We are broadly interested in understanding biological phenomena in a quantitative way, through physical concepts and mathematical and computational tools. In particular, we investigate the relative impacts of optimization and historical contingency in biological evolution. While this question has a long history in biology, remarkable recent experimental advances allow us to investigate it more precisely through quantitative approaches. Our research spans multiple scales, from biomolecules to microbial populations, with specific interests in making sense of protein sequence data and in modeling antibiotic resistance evolution. We develop biophysical models, combining analytical work and numerical simulations. We also perform inference on biological data, using both physics-inspired methods and machine learning. We enjoy collaborating with experimentalists.
Interacting proteins play crucial parts in the cell, acting as enzymes, molecular motors, receptors, and more. The amino-acid sequence of a protein encodes its function, including its structure and interactions. In evolution, random mutations affect the sequence, while natural selection acts at the level of function. Hence, shedding light on the sequence-function mapping of proteins is central to a systems-level understanding of cells, and has far-reaching applications in synthetic biology and drug targeting. The current explosion of available sequence data enables data-driven approaches to this question.
In alignments of homologous protein sequences, which have significant similarity due to shared ancestry, correlations exist between certain amino-acid sites. These correlations can arise both from functional optimization, as homologs tend to maintain similar structures and functions, and from historical contingency. They can thus give us deep insights into protein function and evolution. We employ both traditional inference methods and protein language models. In particular, we are interested in predicting interaction partners from protein sequences, and in making sense of collective modes of correlations in protein sequences.
Selected recent publications on this topic (for a full list of publications go here):
- Protein language models trained on multiple sequence alignments learn phylogenetic relationships
U Lupo, D Sgarbossa, AF BitbolNature Communications 13 (1), 6298 (2022)
- Generative power of a protein language model trained on multiple sequence alignments
D Sgarbossa, U Lupo, AF BitboleLife 12, e79854 (2023)See highlight for more information
- Impact of phylogeny on structural contact inference from protein sequence data
N Dietler, U Lupo, AF BitbolJournal of the Royal Society Interface 20 (199), 20220707 (2023)
- Combining phylogeny and coevolution improves the inference of interaction partners among paralogous proteins
CA Gandarilla-Pérez, S Pinilla, AF Bitbol, M Weigt
PLOS Computational Biology 19 (3), e1011010 (2023)
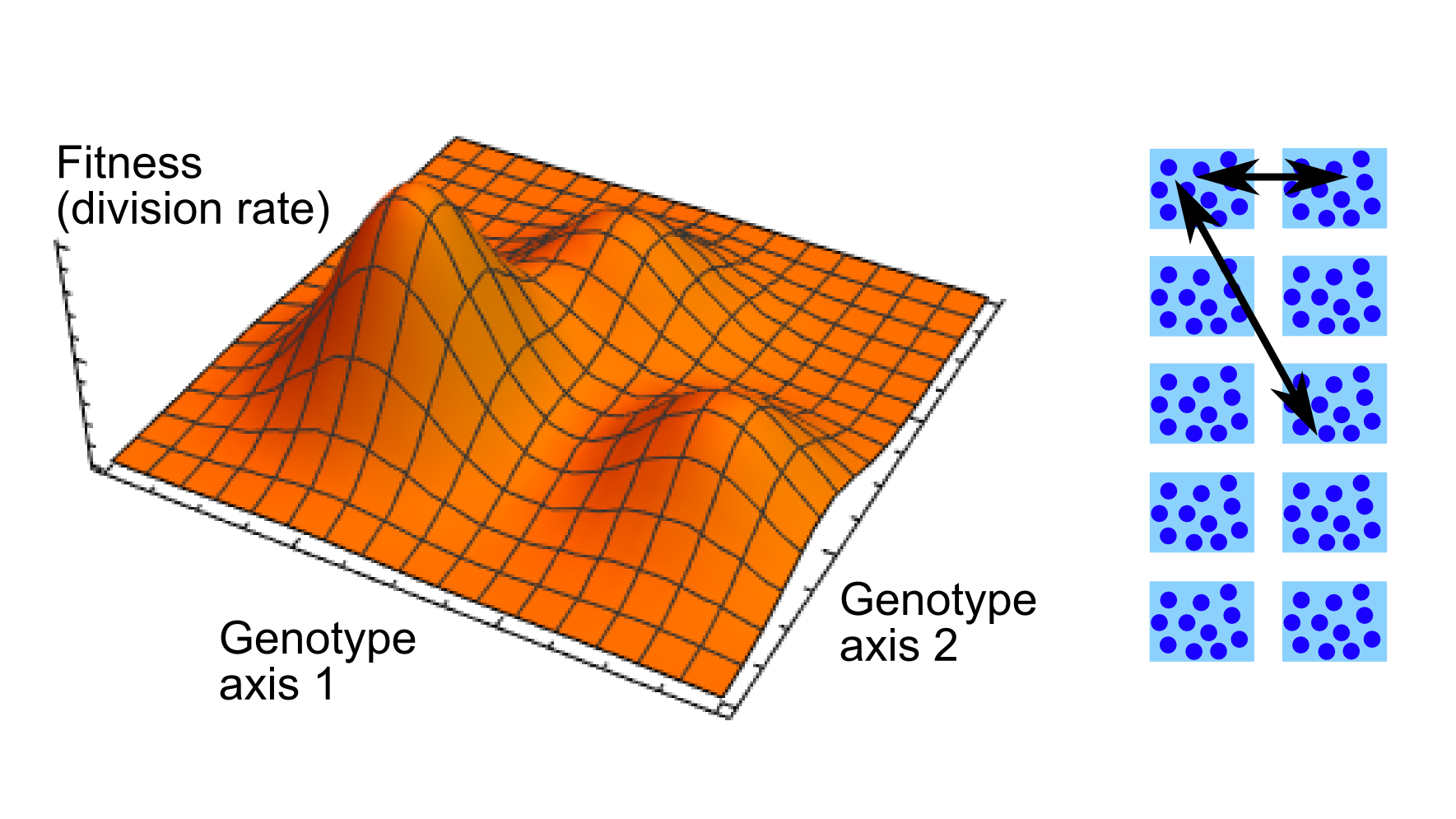
Populations of living organisms are pushed toward optimality through Darwinian evolution by random mutations and natural selection of the fittest. However, populations may not be able to reach the absolute optimum of a fitness landscape from a given starting point, due to the ruggedness of this fitness landscape, just like a physical system with a glassy energy landscape. Populations may also not have time to reach an optimum before the environment and the fitness landscape change. Therefore, evolutionary history can leave a footprint in living organisms, and “replaying life’s tape” might not yield the same outcome of evolution.
Experimental studies of microorganisms’ fitness landscapes are revealing the ubiquitousness of epistasis (interactions between genes and context-dependence of mutational effects) and of ruggedness. Furthermore, real microbial populations are very often subdivided between habitats and possess complex geographic structures. We investigate how a population’s ability to explore its fitness landscape depends on its geographic structure. Some of our projects specifically focus on the evolution of antimicrobial resistance, and on the evolution of bacteria in the gut.
Selected recent publications on this topic (for a full list of publications go here):
- Resist or perish: fate of a microbial population subjected to a periodic presence of antimicrobial
L Marrec, AF Bitbol
PLOS Computational Biology 16 (4), e1007798 (2020) - Toward a universal model for spatially structured populations
L Marrec, I Lamberti, AF Bitbol
Physical Review Letters 127 (21), 218102 (2021) - Hydrodynamic flow and concentration gradients in the gut enhance neutral bacterial diversity
D Labavić, C Loverdo, AF Bitbol
Proceedings of the National Academy of Sciences 119 (1), e2108671119 (2022)
See highlight for more information - Impact of population size on early adaptation in rugged fitness landscapes
R Servajean, AF Bitbol
Philosophical Transactions of the Royal Society B 378 (1877), 20220045 (2023)