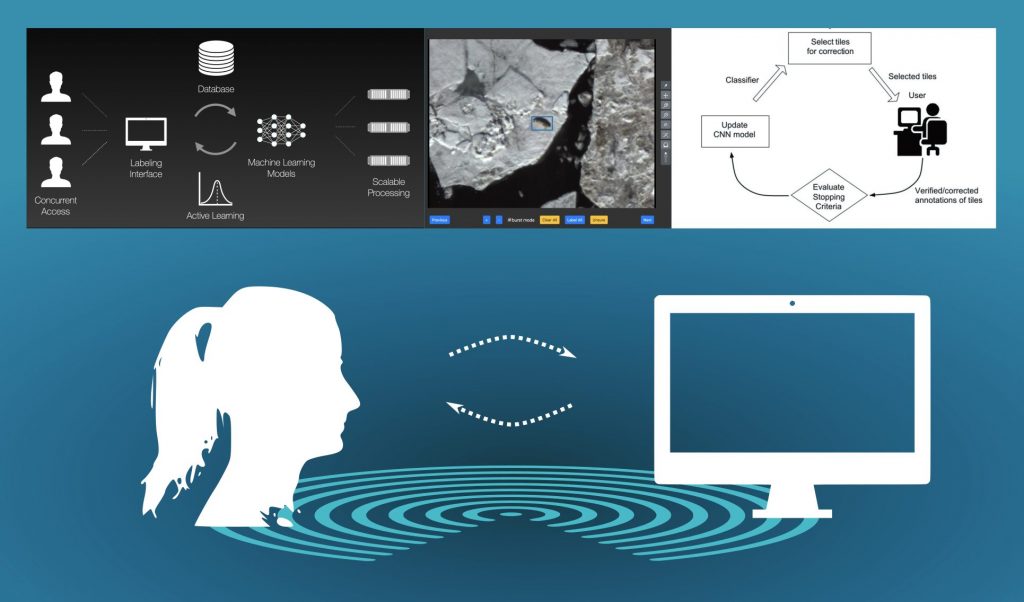
At ECEO, we include humans in the loop and incorporate their knowledge in the algorithms we build. We make active use of the knowledge and experience users have, to feed back information to our models and the other way around! We also work in an open and transparent way, and provide models where one can see what happens under the hood.
Designing machines around and with users has the potential to empower both parties simultaneously.
We design models that have the capability to process large amounts of data, while also looking for feedback from humans for uncertain situations. Effectively, we resort to expert knowledge where it is the most useful, alleviating users from the tedium of large-scale data annotation, and training models with smart instead of expansive collections of data.
Papers
- Hua, Marcos, Mou, Zhu, Tuia (2022). Semantic segmentation of remote sensing images with sparse annotations. IEEE Geoscience and Remote Sensing Letters (paper).
- Vargas, J. E., Tuia, D. and Falcão, A. X. (2021). Deploying machine learning to assist digital humanitarians: making image annotation in OpenStreetMap more efficient. International Journal of Geographic Information Science (paper).
- Kellenberger, B., Tuia, D., Morris, D. (2020). AIDE: Accelerating Image-Based Ecological Surveys with Interactive Machine Learning. Methods in ecology and evolution (paper).
- Tuia, D., Muñoz-Marí (2013) Learning user’s confidence for active learning. IEEE Transactions on Geoscience and Remote Sensing, 51(2):872–880 (preprint).