Remote sensing data are acquired at fast pace and in large quantities. It is nowadays possible to monitor Earth processes at a high temporal resolution or detect objects in near real time. In other words: query the world on demand.
However, it is complicated for non technical people to develop AI-based models specific to the problem of interest, and even when such model exists, using it for finding specific answers is difficult.
Being able to summarize image content or to ask questions in english can increase usage and value of remote sensing.
We develop deep learning models able to caption images or answer questions asked in english about remote sensing image content. Joining expressive power of Convolutional neural networks and language models from NLP, we extract information from both images and text to answer the environmental questions.
Papers
- Chappuis, Zermatten, Lobry, Le Saux, Tuia (2022): Prompt-RSVQA: prompting visual context to a language model for remote sensing visual question answering, Computer Vision and Pattern Recognition (CVPR) Workshops (CVF open access paper)
- Mi, Li, Chappuis, Tuia (2022): Knowledge-aware cross-modal text-image retrieval for remote sensing images, International Joint Conference on Artificial Intelligence (IJCAI) Workshops.
- Lobry, Marcos, Murray, Tuia (2020). RSVQA: Visual Question Answering for Remote Sensing Data, IEEE Transaction on Geoscience and Remote Sensing (arxiv)
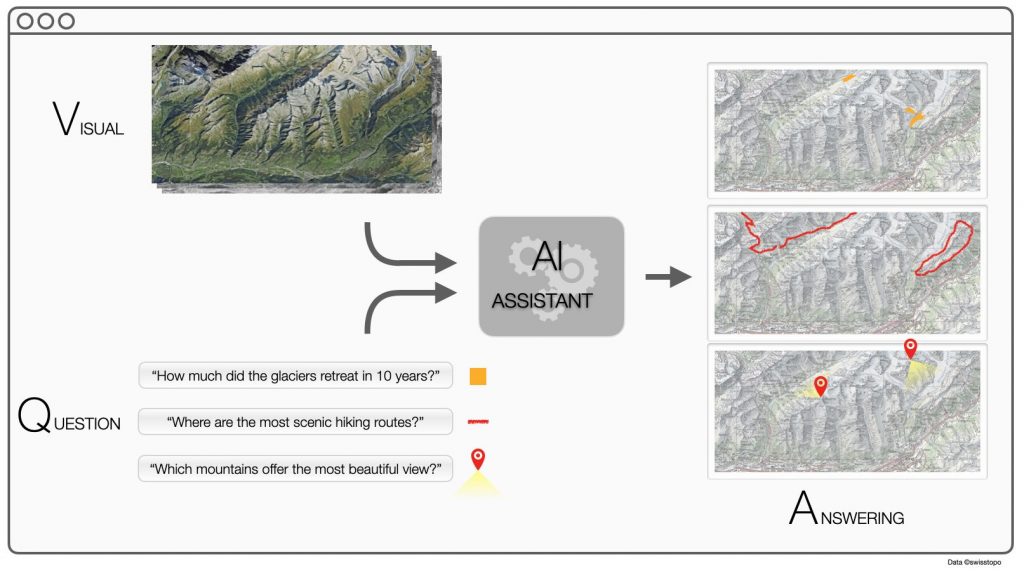