Physics of Complex Systems Laboratory
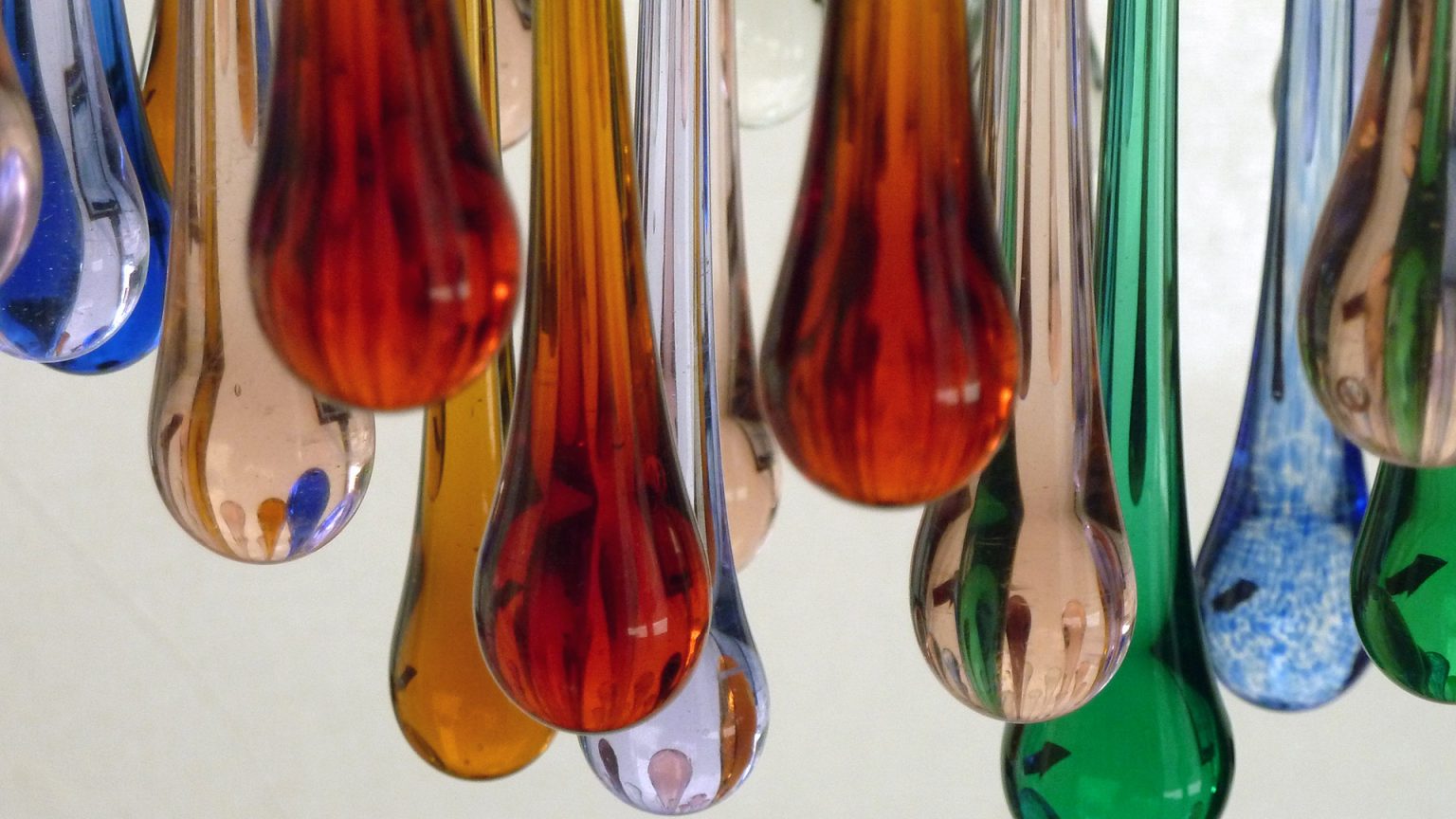
© User:Warburg / Wikimedia Commons / CC-BY-SA-3.0
Welcome to our Research Group
Complex systems present an abundance of long-lived metastable states. They do not equilibrate easily, and therefore remember their past. Examples include glassy materials (such the glass that makes our windows, sand, spin and electron glasses) as well as dynamical systems that appear in biology (neuronal or proteins regulation networks, or ecologies).
The Physics of Complex Systems Laboratory develops tools in statistical mechanics and dynamical systems to address both associated conceptual questions (such as the principles governing sampling in these non-equilibrium situations) and practical ones (e.g. how particles manage to move while avoiding each other in a crowded environment, such as a dense granular flow).
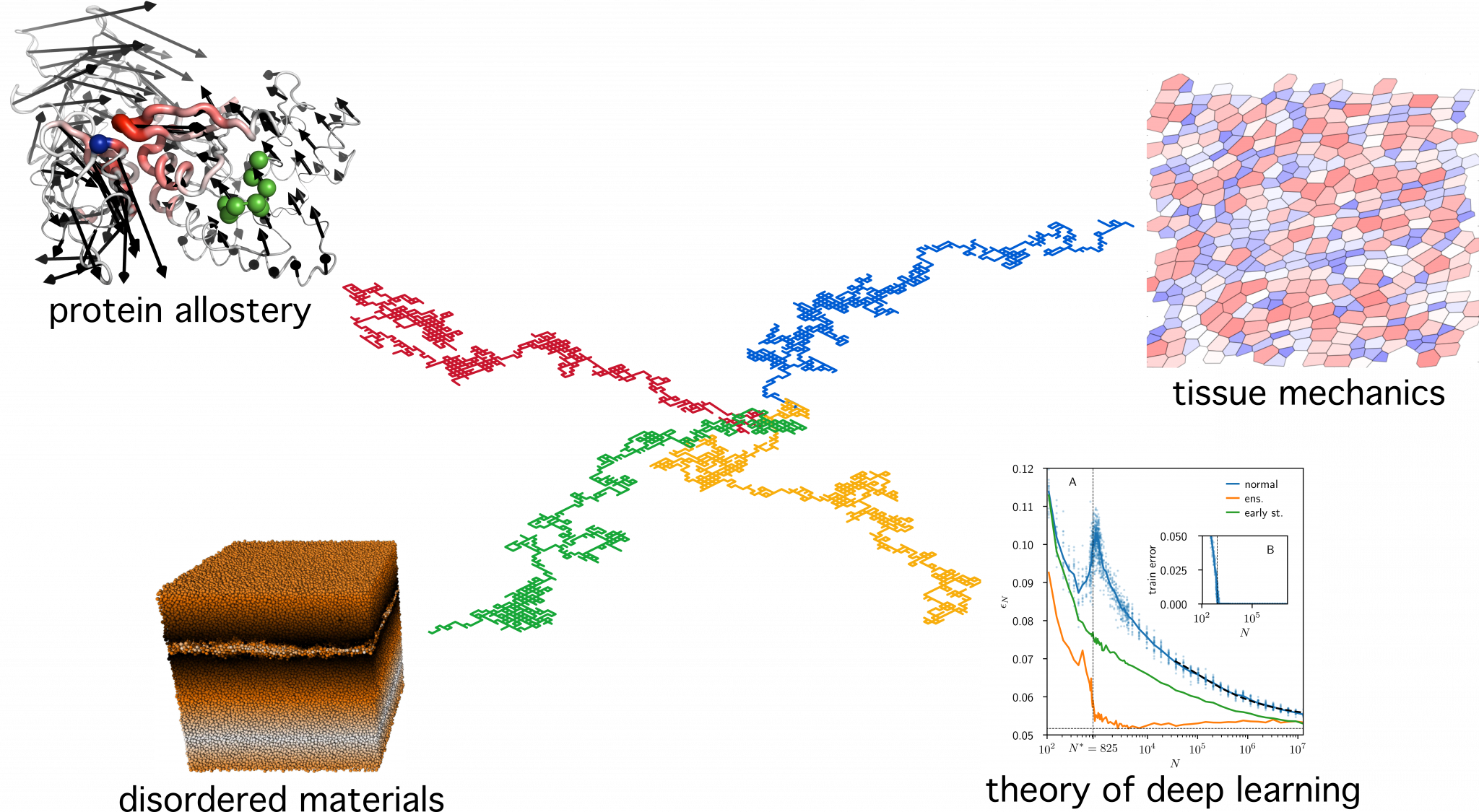
Amorphous materials
Many materials around us are amorphous, including structural glasses, granular materials, foams, colloidal suspensions, pastes and plastics. Describing them remains a central challenge of condensed matter: in their solid phase, they form glassy, out-of-equilibrium structures. In their fluid phase, they are strongly driven and thus cannot be described perturbatively around some equilibrium liquid phase.
We have proposed that key aspects of both the solid and the dense liquid phase are controlled by the proximity of the transition that separates these two phases. This leads to a description of the solid based on the elementary excitations governing their linear response (the “anomalous” or “soft” modes) and their plasticity (localized shear transformations if particles are soft, or rewiring of contact network if particles are hard). The dense liquid phase is then described as a dilute gas of excitations, whose concentration goes to zero as jamming is approached.
We are exploring the consequences of this framework in practical situations. It includes granular flows, where friction raises new fundamental questions, or erosion where only a tiny layer of the material is fluidized. We are currently extending this approach to frictional interfaces between two solids. We seek in particular to describe how collective asperity detachments can nucleate a fracture-like front, ultimately leading to microscopic slip.
Living amorphous matter
Living materials offer a new twist not present in physical systems: they have evolved to be functional. A particularly interesting case are well-folded proteins. Some of these proteins are allosteric: binding a ligand at some allosteric site can trigger a change of function at a distant active site.
Understanding this surprising behavior remains a challenge. Relevant questions include: What are the physical and mechanical principles underlying such action at a distance? What information propagates in the protein? What are the best architectures for propagation, and can we classify them? How does the mechanical organisation of the protein transpire in the now abundant sequence data of protein families?
We approach these questions using in-silico evolution of functional materials and empirical tests of our theory using the Protein Data Bank.
Theory of deep learning
A prerequisite of artificial intelligence is to interact with the world, which often requires making sense of (e.g. classifying) data lying in high dimensions. It is an extremely hard challenge, yet very recently huge progress has been made using deep neural nets. It led to algorithms that beat the best humans at games like go, self-driving cars, efficient translators, etc…
Yet, little is understood about these algorithms, a situation similar to the industrial revolution where heat engines were impacting the world but thermodynamics was not yet there to explain them. For example, we have a very limited understanding of the number of data required to learn a given task, and how to describe mathematically the structure of a task, its symmetry and invariance.
These algorithms have a profound connection to physics, as learning data correspond to a descent in the landscape of a cost function, similar to a rapid quench in the energy landscape of a glass. We are studying the geometry of this landscape by building analogies with physical systems. We are developing scaling theories for how the performance of these algorithms depends on the number of parameters and the number of data, as well as phase diagrams indicating that learning can qualitatively differ depending on the parameters chosen.