Intelligent Maintenance and Operations Systems (IMOS)
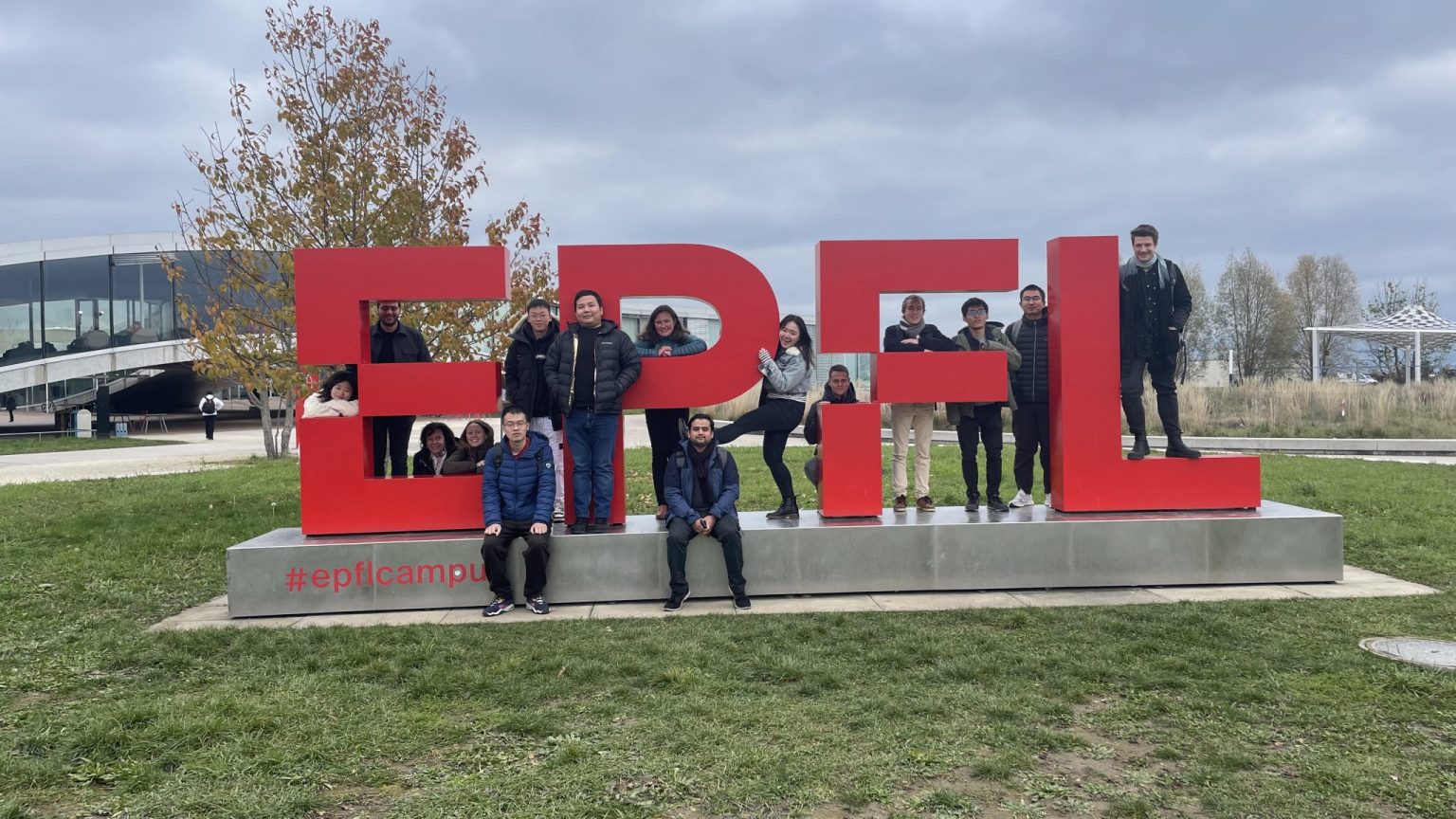
Intelligent Maintenance & Operations Systems
Our research focuses on developing intelligent algorithms to improve performance, reliability and availability of complex industrial assets and making the maintenance more cost efficient.
The amount of measured and collected condition monitoring data for complex industrial assets has been recently increasing significantly due to falling costs, improved technology, and increased reliability of sensors and data transmission.
The measured condition monitoring signals of complex industrial assets are typically high dimensional, highly redundant, have several interdependencies and prevalent non-linear relationships. The diversity of the fault types and operating conditions makes it often impossible to extract and learn the fault patterns of all the possible fault types affecting a system and to develop model-based approaches. Even collecting a representative dataset with all possible operating conditions can be a challenging task (depending on the variability of the operating regimes of the assets) and may delay the implementation of data-driven fault detection systems.
This mission involves tackling the following challenges:
- Decision support: Decision making for effective maintenance of complex systems and fleets of systems is complex and requires an integration of several sources of information with different degrees of uncertainty :
- The current health condition of each system.
- Predicted evolution of the system state condition including the uncertainty of the performed predictions.
- The scheduled maintenance plan.
- Scheduled production or operation plan.
- Anticipated future operating conditions.
- Costs of maintenance resources and unavailability.
- Restrictions of resource availability.
- The system configuration and decision alternatives.