Fostering excellence in imaging at EPFL, across scales and domains
A holistic vision of imaging
The EPFL Center for Imaging strives to accelerate discoveries in imaging and translate them to applications, favoring the reliance on shared tools and resources for the EPFL community.
By looking at imaging through a unifying lens across scales and applications, we nurture a thriving imaging network on campus and work to design common solutions to shared problems.
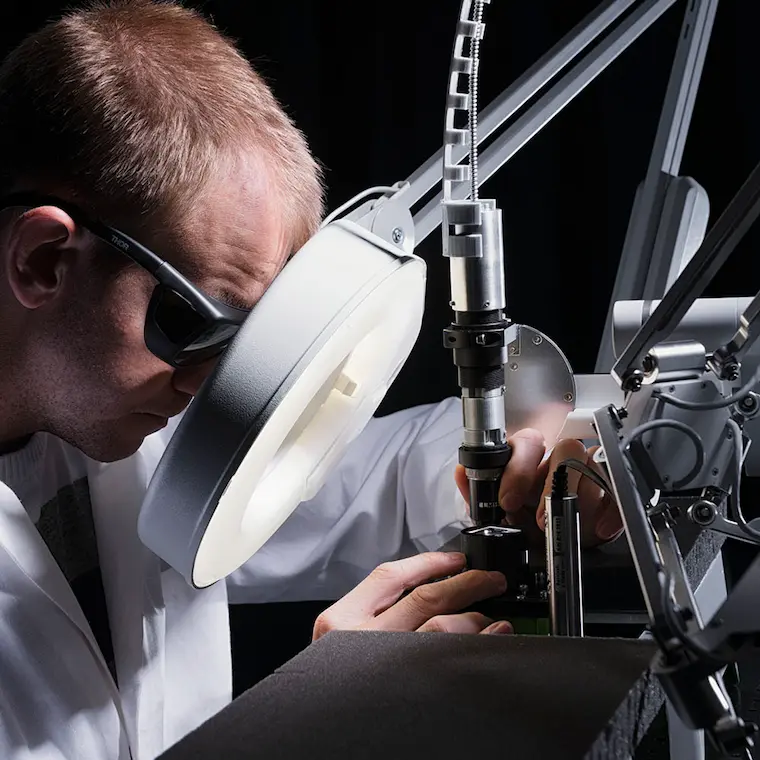
Meet the EPFL labs active in imaging
We pool the expertise of over sixty EPFL laboratories developing cutting-edge imaging technology, from acquisition instruments to computer vision and image processing. Browse and discover the actors of EPFL's thriving imaging community!
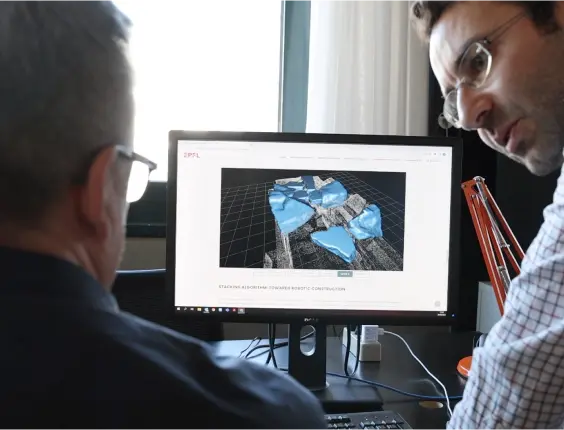
Get support from our imaging experts!
Need help with your project or with finding the right tools? Our team is here to provide advice and technical support to all EPFL scientists for all image-analysis related questions. Get proper support!
Network and learn at our imaging events
Connect with your peers and advance your imaging skills: Save the dates to our year-round events!
Acquire top-level skills in imaging
See how the EPFL Center for Imaging fosters top-level training in imaging for EPFL students and research staff.
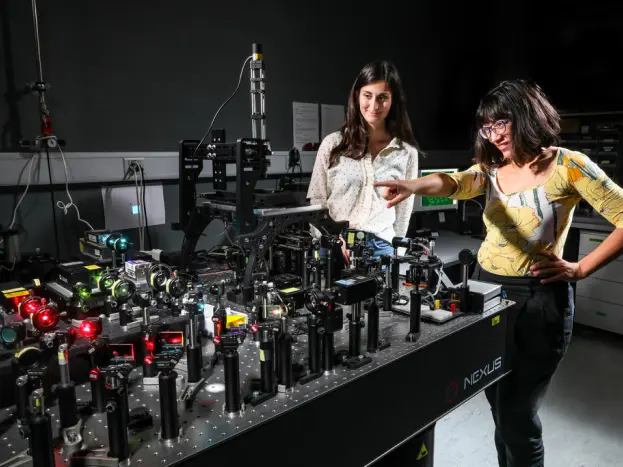
Catch our latest news in imaging
Browse our news to uncover the latest breakthroughs and discoveries in imaging at EPFL.