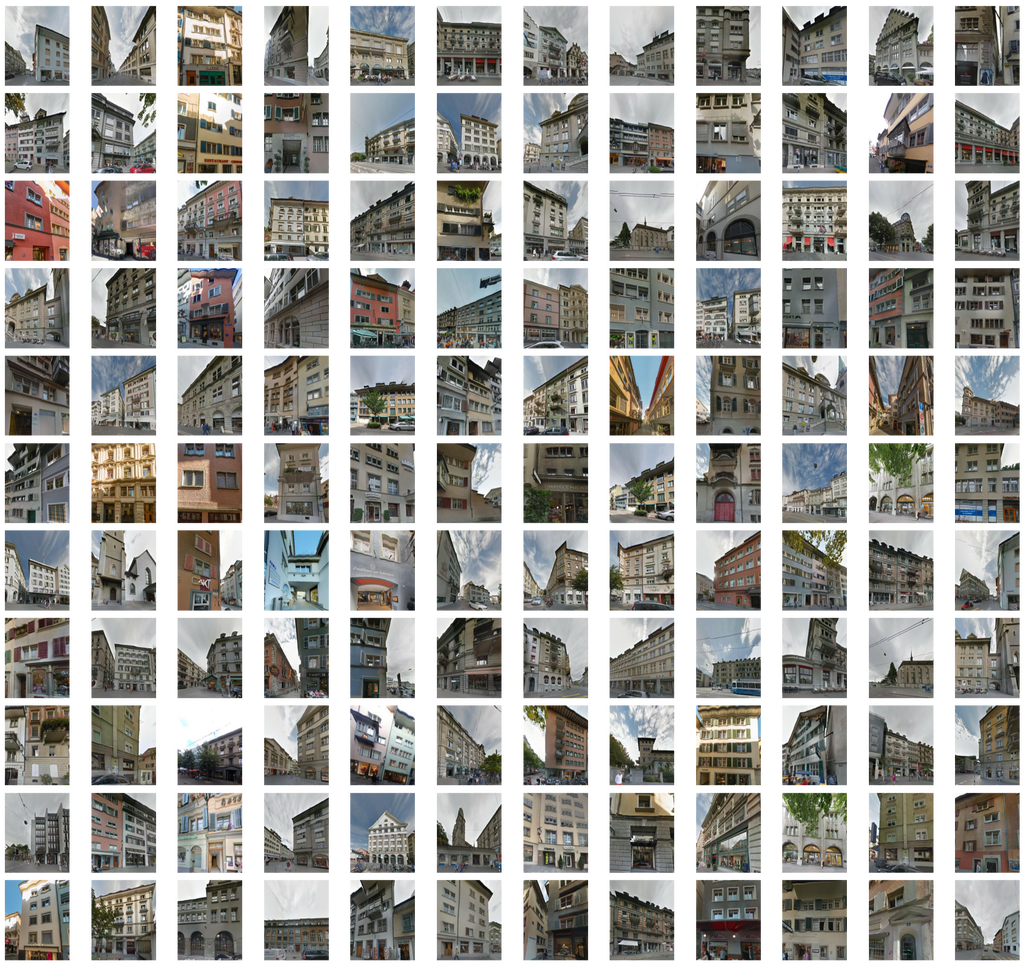
In this research experiment, the GAN algorithm serves as a new mode of vision, bypassing the preconceptions of the human designer in order to reveal an unbiased pool of ideas. The algorithm zeroes in on details otherwise unnoticed to generate new interpretations. The curation of the input data set and the critical analysis of the generated images are critical to the success of the assignment.
Images of the Zürich Altstadt are generated using a GAN algorithm (generative adversarial network) from a data set of about 5000 images. Each researcher trains his or her own GAN on Google Colab (a remote server) with a personal selection of images from the common image pool: the more images the better the algorithm will work. The GAN will eventually learn from these images to generate new interpretation of Zürich Altstadt architecture, and produce a final selection of 144 images which capture “Zürichness” the best.
The data collected establishes the base from which the machine learns as the first point from which to establish the investigation. How to focus the learning to a desired line of study? The number, type of image and composition of the photo determines the variables from which to start working. However, a sufficient number of data must be reached for learning to be optimal, which makes it difficult to work in a very specific line and with sufficient data variants so that the final conversion will be optimal. This fixation can occur, for example, in architectural elements. What happens if very different elements intersect? How to avoid the collapse of learning? Is data curation a political act? Can one make a selection of images that establish an alternative line to formal architecture?
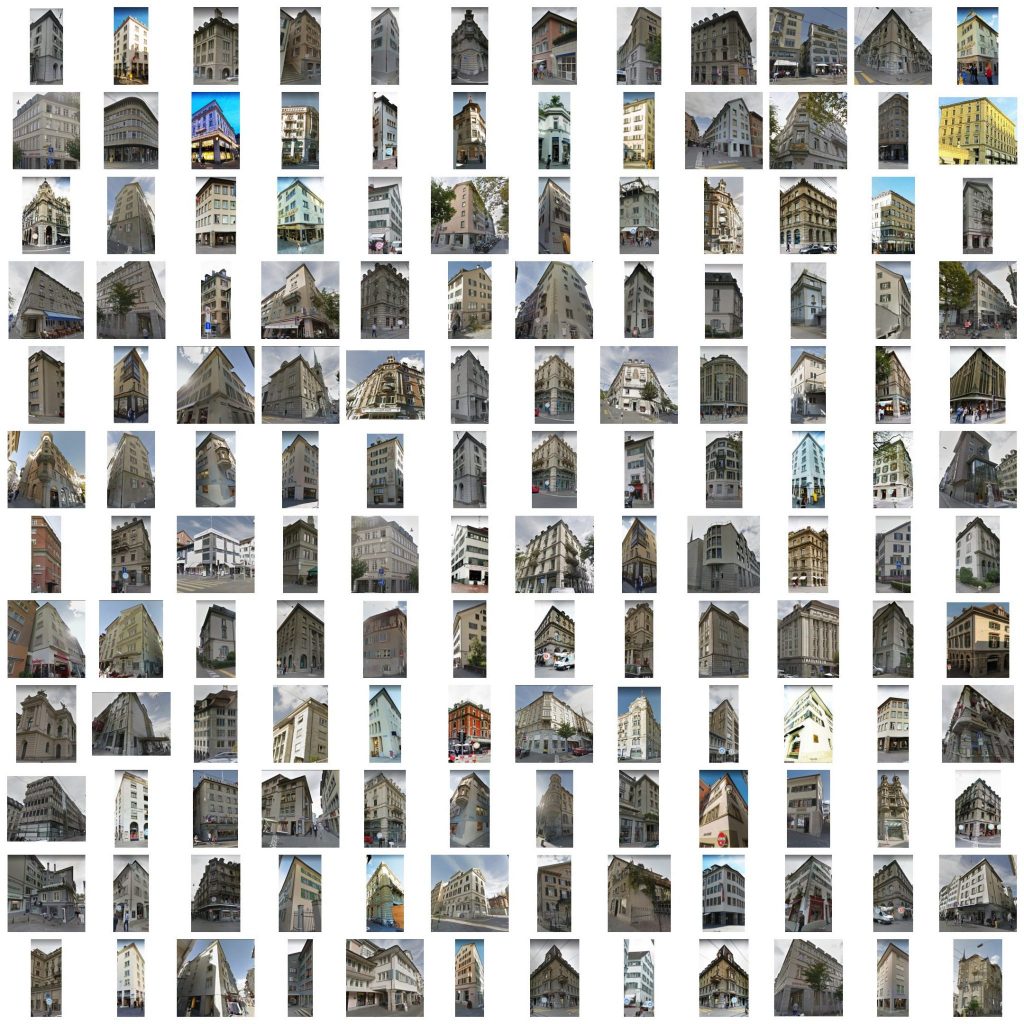
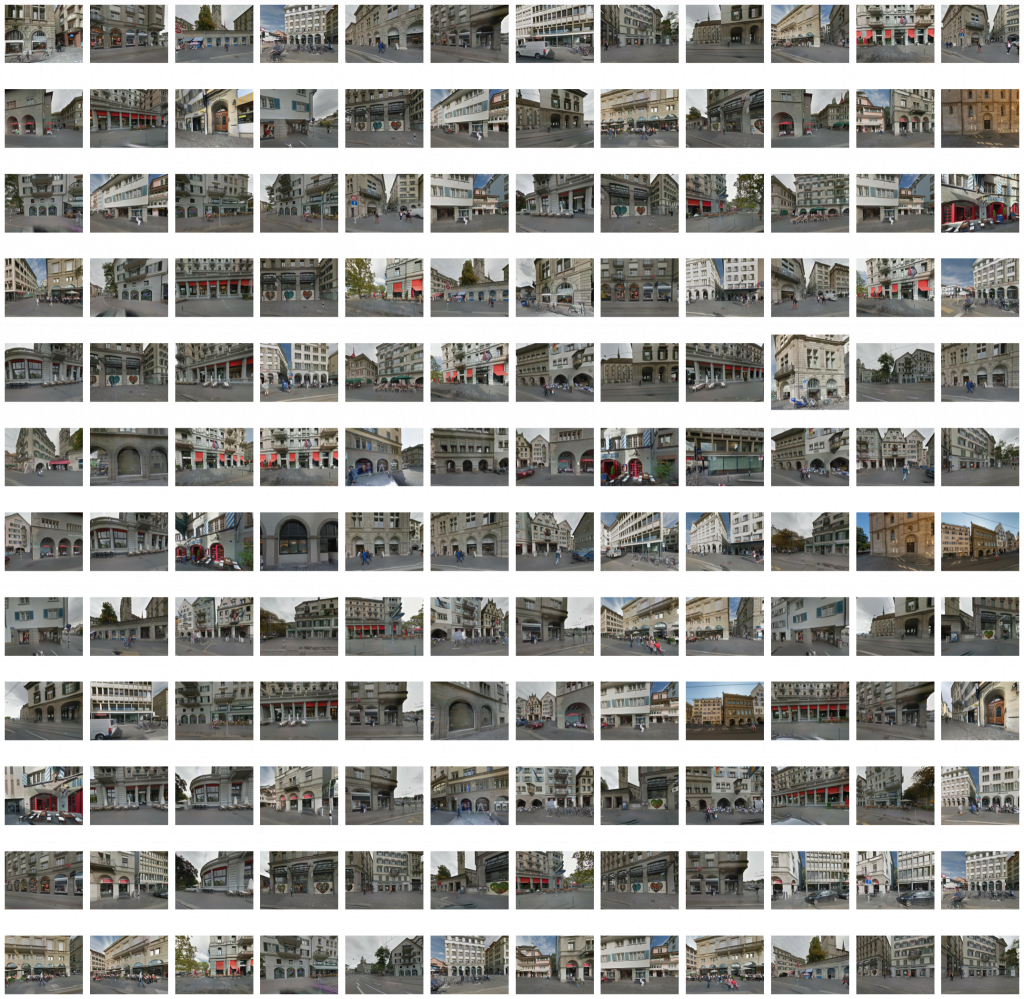
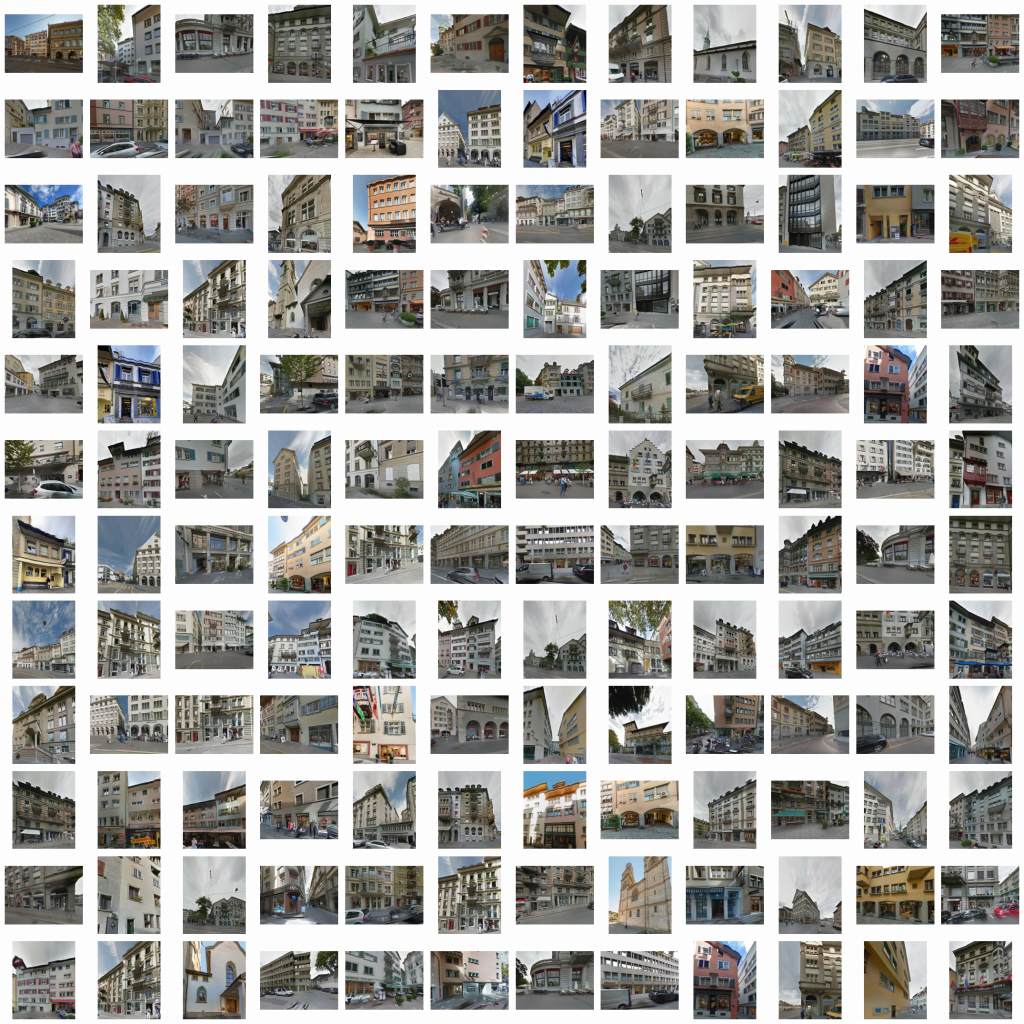
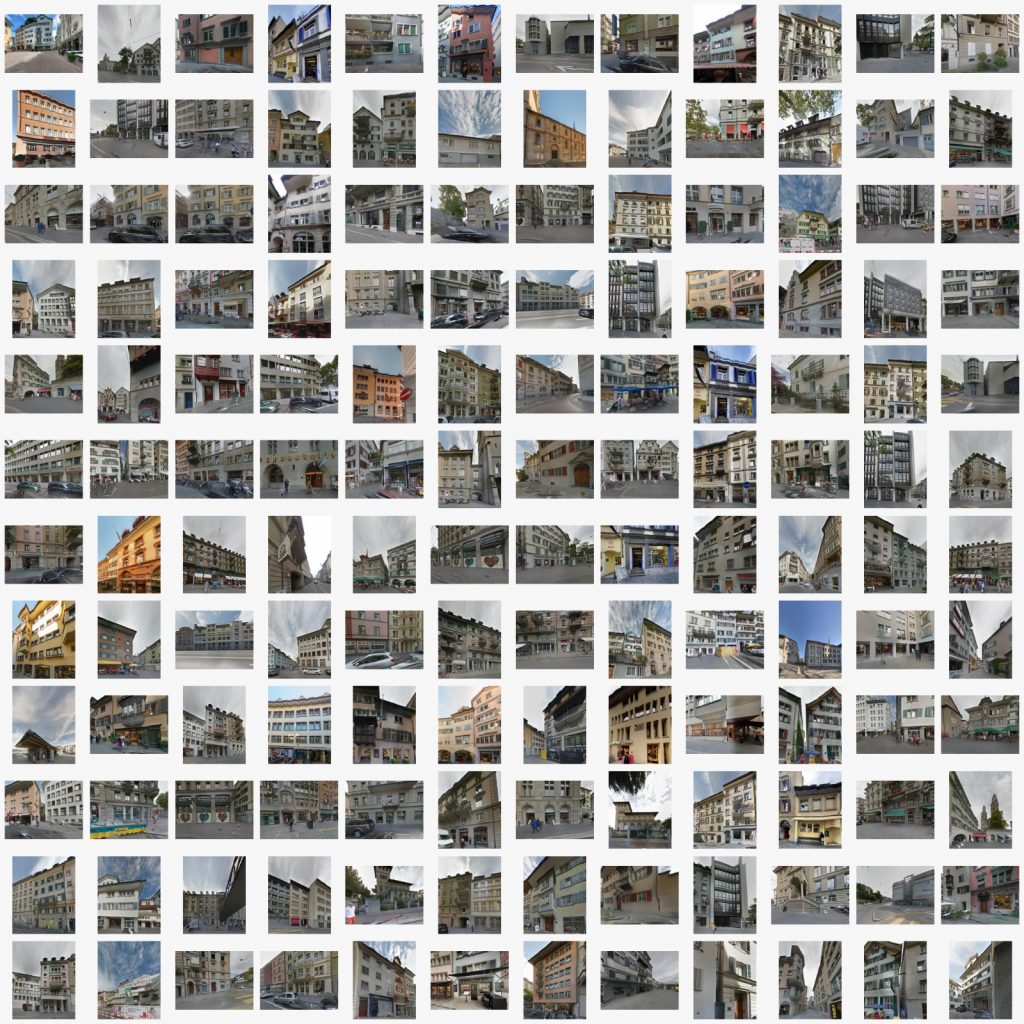
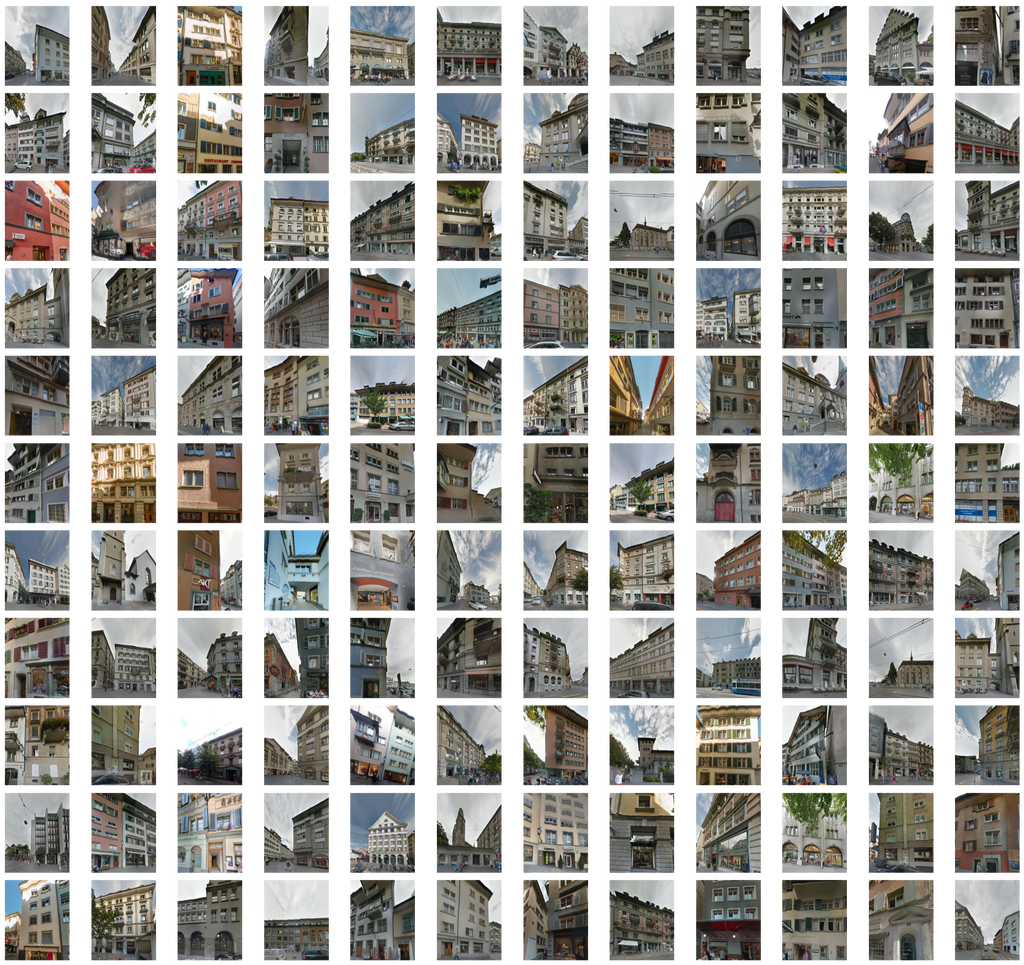
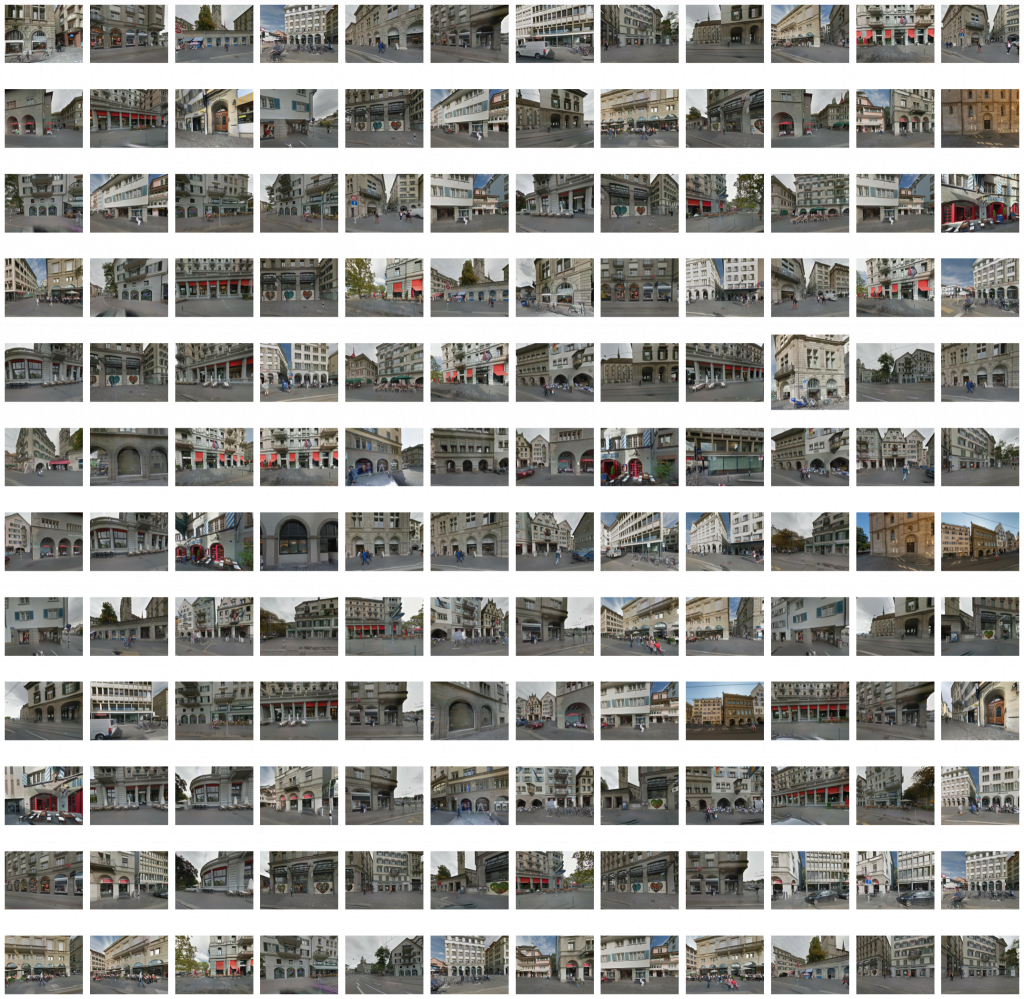