Quality of Space
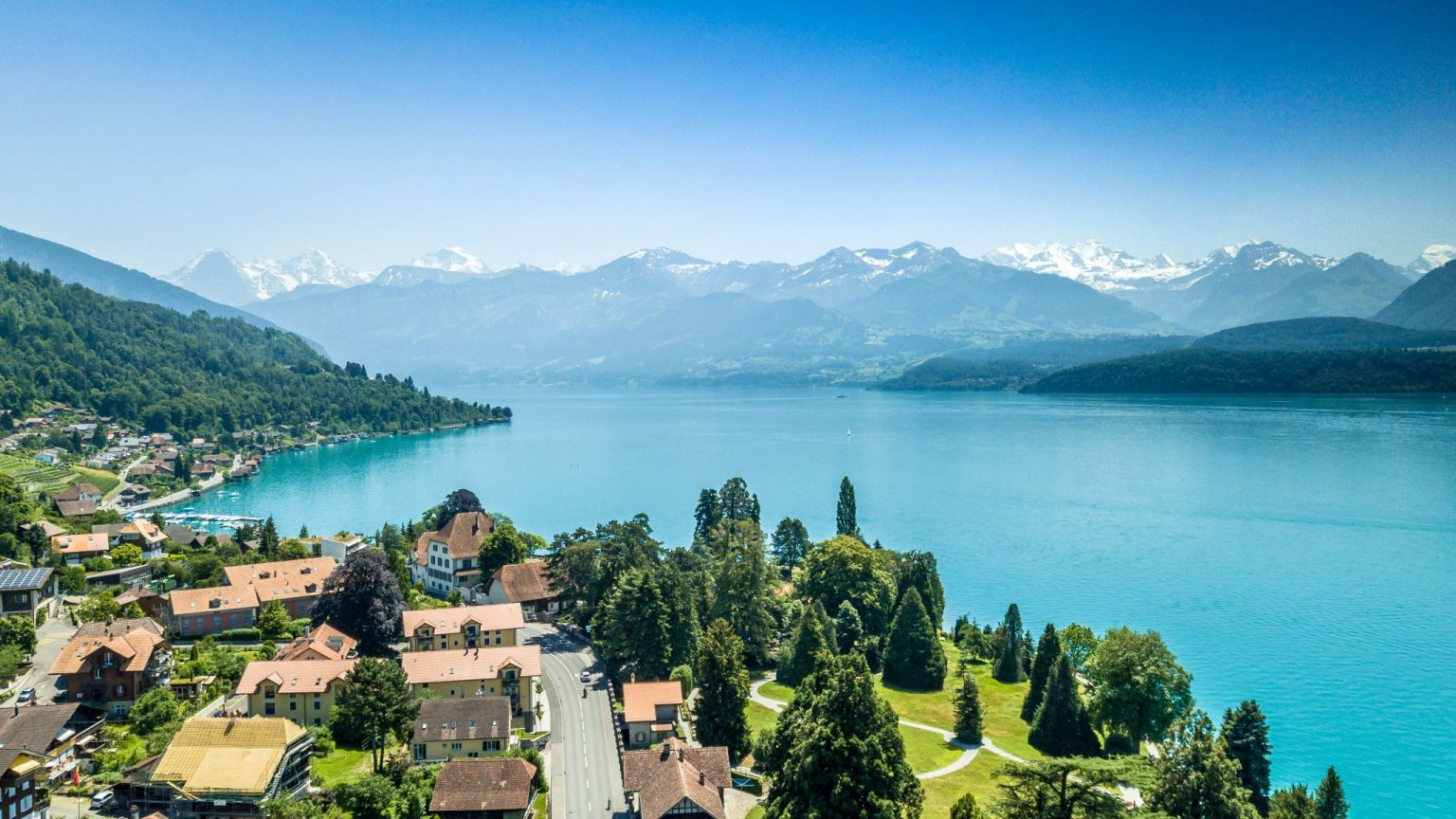
(c) Andreas M
Project team: Claudia R. Binder, Emanuele Massaro, Lorenzo Donadio – EPFL
Funding: Swiss Mobiliar
Duration: 2017 – 2022
For centuries, quality of space was a subject of studies across different disciplines. Over time it has become clear that quality of space in cities depends on several parameters such as social, economic and well-being measures. Those parameters are based on general statistical quantities. However, not all parameters are seen equally important for different parties. For example, policy makers put more emphasis on the economic ones, while individuals might have differing preferences. Thus, in some cases, economic parameters can be negatively correlated with certain quality of space facets, such as people leisure time or their need to live in healthy environments.
The goal of this project is to provide data-driven solutions that can be used to predict quality of life on a city and/or neighborhood level. Currently different quality of space parameters are often calculated on a much more coarse-grained scale than cities (e.g. for regions, provinces or whole countries), although some Swiss cities have started to include measures of quality of space, and of perceived utility of urban policies in their regular population surveys. What is needed is to be able to “zoom in” to the city or neighborhood level so that we can describe the quality of space for the exact location where people live in with indices, rather than using average values that very often have large deviations. Thus, we need to be able to depict people’s preferences for space and space characteristics.
Insurance datasets are of paramount interest since they could provide a panoramic view of human life through the lens of wellbeing, social cliques, urban safety, etc. However, unlike specific datasets that are collected using a certain methodology, this raw ad-hoc data could not bring insights directly, and urges for developing models to link individual information to comprehensible indicators that can be used in urban planning, policy making and business decisions. A key challenge lies in uncovering the hidden information buried in these unexplored datasets to address some of the urgent societal issues of our time. In this project, we aim to elucidate how bigger socio-economic patterns and urban trends could be understood utilizing Swiss Mobiliar dataset.
The goal of our research is to understand quality of space on a city level using data analytics and machine learning techniques. We will develop a methodology approach that can be used to characterize quality of space on a city or neighborhood level starting from individual insurance customer’s data and linking it with statistical information. With our model, aim to “zoom in” to the city or neighborhood level in order to provide people indices that can describe quality of space for the exact location where they live, rather than provide them with average values that very often have large deviations. Furthermore, we consider that this information can be used either by the policy makers at the city or cantonal level.