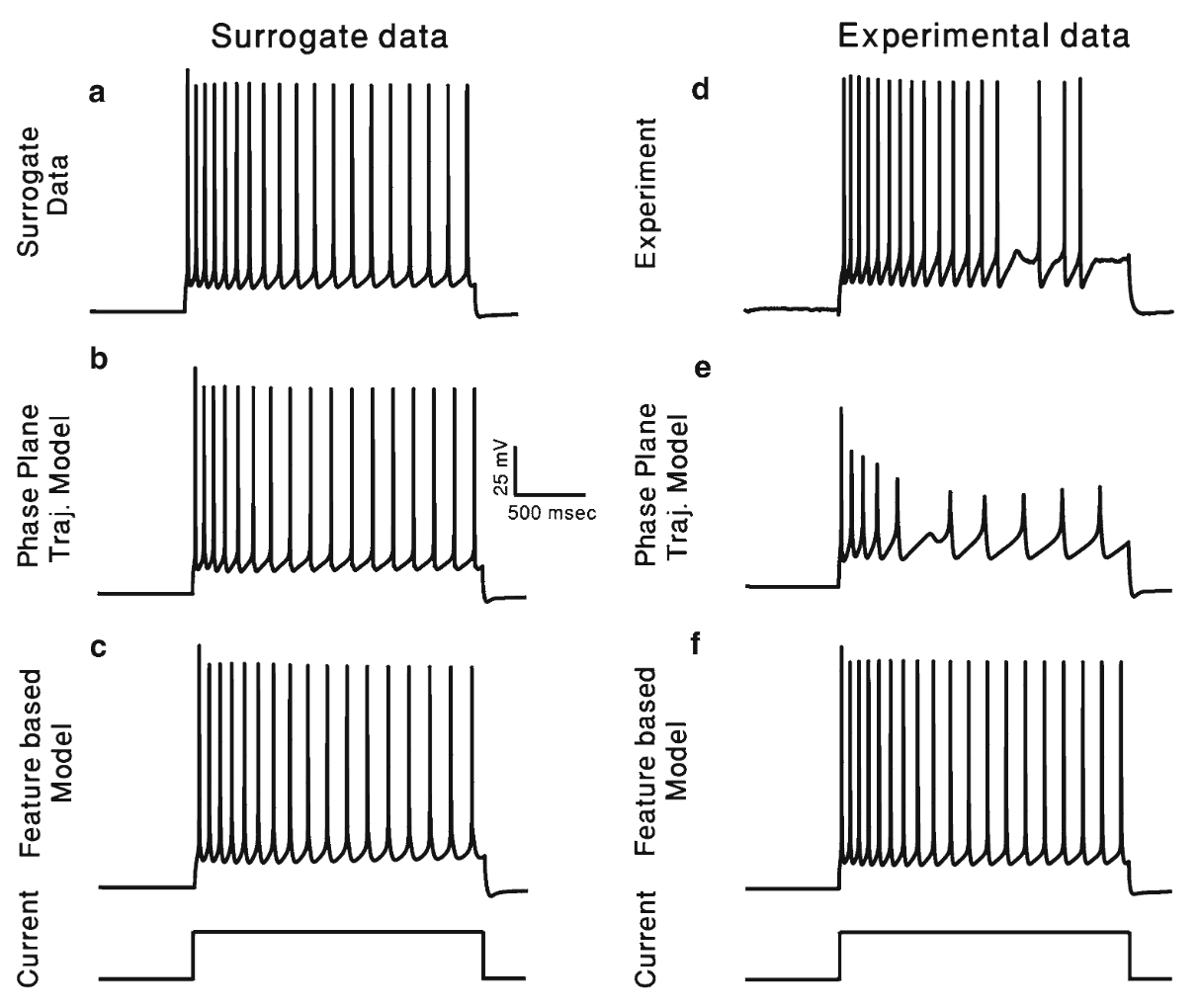
Druckmann, S. Berger, TK. Hill, S. Schürmann, F. Markram, H. Segev, I. Evaluating automated parameter constraining procedures of neuron models by experimental and surrogate data Biol Cybern. 2008 Nov;99(4-5):371-9. Epub 2008 Nov 15.
DOI: https://doi.org/10.1007/s00422-008-0269-2
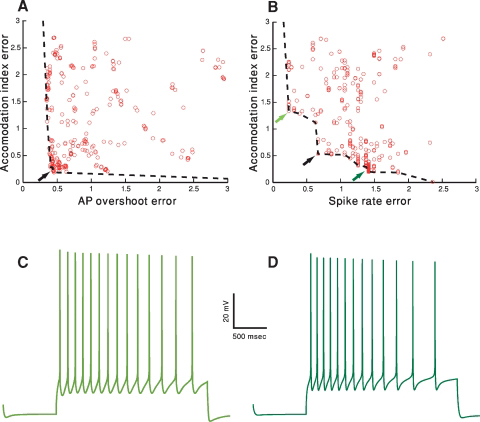
Druckmann, S. Banitt, Y. Gidon, A. Schürmann, F. Markram, H. and Segev, I. A Novel Multiple Objective Optimization Framework for Constraining Conductance-Based Neuron Models by Experimental Data Frontiers in Neuroscience, Vol. 1, Issue 1, 2007
DOI: 10.3389/neuro.01.1.1.001.2007
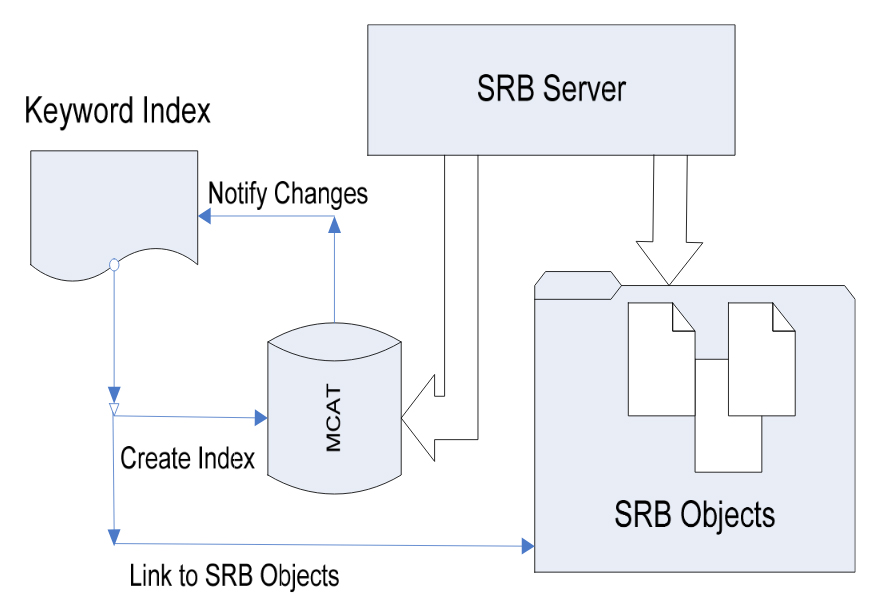
Abid, A. Jan, A. Francioli, L. Sfyrakis, K. and Schürmann, F. Keyword Based Indexing and Searching over Storage Resource Broker OTM Conferences, 2007, Proceedings, Part II. Lecture Notes in Computer Science 4804 Springer 2007, ISBN 978-3-540-76835-7, pp. 1233-43
DOI: 10.1007/978-3-540-76843-2_6
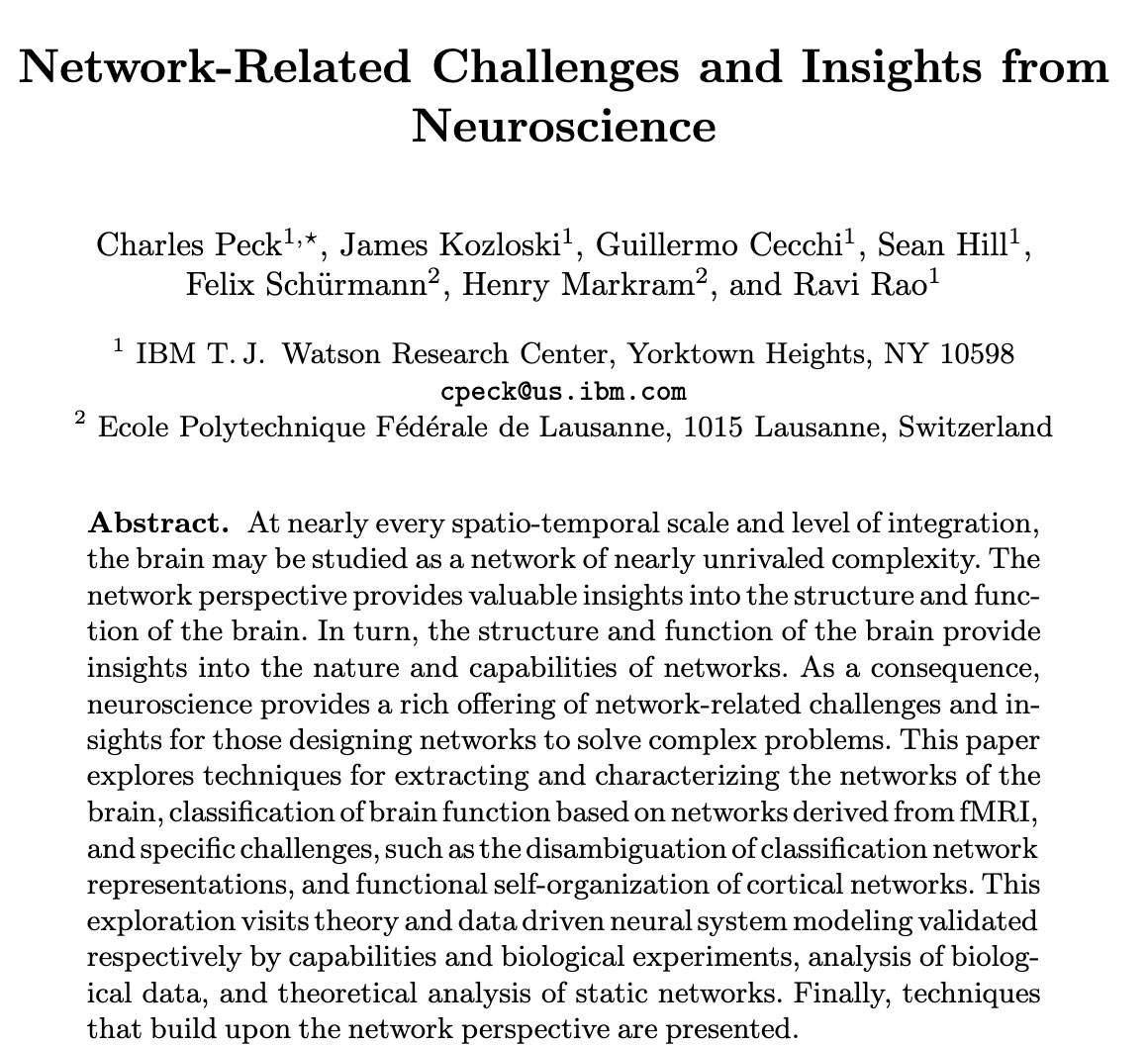
Peck, C. Kozloski, J. Cecchi, G. Hill, S. Schürmann, F. Markram, H. Rao, R Network-Related Challenges and Insights from Neuroscience BIOWIRE 2007, Lecture Notes in Computer Science 5151 Springer 2008, ISBN 978-3-540-92190-5, pp. 67-78
DOI: 10.1007/978-3-540-92191-2_7
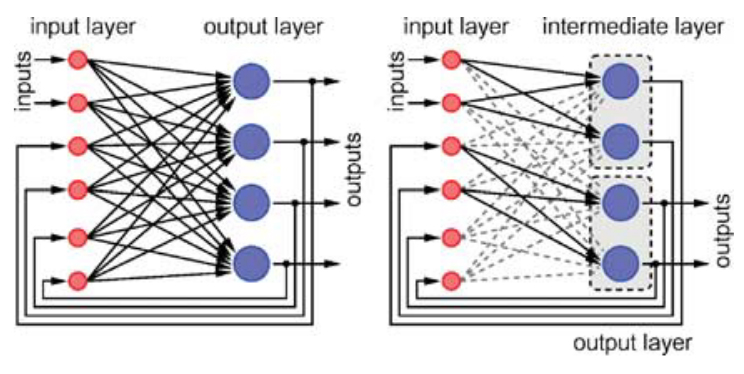
Schemmel, J. Hohmann, S. Meier, K. Schürmann, F. A Mixed-Mode Analog Neural Network using Current-Steering Synapses Special Issue of Analog Integrated Circuits and Signal Processing, Kluwer, Vol 38, Issue 2-3, pp. 233-44, 2004
DOI: 10.1023/B:ALOG.0000011170.92377.6e
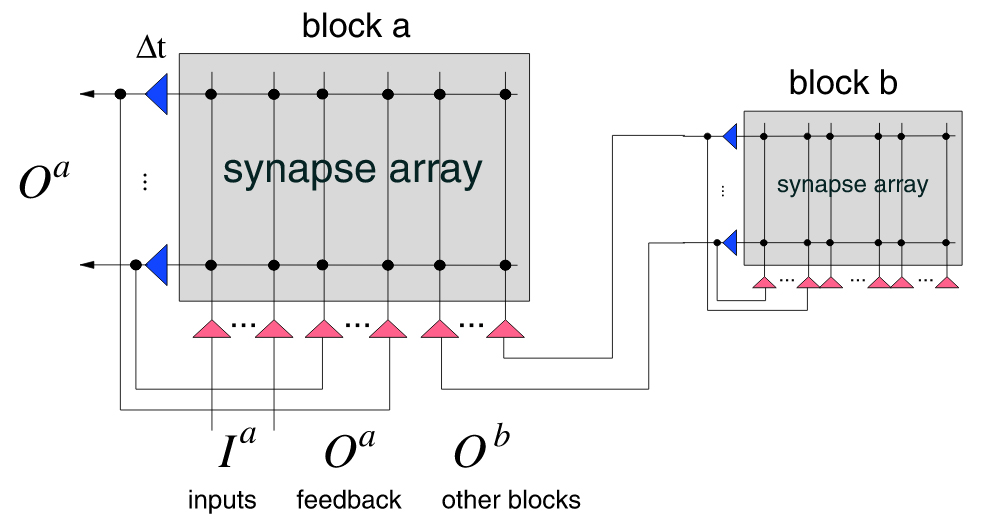
Schürmann, F. Meier, K. Schemmel, J. Edge of Chaos Computation in Mixed-Mode VLSI – “A Hard Liquid” Advances in Neural Information Processing Systems 17 (NIPS 2004), eds. Saul, Weiss and Bottou, MIT Press, pp.1201-08, 2005
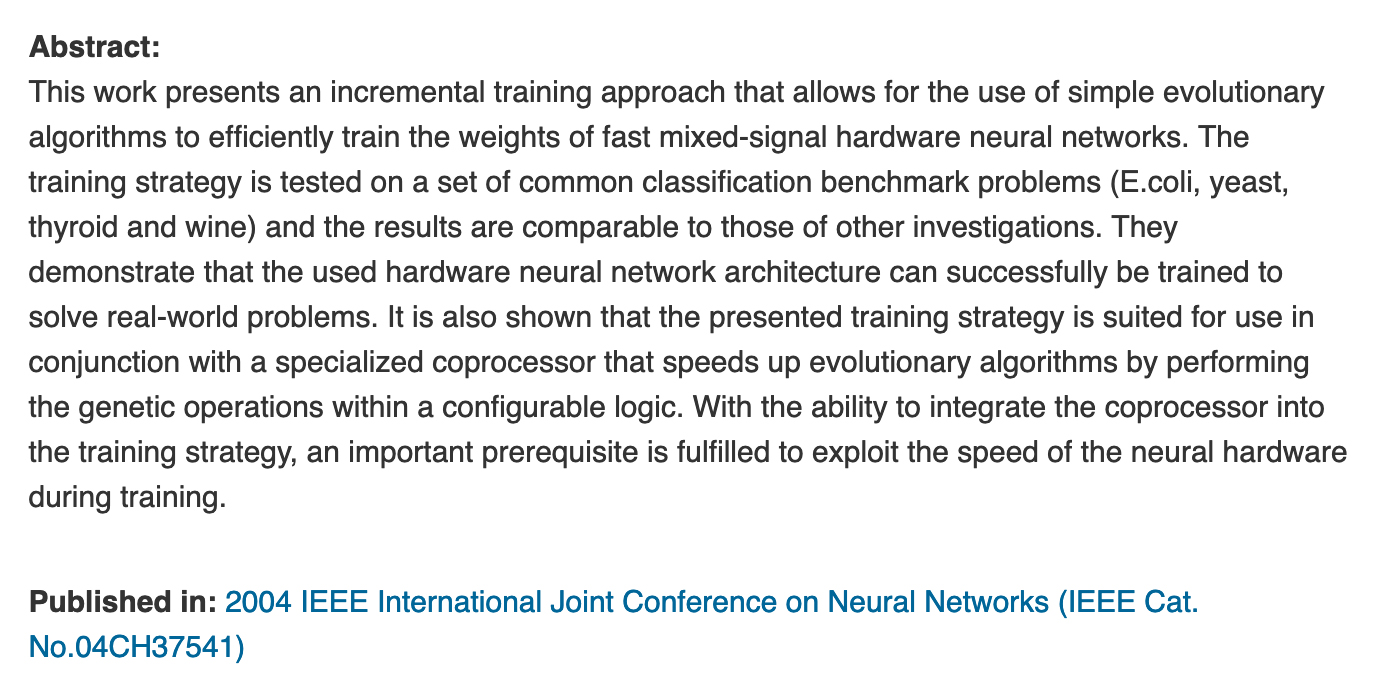
Hohmann, S. Fieres, J. Meier, K. Schemmel, J. Schmitz, T. Schürmann, F. Training Fast Mixed-Signal Neural Networks for Data Classification Proceedings of the 2004 International Joint Conference on Neural Networks IJCNN”04, Budapest, Hungray, July 25.-29., 2004, IEEE Press
DOI: 10.1109/IJCNN.2004.1381065

Fieres, J. Grübl, A. Philipp, S. Meier, K. Schemmel, J. Schürmann, F. A Platform for Parallel Operation of VLSI Neural Networks Brain Inspired Cognitive Systems BICS2004,
University of Stirling, Scotland, UK, August 29 – September 1, 2004
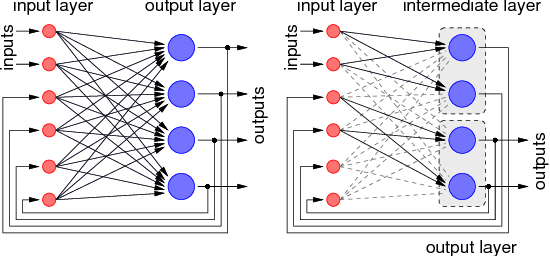
Schürmann, F. Hohmann, S. Meier, K. Schemmel, J. Interfacing Binary Neurons to Multi-valued Signals Supplementary Proceedings of the Joint International Conference ICANN/ICONIP 2003, pp. 430-433,
ISBN: 975-518-209-8, 2003
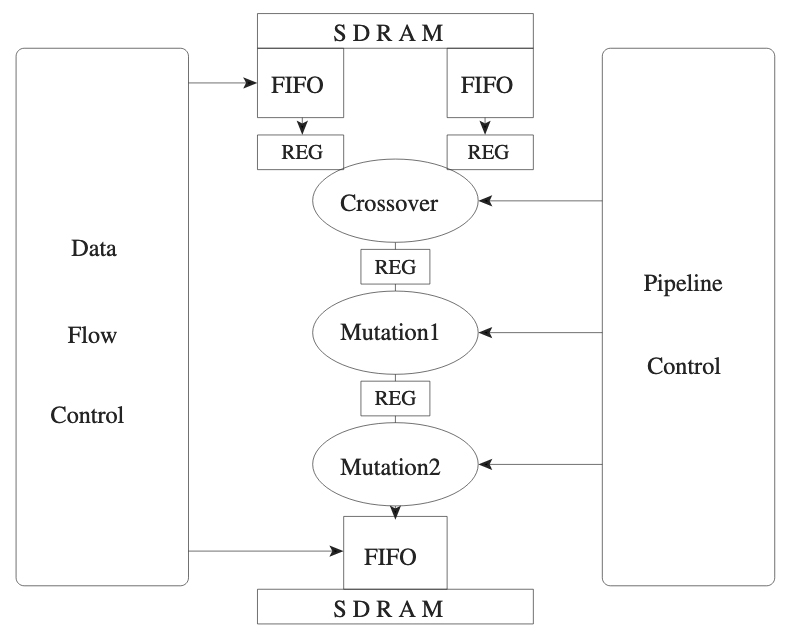
Schmitz, T. Hohmann, S. Meier, K. Schemmel, J. Schürmann, F. Speeding Up Hardware Evolution: A Coprocessor for Evolutionary Algorithms Proceedings of the International Conference on Evolvable Systems ICES 2003, pp. 274-285, Springer Verlag, 2003
DOI: 10.1007/3-540-36553-2_25
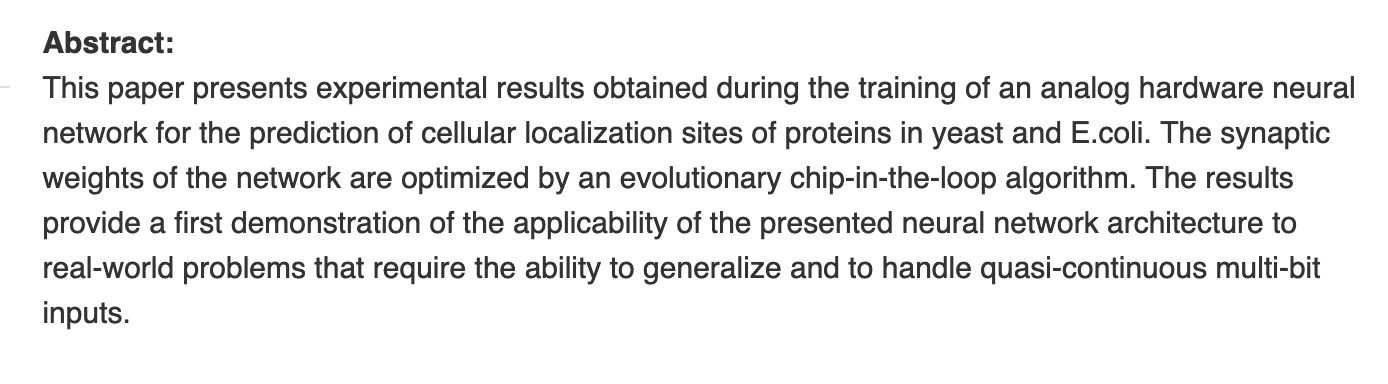
Hohmann, S. Schemmel, J. Schürmann, F. Meier, K. Predicting Protein Cellular Localization Sites with a Hardware Analog Neural Network Proc. of July 2003 Int. Joint Conf. on Neural Networks, Portland, Oregon, IEEE Press, 0-7803-7899-7, pp 381-86, 2003
DOI: 10.1109/IJCNN.2003.1223376
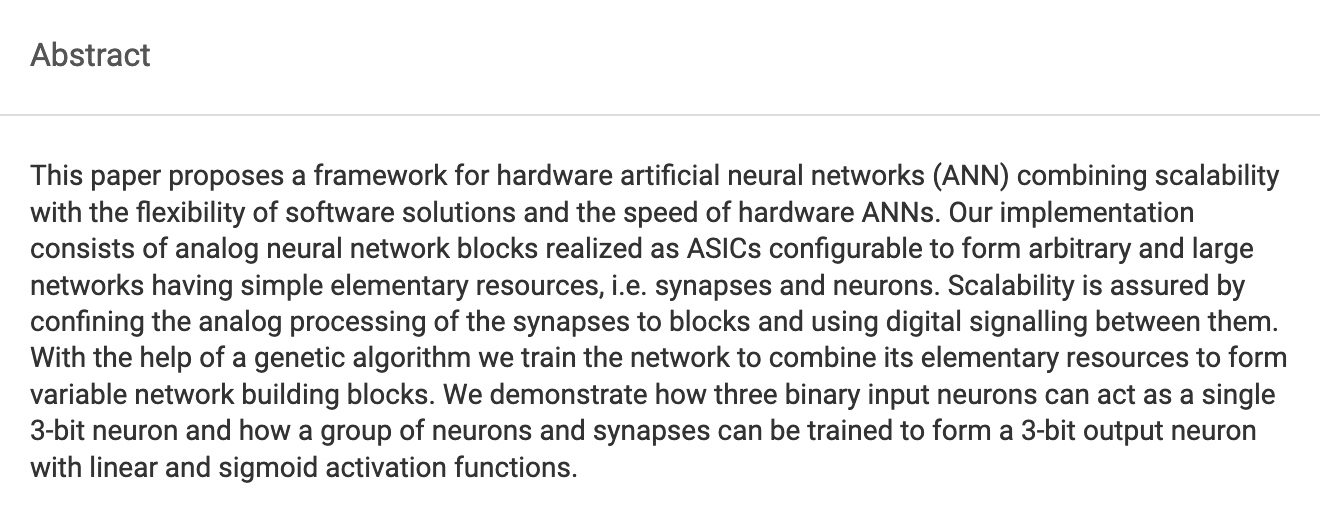
Schürmann, F. Hohmann, S. Schemmel, J. Meier, K. Towards an Artificial Neural Network Framework Proceedings of the 2002 NASA/DoD Conference on Evolvable Hardware, pp. 266-273, IEEE Computer Society, 2002
DOI: 10.1109/EH.2002.1029893
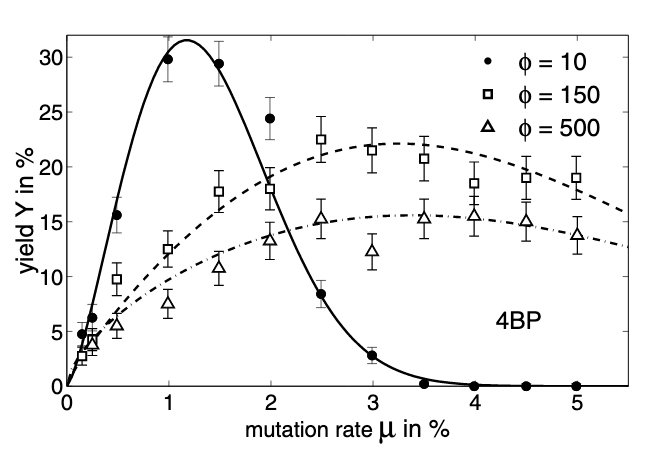
Hohmann, S. Schemmel, J. Schürmann, F. Meier, K. Exploring the Parameter Space of a Genetic Algorithm for Training an Analog Neural Network Proceedings of the Genetic and Evolutionary Computation Conference GECCO 2002, pp.375-382,
Morgan Kaufmann Publishers, 2002
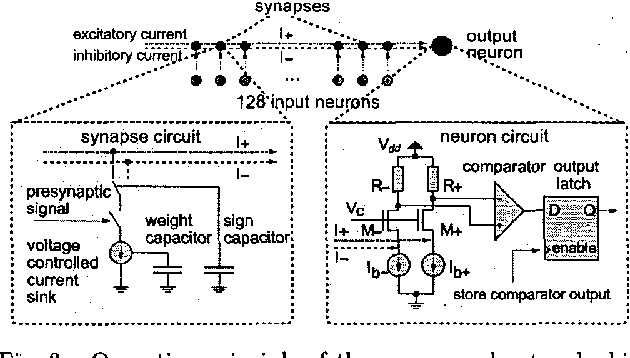
Schemmel, J. Schürmann, F. Hohmann, S. Meier, K. An Integrated Mixed-Mode Neural Network Architecture for Megasynapse ANNs Proceedings of the 2002 International Joint Conference on Neural Networks IJCNN 2002, pp. 2704-2710, IEEE Press, 2002
DOI: 10.1109/IJCNN.2002.1007574
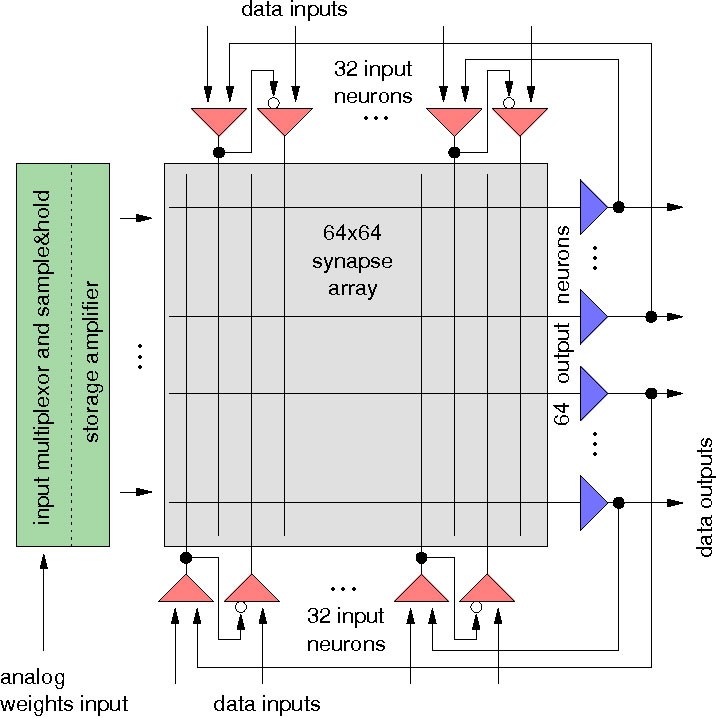
Schemmel, J. Schürmann, F. Meier, K. A VLSI Implementation of an Analog Neural Network suited for Genetic Algorithms Proceedings of the International Conference on Evolvable Systems ICES 2001, Springer Verlag, 2001 Summary Articles
DOI: 10.1007/3-540-45443-8_5
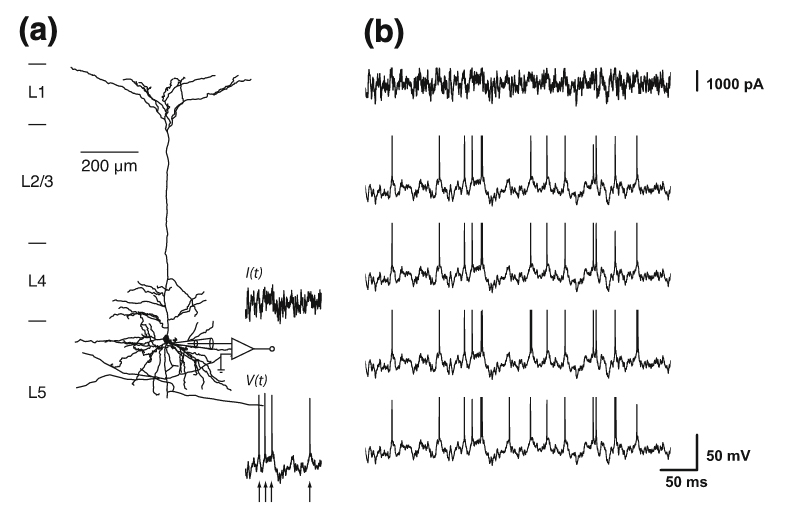
Jolivet, R. Schürmann, F. Berger, T. Naud, R. Gerstner, W. Roth, A. The quantitative single-neuron modeling competition Biological Cybernetics 99(4-5): 417-26, 2008
DOI: 10.1007/s00422-008-0261-x
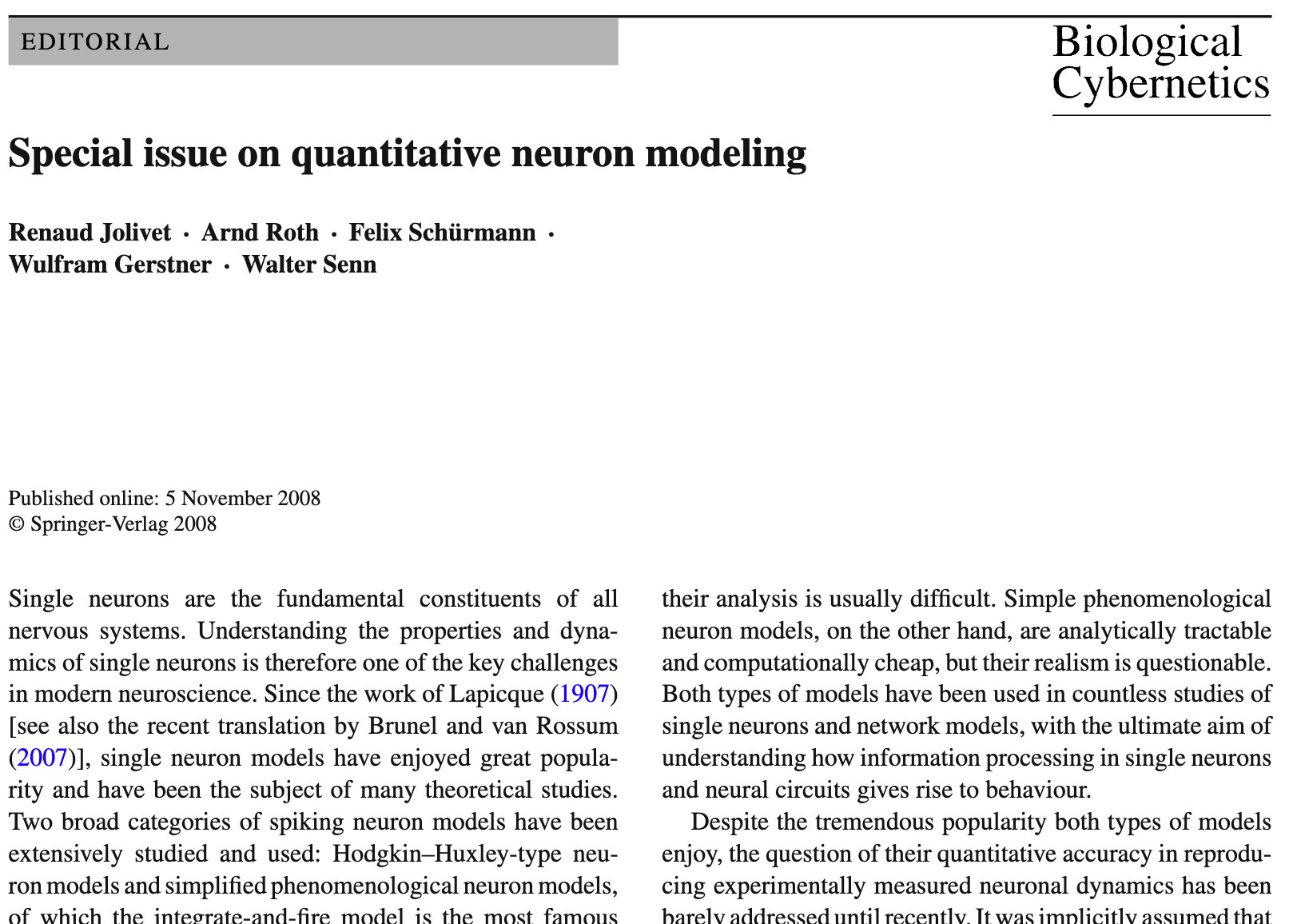
Jolivet, R. Roth, A. Schürmann, F. Gerstner, W. Senn, W. Special issue on quantitative neuron modeling Biological Cybernetics 99(4-5): 237-39, 2008
DOI10.1007/s00422-008-0274-5
Contributions to books
Anwar H, Riachi I, Hill S, Schürmann F, Markram H: An Approach to Capturing Neuron Morphological Diversity. Computational Modeling Methods for Neuroscientists, E. de Schutter (ed), MIT Press, 2010
DOI: 10.7551/mitpress/9780262013277.001.0001
PhD Thesis
Schürmann F, Exploring Liquid Computing in a Hardware Adaptation: Construction and Operation of a Neural Network Experiment, PhD thesis, urn:nbn:de:bsz:16-opus-56156, 2005
DOI: https://doi.org/10.11588/heidok.00005615